-
Notifications
You must be signed in to change notification settings - Fork 6.8k
Example on Deconvolution Layer's Configuration #1514
Comments
deconvolution with 2x upsampling can be done like this:
|
Thanks @piiswrong , I appreciate your helps a lot. Sincerely, |
I think you should define your label as 0 and 1, where 0 is membrane and 1 is not. It is not a good option to use 255 unless you want this label to be ignored. @tmquan |
@ascust Then I set the deconvolutional output as a LogisticRegressionOutput as following: In contrast, the example on fcn-xs folder said that I should use the SoftmaxOutput layer to make a prediction on entire segmentation. It confused me and I am struggling with that issue quite a bit. Best regards, |
LogisticRegressionOutput is for binary classification, it is supposed to work. Since I never use this layer before, I can not say much about it. Anyway, SoftmaxOutput is for Multiclass classification and binary classification is only a special case, I am sure this will work. Just like your settings, if 30 is your batch size, there is a (2, 512, 512) score map for each image in the batch. For prediction task, you can simply choose use argmax along the first axis. |
Dear mxnet community,
The current documentation on Deconvolution layer is somehow difficult to catch up.
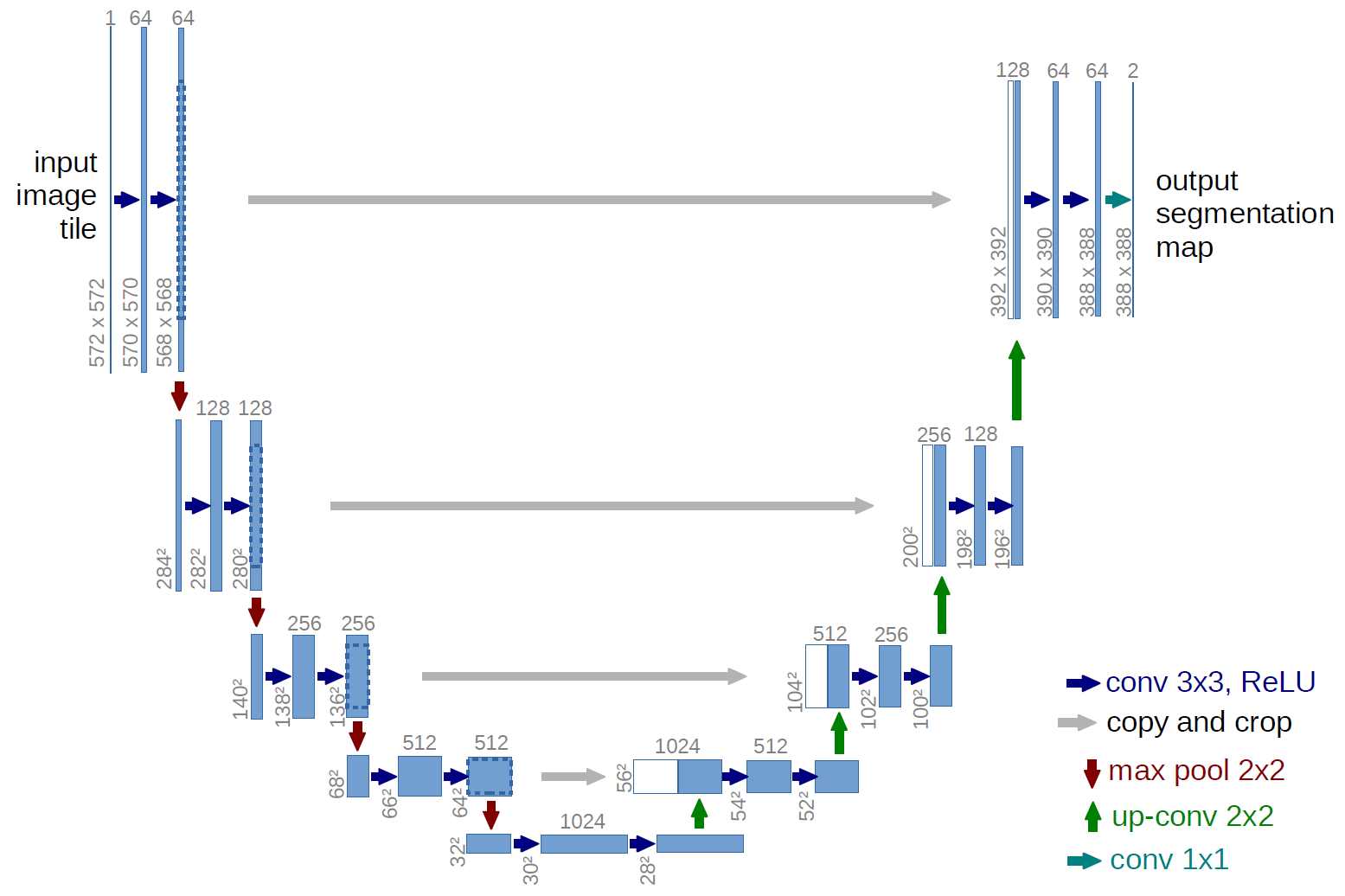
In particular, I want to reproduce u-net (for image segmentation purpose) available at http://lmb.informatik.uni-freiburg.de/people/ronneber/u-net/
I stopped by the example https://github.com/dmlc/mxnet/blob/master/example/fcn-xs/symbol_fcnxs.py
and its utilization is still fuzzy, too.
Could you give me some direction (or example) how to use Deconvolution layer for such image segmentation task as follows:
I have a collection of n training volume images (t+xy) and their associative segmentation
(n, 64, 128, 128) ~> (n, 64, 128, 128)
where n is number of training instances, 64 is the temporal dimension, 128 is the spatial dimension.
How to construct a simple fully convolutional network using mxnet on this problem?
data ~> convolutional layer ~> pooling (downsample by 2) ~> deconvolutional layer ~> Upsampling by 2 ~> segmentation?
Thanks a lot
The text was updated successfully, but these errors were encountered: