diff --git a/.github/workflows/docs.yml b/.github/workflows/docs.yml
index 10b7cf9a432..7b3ab8f9925 100644
--- a/.github/workflows/docs.yml
+++ b/.github/workflows/docs.yml
@@ -18,6 +18,8 @@ jobs:
uses: actions/setup-python@v1
with:
python-version: '3.x'
+ - name: Install CN fonts
+ run: sudo apt-get update && sudo apt-get install fonts-arphic-uming
- name: install Python Dependencies
run: pip3 install nbconvert==5.6.1 mkdocs mkdocs-exclude mknotebooks==0.4.1 mkdocs-material jupyter Pygments Markdown==3.2.2
- name: Install IJava kernel
diff --git a/api/src/main/java/ai/djl/Application.java b/api/src/main/java/ai/djl/Application.java
index 76d8e8926ac..299c7e1ccc0 100644
--- a/api/src/main/java/ai/djl/Application.java
+++ b/api/src/main/java/ai/djl/Application.java
@@ -268,15 +268,6 @@ public interface Tabular {
* @see The D2L
* chapter introducing this application
*/
- Application SOFTMAX_REGRESSION = new Application("tabular/linear_regression");
-
- /**
- * This is erroneous because random forest is a technique (not deep learning), not an
- * application.
- *
- *
The actual application is likely to be in {@link Tabular}, especially {@link
- * #SOFTMAX_REGRESSION}.
- */
- Application RANDOM_FOREST = new Application("tabular/random_forest");
+ Application SOFTMAX_REGRESSION = new Application("tabular/softmax_regression");
}
}
diff --git a/api/src/main/java/ai/djl/BaseModel.java b/api/src/main/java/ai/djl/BaseModel.java
index 31e81f0efd2..d6fa016fc18 100644
--- a/api/src/main/java/ai/djl/BaseModel.java
+++ b/api/src/main/java/ai/djl/BaseModel.java
@@ -267,6 +267,22 @@ public Path getModelPath() {
return modelDir;
}
+ /** {@inheritDoc} */
+ @Override
+ public String toString() {
+ StringBuilder sb = new StringBuilder(200);
+ sb.append("Model (\n\tName: ").append(modelName);
+ if (modelDir != null) {
+ sb.append("\n\tModel location: ").append(modelDir.toAbsolutePath());
+ }
+ sb.append("\n\tData Type: ").append(dataType);
+ for (Map.Entry entry : properties.entrySet()) {
+ sb.append("\n\t").append(entry.getKey()).append(": ").append(entry.getValue());
+ }
+ sb.append("\n)");
+ return sb.toString();
+ }
+
/** {@inheritDoc} */
@SuppressWarnings("deprecation")
@Override
diff --git a/api/src/main/java/ai/djl/engine/Engine.java b/api/src/main/java/ai/djl/engine/Engine.java
index 7673673a116..336aad53648 100644
--- a/api/src/main/java/ai/djl/engine/Engine.java
+++ b/api/src/main/java/ai/djl/engine/Engine.java
@@ -15,6 +15,7 @@
import ai.djl.Device;
import ai.djl.Model;
import ai.djl.ndarray.NDManager;
+import ai.djl.nn.SymbolBlock;
import ai.djl.training.GradientCollector;
import ai.djl.training.LocalParameterServer;
import ai.djl.training.ParameterServer;
@@ -190,6 +191,14 @@ public Device defaultDevice() {
return defaultDevice;
}
+ /**
+ * Construct an empty SymbolBlock for loading.
+ *
+ * @param manager the manager to manage parameters
+ * @return Empty {@link SymbolBlock} for static graph
+ */
+ public abstract SymbolBlock newSymbolBlock(NDManager manager);
+
/**
* Constructs a new model.
*
diff --git a/api/src/main/java/ai/djl/modality/nlp/Decoder.java b/api/src/main/java/ai/djl/modality/nlp/Decoder.java
index 72a6ead095f..e6dd38fedf3 100644
--- a/api/src/main/java/ai/djl/modality/nlp/Decoder.java
+++ b/api/src/main/java/ai/djl/modality/nlp/Decoder.java
@@ -64,8 +64,8 @@ public void initializeChildBlocks(NDManager manager, DataType dataType, Shape...
/** {@inheritDoc} */
@Override
- public Shape[] getOutputShapes(NDManager manager, Shape[] inputShapes) {
- return block.getOutputShapes(manager, inputShapes);
+ public Shape[] getOutputShapes(Shape[] inputShapes) {
+ return block.getOutputShapes(inputShapes);
}
/** {@inheritDoc} */
diff --git a/api/src/main/java/ai/djl/modality/nlp/Encoder.java b/api/src/main/java/ai/djl/modality/nlp/Encoder.java
index 7ad4fbdcf6f..e3b1631322f 100644
--- a/api/src/main/java/ai/djl/modality/nlp/Encoder.java
+++ b/api/src/main/java/ai/djl/modality/nlp/Encoder.java
@@ -79,8 +79,8 @@ public void initializeChildBlocks(NDManager manager, DataType dataType, Shape...
/** {@inheritDoc} */
@Override
- public Shape[] getOutputShapes(NDManager manager, Shape[] inputShapes) {
- return block.getOutputShapes(manager, inputShapes);
+ public Shape[] getOutputShapes(Shape[] inputShapes) {
+ return block.getOutputShapes(inputShapes);
}
/** {@inheritDoc} */
diff --git a/api/src/main/java/ai/djl/modality/nlp/EncoderDecoder.java b/api/src/main/java/ai/djl/modality/nlp/EncoderDecoder.java
index afc0958ace9..a7c47380a9d 100644
--- a/api/src/main/java/ai/djl/modality/nlp/EncoderDecoder.java
+++ b/api/src/main/java/ai/djl/modality/nlp/EncoderDecoder.java
@@ -97,19 +97,18 @@ public NDList forward(
* @param manager the NDManager to initialize the parameters
* @param dataType the datatype of the parameters
* @param inputShapes the shapes of the inputs to the block
- * @return the shapes of the outputs of the block
*/
@Override
- public Shape[] initialize(NDManager manager, DataType dataType, Shape... inputShapes) {
+ public void initialize(NDManager manager, DataType dataType, Shape... inputShapes) {
beforeInitialize(inputShapes);
encoder.initialize(manager, dataType, inputShapes[0]);
- return decoder.initialize(manager, dataType, inputShapes[1]);
+ decoder.initialize(manager, dataType, inputShapes[1]);
}
/** {@inheritDoc} */
@Override
- public Shape[] getOutputShapes(NDManager manager, Shape[] inputShapes) {
- return decoder.getOutputShapes(manager, new Shape[] {inputShapes[1]});
+ public Shape[] getOutputShapes(Shape[] inputShapes) {
+ return decoder.getOutputShapes(new Shape[] {inputShapes[1]});
}
/** {@inheritDoc} */
diff --git a/api/src/main/java/ai/djl/modality/nlp/embedding/TrainableTextEmbedding.java b/api/src/main/java/ai/djl/modality/nlp/embedding/TrainableTextEmbedding.java
index f848f25f3e5..4fd7dc71277 100644
--- a/api/src/main/java/ai/djl/modality/nlp/embedding/TrainableTextEmbedding.java
+++ b/api/src/main/java/ai/djl/modality/nlp/embedding/TrainableTextEmbedding.java
@@ -88,7 +88,7 @@ public void initializeChildBlocks(NDManager manager, DataType dataType, Shape...
/** {@inheritDoc} */
@Override
- public Shape[] getOutputShapes(NDManager manager, Shape[] inputShapes) {
- return trainableWordEmbedding.getOutputShapes(manager, inputShapes);
+ public Shape[] getOutputShapes(Shape[] inputShapes) {
+ return trainableWordEmbedding.getOutputShapes(inputShapes);
}
}
diff --git a/api/src/main/java/ai/djl/ndarray/BaseNDManager.java b/api/src/main/java/ai/djl/ndarray/BaseNDManager.java
index 1b80170d908..2459b37f333 100644
--- a/api/src/main/java/ai/djl/ndarray/BaseNDManager.java
+++ b/api/src/main/java/ai/djl/ndarray/BaseNDManager.java
@@ -15,6 +15,7 @@
import ai.djl.Device;
import ai.djl.ndarray.types.DataType;
import ai.djl.ndarray.types.Shape;
+import ai.djl.util.Pair;
import ai.djl.util.PairList;
import java.nio.Buffer;
import java.nio.file.Path;
@@ -34,12 +35,14 @@ public abstract class BaseNDManager implements NDManager {
protected String name;
protected Device device;
protected ConcurrentHashMap resources;
+ protected ConcurrentHashMap> tempResources;
protected AtomicBoolean closed = new AtomicBoolean(false);
protected BaseNDManager(NDManager parent, Device device) {
this.parent = parent;
this.device = Device.defaultIfNull(device, getEngine());
resources = new ConcurrentHashMap<>();
+ tempResources = new ConcurrentHashMap<>();
uid = UUID.randomUUID().toString();
}
@@ -49,6 +52,12 @@ public NDArray create(String data) {
throw new UnsupportedOperationException("Not supported!");
}
+ /** {@inheritDoc} */
+ @Override
+ public NDArray create(String[] data) {
+ throw new UnsupportedOperationException("Not supported!");
+ }
+
/** {@inheritDoc} */
@Override
public NDArray create(Shape shape, DataType dataType) {
@@ -197,7 +206,7 @@ public String toString() {
/** {@inheritDoc} */
@Override
- public synchronized void attach(String resourceId, AutoCloseable resource) {
+ public synchronized void attachInternal(String resourceId, AutoCloseable resource) {
if (closed.get()) {
throw new IllegalStateException("NDManager has been closed already.");
}
@@ -206,7 +215,17 @@ public synchronized void attach(String resourceId, AutoCloseable resource) {
/** {@inheritDoc} */
@Override
- public synchronized void detach(String resourceId) {
+ public void tempAttachInternal(
+ NDManager originalManager, String resourceId, NDResource resource) {
+ if (closed.get()) {
+ throw new IllegalStateException("NDManager has been closed already.");
+ }
+ tempResources.put(resourceId, new Pair<>(resource, originalManager));
+ }
+
+ /** {@inheritDoc} */
+ @Override
+ public synchronized void detachInternal(String resourceId) {
if (closed.get()) {
// This may happen in the middle of BaseNDManager.close()
return;
@@ -238,7 +257,14 @@ public synchronized void close() {
logger.error("Resource close failed.", e);
}
}
- parent.detach(uid);
+ for (Pair resource : tempResources.values()) {
+ try {
+ resource.getKey().attach(resource.getValue());
+ } catch (Exception e) {
+ logger.error("Temporary resource return failed.", e);
+ }
+ }
+ parent.detachInternal(uid);
resources.clear();
}
}
diff --git a/api/src/main/java/ai/djl/ndarray/NDArray.java b/api/src/main/java/ai/djl/ndarray/NDArray.java
index 758c052537f..559439e68b3 100644
--- a/api/src/main/java/ai/djl/ndarray/NDArray.java
+++ b/api/src/main/java/ai/djl/ndarray/NDArray.java
@@ -40,7 +40,7 @@
* href="https://github.com/awslabs/djl/blob/master/docs/development/memory_management.md">NDArray
* Memory Management Guide
*/
-public interface NDArray extends AutoCloseable {
+public interface NDArray extends NDResource {
/**
* Decodes {@code NDArray} from bytes.
@@ -53,13 +53,6 @@ static NDArray decode(NDManager manager, byte[] byteArray) {
return manager.decode(byteArray);
}
- /**
- * Returns the {@link NDManager} used to create this {@code NDArray}.
- *
- * @return the {@link NDManager} used to create this {@code NDArray}
- */
- NDManager getManager();
-
/**
* Returns the name of this {@code NDArray}.
*
@@ -146,27 +139,6 @@ default byte[] encode() {
return NDSerializer.encode(this);
}
- /**
- * Attaches this {@code NDArray} to the specified {@link NDManager}.
- *
- *
Attached resource will be closed when the {@link NDManager} is closed.
- *
- * @param manager the {@link NDManager} to be attached
- * @return the original {@link NDManager}
- */
- NDManager attach(NDManager manager);
-
- /**
- * Detaches the {@code NDArray} from current {@link NDManager}'s lifecycle.
- *
- *
The {@code NDArray} becomes un-managed, it is the user's responsibility to close the
- * {@code NDArray}. Failure to close the resource might cause your machine to run out of native
- * memory.
- *
- * @see NDManager
- */
- void detach();
-
/**
* Moves this {@code NDArray} to a different {@link Device}.
*
@@ -371,6 +343,15 @@ default boolean[] toBooleanArray() {
return ret;
}
+ /**
+ * Converts this {@code NDArray} to a String array.
+ *
+ *
This method is only applicable to the String typed NDArray and not for printing purpose
+ *
+ * @return Array of Strings
+ */
+ String[] toStringArray();
+
/**
* Converts this {@code NDArray} to a Number array based on its {@link DataType}.
*
diff --git a/api/src/main/java/ai/djl/ndarray/NDArrayAdapter.java b/api/src/main/java/ai/djl/ndarray/NDArrayAdapter.java
index 8afb9ca3ec0..613338ad025 100644
--- a/api/src/main/java/ai/djl/ndarray/NDArrayAdapter.java
+++ b/api/src/main/java/ai/djl/ndarray/NDArrayAdapter.java
@@ -83,7 +83,7 @@ default SparseFormat getSparseFormat() {
/** {@inheritDoc} */
@Override
- default NDManager attach(NDManager manager) {
+ default void attach(NDManager manager) {
throw new UnsupportedOperationException(UNSUPPORTED_MSG);
}
@@ -135,6 +135,12 @@ default NDArray stopGradient() {
throw new UnsupportedOperationException(UNSUPPORTED_MSG);
}
+ /** {@inheritDoc} */
+ @Override
+ default String[] toStringArray() {
+ throw new UnsupportedOperationException(UNSUPPORTED_MSG);
+ }
+
/** {@inheritDoc} */
@Override
default ByteBuffer toByteBuffer() {
diff --git a/api/src/main/java/ai/djl/ndarray/NDList.java b/api/src/main/java/ai/djl/ndarray/NDList.java
index 88f727cf807..021de74e810 100644
--- a/api/src/main/java/ai/djl/ndarray/NDList.java
+++ b/api/src/main/java/ai/djl/ndarray/NDList.java
@@ -19,12 +19,10 @@
import java.io.DataInputStream;
import java.io.DataOutputStream;
import java.io.IOException;
+import java.io.InputStream;
import java.util.ArrayList;
import java.util.Arrays;
import java.util.Collection;
-import java.util.List;
-import java.util.stream.Collectors;
-import java.util.stream.IntStream;
/**
* An {@code NDList} represents a sequence of {@link NDArray}s with names.
@@ -34,7 +32,7 @@
*
* @see NDArray
*/
-public class NDList extends ArrayList implements AutoCloseable {
+public class NDList extends ArrayList implements NDResource {
private static final long serialVersionUID = 1L;
@@ -77,7 +75,18 @@ public NDList(Collection other) {
* @return {@code NDList}
*/
public static NDList decode(NDManager manager, byte[] byteArray) {
- try (DataInputStream dis = new DataInputStream(new ByteArrayInputStream(byteArray))) {
+ return decode(manager, new ByteArrayInputStream(byteArray));
+ }
+
+ /**
+ * Decodes NDList from {@link InputStream}.
+ *
+ * @param manager manager assigned to {@link NDArray}
+ * @param is input stream contains the ndlist information
+ * @return {@code NDList}
+ */
+ public static NDList decode(NDManager manager, InputStream is) {
+ try (DataInputStream dis = new DataInputStream(is)) {
int size = dis.readInt();
if (size < 0) {
throw new IllegalArgumentException("Invalid NDList size: " + size);
@@ -200,36 +209,28 @@ public NDList toDevice(Device device, boolean copy) {
return newNDList;
}
- /**
- * Attaches each ndarray in this list to the specified manager.
- *
- * @param manager the manager to attach the lists to
- * @return a list of {@code NDManager} with which original NDArray are attached
- * @see NDArray#attach(NDManager)
- */
- public List attach(NDManager manager) {
- return stream().map(array -> array.attach(manager)).collect(Collectors.toList());
+ /** {@inheritDoc} */
+ @Override
+ public NDManager getManager() {
+ return head().getManager();
}
- /**
- * Attaches each ndarray in this list to the specified manager.
- *
- * @param managers the list of managers to attach
- * @return a list of {@code NDManager} with which original NDArray are attached
- */
- public List attach(List managers) {
- return IntStream.range(0, size())
- .mapToObj(i -> get(i).attach(managers.get(i)))
- .collect(Collectors.toList());
+ /** {@inheritDoc} */
+ @Override
+ public void attach(NDManager manager) {
+ stream().forEach(array -> array.attach(manager));
}
- /**
- * Detaches each ndarray in this list from their current managers.
- *
- * @see NDArray#detach()
- */
+ /** {@inheritDoc} */
+ @Override
+ public void tempAttach(NDManager manager) {
+ stream().forEach(array -> array.tempAttach(manager));
+ }
+
+ /** {@inheritDoc} */
+ @Override
public void detach() {
- forEach(NDArray::detach);
+ stream().forEach(NDResource::detach);
}
/**
diff --git a/api/src/main/java/ai/djl/ndarray/NDManager.java b/api/src/main/java/ai/djl/ndarray/NDManager.java
index cfcf1609b1c..b7beb80c2b2 100644
--- a/api/src/main/java/ai/djl/ndarray/NDManager.java
+++ b/api/src/main/java/ai/djl/ndarray/NDManager.java
@@ -133,6 +133,16 @@ static NDManager newBaseManager(Device device, String engineName) {
return Engine.getEngine(engineName).newBaseManager(device);
}
+ /**
+ * Creates a new manager based on the given resource.
+ *
+ * @param resource the resource to use
+ * @return a new memory scrope containing the array
+ */
+ static NDManager from(NDResource resource) {
+ return resource.getManager().newSubManager();
+ }
+
/**
* Allocates a new engine specific direct byte buffer.
*
@@ -235,14 +245,21 @@ default NDArray create(boolean data) {
}
/**
- * Creates and initializes a scalar {@link NDArray}. NDArray of String DataType only supports
- * scalar.
+ * Creates and initializes a scalar {@link NDArray}.
*
* @param data the String data that needs to be set
* @return a new instance of {@link NDArray}
*/
NDArray create(String data);
+ /**
+ * Creates and initializes 1D {@link NDArray}.
+ *
+ * @param data the String data that needs to be set
+ * @return a new instance of {@link NDArray}
+ */
+ NDArray create(String[] data);
+
/**
* Creates and initializes a 1D {@link NDArray}.
*
@@ -1274,14 +1291,34 @@ default NDArray randomNormal(
Device getDevice();
/**
- * Attaches a {@link NDArray} or {@code NDManager} to this {@code NDManager}.
+ * Attaches a resource to this {@code NDManager}.
+ *
+ *
The attached resource will be closed when this {@code NDManager} is closed.
*
- *
Attached resource will be closed when this {@code NDManager} is closed.
+ *
This attachment is internal. Many resources will internally track which manager they are
+ * attached to. In that case, you should call {@link NDResource#attach(NDManager)} instead and
+ * that should then call attachInternal.
*
* @param resourceId the unique resourceId
* @param resource the {@link AutoCloseable} resource to be attached
*/
- void attach(String resourceId, AutoCloseable resource);
+ void attachInternal(String resourceId, AutoCloseable resource);
+
+ /**
+ * Temporarily attaches a resource to this {@code NDManager} to be returned when this is closed.
+ *
+ *
The attached resource will be returned to it's original manager when this {@code
+ * NDManager} is closed.
+ *
+ *
This attachment is internal. Many resources will internally track which manager they are
+ * attached to. In that case, you should call {@link NDResource#attach(NDManager)} instead and
+ * that should then call tempAttachInternal.
+ *
+ * @param originalManager the original manager to return the resource to
+ * @param resourceId the unique resourceId
+ * @param resource the {@link AutoCloseable} resource to be attached
+ */
+ void tempAttachInternal(NDManager originalManager, String resourceId, NDResource resource);
/**
* Detaches a {@link NDArray} from this {@code NDManager}'s lifecycle.
@@ -1290,9 +1327,49 @@ default NDArray randomNormal(
* resource. Failed to close the resource has to wait on GC to be freed, and might cause out of
* native memory.
*
+ *
This detach is internal. Many resources will internally track which manager they are
+ * attached to. In that case, you should call {@link NDResource#detach()} instead and that
+ * should then call detachInternal.
+ *
* @param resourceId the resourceId to be removed from this {@code NDManager}'s lifecycle
*/
- void detach(String resourceId);
+ void detachInternal(String resourceId);
+
+ /**
+ * Returns a value outside of this manager by attaching to this manager's parent.
+ *
+ * @param resource the resource to return
+ * @param the type of the resource
+ * @return the passed in resource, after attaching to a new manager
+ */
+ default T ret(T resource) {
+ resource.attach(getParentManager());
+ return resource;
+ }
+
+ /**
+ * Attaches all resources to this manager.
+ *
+ * @param resources the resources to attach
+ * @see NDResource#attach(NDManager)
+ */
+ default void attachAll(NDResource... resources) {
+ for (NDResource resource : resources) {
+ resource.attach(this);
+ }
+ }
+
+ /**
+ * Temporarily attaches all resources to this manager.
+ *
+ * @param resources the resources to attach
+ * @see NDResource#tempAttach(NDManager)
+ */
+ default void tempAttachAll(NDResource... resources) {
+ for (NDResource resource : resources) {
+ resource.tempAttach(this);
+ }
+ }
/**
* An engine specific generic invocation to native operation.
diff --git a/api/src/main/java/ai/djl/ndarray/NDResource.java b/api/src/main/java/ai/djl/ndarray/NDResource.java
new file mode 100644
index 00000000000..8033d022608
--- /dev/null
+++ b/api/src/main/java/ai/djl/ndarray/NDResource.java
@@ -0,0 +1,57 @@
+/*
+ * Copyright 2021 Amazon.com, Inc. or its affiliates. All Rights Reserved.
+ *
+ * Licensed under the Apache License, Version 2.0 (the "License"). You may not use this file except in compliance
+ * with the License. A copy of the License is located at
+ *
+ * http://aws.amazon.com/apache2.0/
+ *
+ * or in the "license" file accompanying this file. This file is distributed on an "AS IS" BASIS, WITHOUT WARRANTIES
+ * OR CONDITIONS OF ANY KIND, either express or implied. See the License for the specific language governing permissions
+ * and limitations under the License.
+ */
+package ai.djl.ndarray;
+
+/** An object which is managed by an {@link NDManager} and tracks the manager it is attached to. */
+public interface NDResource extends AutoCloseable {
+
+ /**
+ * Returns the {@link NDManager} that manages this.
+ *
+ * @return the {@link NDManager} that manages this.
+ */
+ NDManager getManager();
+
+ /**
+ * Attaches this {@link NDResource} to the specified {@link NDManager}.
+ *
+ *
Attached resource will be closed when the {@link NDManager} is closed.
+ *
+ * @param manager the {@link NDManager} to be attached to
+ */
+ void attach(NDManager manager);
+
+ /**
+ * Temporarily attaches this {@link NDResource} to the specified {@link NDManager}.
+ *
+ *
Attached resource will be returned to the original manager when the {@link NDManager} is
+ * closed.
+ *
+ * @param manager the {@link NDManager} to be attached to
+ */
+ void tempAttach(NDManager manager);
+
+ /**
+ * Detaches the {@link NDResource} from current {@link NDManager}'s lifecycle.
+ *
+ *
This becomes un-managed and it is the user's responsibility to close this. Failure to
+ * close the resource might cause your machine to run out of native memory.
+ *
+ * @see NDManager
+ */
+ void detach();
+
+ /** {@inheritDoc} */
+ @Override
+ void close();
+}
diff --git a/api/src/main/java/ai/djl/nn/AbstractBlock.java b/api/src/main/java/ai/djl/nn/AbstractBlock.java
index 6d4c2c4ecd2..7f67d8431a6 100644
--- a/api/src/main/java/ai/djl/nn/AbstractBlock.java
+++ b/api/src/main/java/ai/djl/nn/AbstractBlock.java
@@ -30,7 +30,7 @@
import java.util.LinkedHashMap;
import java.util.List;
import java.util.Locale;
-import java.util.function.Function;
+import java.util.function.Predicate;
/**
* {@code AbstractBlock} is an abstract implementation of {@link Block}.
@@ -43,12 +43,11 @@
*
*
Define a version for serializing parameter and metadata and pass it to the parent
* constructor
- *
Use {@link AbstractBlock#addParameter(Parameter, Shape)} or {@link
- * AbstractBlock#addParameter(Parameter, Function)} to add parameters to your block in the
+ *
Use {@link AbstractBlock#addParameter(Parameter)} to add parameters to your block in the
* constructor if necessary.
*
Use {@link AbstractBlock#addChildBlock(String, Block)} to add child blocks if necessary.
- *
Override {@link AbstractBlock#getOutputShapes(NDManager, Shape[])} to determine the shape
- * of your custom block's output based on the input it will receive.
+ *
Override {@link Block#getOutputShapes(Shape[])} to determine the shape of your custom
+ * block's output based on the input it will receive.
*
Override {@link AbstractBlock#initializeChildBlocks(NDManager, DataType, Shape...)} if you
* added child blocks to initialize them based on the input shape your block will receive. You
* can skip this if your block does not contain child blocks
@@ -61,9 +60,9 @@
*
*
*
If you use {@link AbstractBlock#addParameter(Parameter)} to add parameters, you have to take
- * care of parameter initialization yourself. In this case, you need to override {@link
- * AbstractBlock#getParameterShape(String, Shape[])} to determine the shape of your parameters. If
- * you use the other variants of {@code addParameter} this is done for you.
+ * care of parameter initialization yourself. In this case, you need to setShape to your parameters
+ * if you know the shape of Parameter or you can implement prepare to setShape when you see the
+ * input shape.
*/
// Using LinkedHashMap instead of Map is intentional: we want to make sure that consumers
// of this API know the children and parameters are always iterated over in insertion order.
@@ -99,14 +98,6 @@ public abstract class AbstractBlock implements Block {
*/
protected LinkedHashMap parameters = new LinkedHashMap<>();
- /**
- * Callbacks to determine the shape of a parameter. Values may be null in which case extending
- * classes need to override {@link Block#getParameterShape(String, Shape[])} and implement
- * parameter shape resolution manually.
- */
- protected LinkedHashMap> parameterShapeCallbacks =
- new LinkedHashMap<>();
-
/**
* Builds an empty block with the given version for parameter serialization.
*
@@ -195,73 +186,20 @@ protected final B addChildBlock(String name, B block) {
return block;
}
- /**
- * Adds a parameter to this block. If parameters are added with this method, subclasses need to
- * override {@link Block#getParameterShape(String, Shape[])} and return the shapes of parameters
- * themselves.
- *
- * @param parameter the parameter to add, not null
- * @param
the specific parameter subclass
- * @return the parameter passed as arguments to make it easier to create and assign paramters in
- * one line
- */
- protected final
P addParameter(P parameter) {
- return addParameter(parameter, (Function) null);
- }
-
/**
* Adds a parameter to this block. If parameters are added with this method, intialization of
* the parameter works out of the box
*
- * @param parameter the parameter to add, not null
- * @param shape the shape of the parameter
* @param
the specific parameter subclass
- * @return the parameter passed as arguments to make it easier to create and assign paramters in
- * one line
- */
- protected final
P addParameter(P parameter, Shape shape) {
- return addParameter(parameter, (inputShapes) -> shape);
- }
-
- /**
- * Adds a parameter to this block. If parameters are added with this method, intialization of
- * the parameter works out of the box
- *
* @param parameter the parameter to add, not null
- * @param shapeCallback the method to call once the input shape of this block is known to
- * determine the shape of the given parameter
- * @param
the specific parameter subclass
* @return the parameter passed as arguments to make it easier to create and assign parameters
* in one line
*/
- protected final
P addParameter(
- P parameter, Function shapeCallback) {
+ protected final
P addParameter(P parameter) {
parameters.put(parameter.getName(), parameter);
- parameterShapeCallbacks.put(parameter.getName(), shapeCallback);
return parameter;
}
- /** {@inheritDoc} */
- @Override
- public Shape getParameterShape(String name, Shape[] inputShapes) {
- Function callback = parameterShapeCallbacks.get(name);
- if (callback == null) {
- Parameter parameter = parameters.get(name);
- if (parameter == null) {
- throw new IllegalArgumentException(
- "No parameter named " + name + " found in this block.");
- } else {
- throw new IllegalStateException(
- "No shape initializer for parameter "
- + name
- + "found. "
- + "Either pass an initializer for the shape when adding the "
- + "parameter or override getParameterShape in the subclass.");
- }
- }
- return callback.apply(inputShapes);
- }
-
/** {@inheritDoc} */
@Override
public BlockList getChildren() {
@@ -285,13 +223,9 @@ public PairList describeInput() {
/** {@inheritDoc} */
@Override
- public void setInitializer(Initializer initializer) {
- for (Parameter parameter : parameters.values()) {
- parameter.setInitializer(initializer, false);
- }
- for (Block child : children.values()) {
- child.setInitializer(initializer);
- }
+ public void setInitializer(Initializer initializer, Parameter.Type params) {
+ Predicate predicate = parameter -> parameter.getType().equals(params);
+ setInitializer(initializer, predicate);
}
/** {@inheritDoc} */
@@ -301,18 +235,50 @@ public void setInitializer(Initializer initializer, String paramName) {
if (parameter == null) {
throw new IllegalArgumentException("Could not find parameter " + paramName);
}
- parameter.setInitializer(initializer, true);
+ parameter.setInitializer(initializer);
}
/** {@inheritDoc} */
@Override
- public Shape[] initialize(NDManager manager, DataType dataType, Shape... inputShapes) {
+ public void setInitializer(Initializer initializer, Predicate predicate) {
+ List params = getParameters().values();
+ for (Parameter param : params) {
+ if (predicate.test(param)) {
+ param.setInitializer(initializer);
+ }
+ }
+ }
+
+ /** {@inheritDoc} */
+ @Override
+ public void initialize(NDManager manager, DataType dataType, Shape... inputShapes) {
beforeInitialize(inputShapes);
+ // if parameters are initialized, skip it
+ if (!isInitialized()) {
+ // setShape for all params
+ prepare(inputShapes);
+ }
for (Parameter parameter : parameters.values()) {
- parameter.initialize(manager, dataType, inputShapes);
+ parameter.initialize(manager, dataType);
}
initializeChildBlocks(manager, dataType, inputShapes);
- return getOutputShapes(manager, inputShapes);
+ }
+
+ /**
+ * Performs any action necessary before initialization. For example, keep the input information
+ * or verify the layout.
+ *
+ * @param inputShapes the expected shapes of the input
+ */
+ protected void beforeInitialize(Shape... inputShapes) {
+ if (inputNames.isEmpty()) {
+ // automatically assign input names
+ inputNames = new ArrayList<>();
+ for (int i = 0; i < inputShapes.length; ++i) {
+ inputNames.add("data" + i);
+ }
+ }
+ this.inputShapes = inputShapes;
}
/**
@@ -355,20 +321,11 @@ public ParameterList getDirectParameters() {
}
/**
- * Performs any action necessary before initialization.
+ * Sets the shape of {@link Parameter}s.
*
- * @param inputShapes the expected shapes of the input
+ * @param inputShapes the shapes of inputs
*/
- protected void beforeInitialize(Shape[] inputShapes) {
- if (inputNames.isEmpty()) {
- // automatically assign input names
- inputNames = new ArrayList<>();
- for (int i = 0; i < inputShapes.length; ++i) {
- inputNames.add("data" + i);
- }
- }
- this.inputShapes = inputShapes;
- }
+ protected void prepare(Shape[] inputShapes) {}
/** {@inheritDoc} */
@Override
@@ -494,7 +451,7 @@ public String toString() {
appendShape(sb, inputShapeDescription.values().toArray(new Shape[0]));
sb.append(" -> ");
Shape[] outputShapes =
- getOutputShapes(null, inputShapeDescription.values().toArray(new Shape[0]));
+ getOutputShapes(inputShapeDescription.values().toArray(new Shape[0]));
appendShape(sb, outputShapes);
} else {
sb.append("Uninitialized");
diff --git a/api/src/main/java/ai/djl/nn/AbstractSymbolBlock.java b/api/src/main/java/ai/djl/nn/AbstractSymbolBlock.java
index f870eea148f..fdd7fcc0d6c 100644
--- a/api/src/main/java/ai/djl/nn/AbstractSymbolBlock.java
+++ b/api/src/main/java/ai/djl/nn/AbstractSymbolBlock.java
@@ -12,7 +12,6 @@
*/
package ai.djl.nn;
-import ai.djl.ndarray.NDManager;
import ai.djl.ndarray.types.Shape;
/** {@code AbstractSymbolBlock} is an abstract implementation of {@link SymbolBlock}. */
@@ -29,7 +28,7 @@ public AbstractSymbolBlock(byte version) {
/** {@inheritDoc} */
@Override
- public Shape[] getOutputShapes(NDManager manager, Shape[] inputShapes) {
+ public Shape[] getOutputShapes(Shape[] inputShapes) {
throw new UnsupportedOperationException("not implement!");
}
}
diff --git a/api/src/main/java/ai/djl/nn/Block.java b/api/src/main/java/ai/djl/nn/Block.java
index 6febeff5f6f..4bd3fb09e2b 100644
--- a/api/src/main/java/ai/djl/nn/Block.java
+++ b/api/src/main/java/ai/djl/nn/Block.java
@@ -25,6 +25,7 @@
import java.io.DataInputStream;
import java.io.DataOutputStream;
import java.io.IOException;
+import java.util.function.Predicate;
/**
* A {@code Block} is a composable function that forms a neural network.
@@ -158,11 +159,12 @@ default NDList forward(
}
/**
- * Sets an {@link Initializer} to the block.
+ * Sets an {@link Initializer} to all the parameters that match parameter type in the block.
*
* @param initializer the initializer to set
+ * @param type the Parameter Type we want to setInitializer
*/
- void setInitializer(Initializer initializer);
+ void setInitializer(Initializer initializer, Parameter.Type type);
/**
* Sets an {@link Initializer} to the specified direct parameter of the block, overriding the
@@ -173,15 +175,22 @@ default NDList forward(
*/
void setInitializer(Initializer initializer, String paramName);
+ /**
+ * Sets an {@link Initializer} to all the parameters that match Predicate in the block.
+ *
+ * @param initializer the initializer to be set
+ * @param predicate predicate function to indicate parameters you want to set
+ */
+ void setInitializer(Initializer initializer, Predicate predicate);
+
/**
* Initializes the parameters of the block. This method must be called before calling `forward`.
*
* @param manager the NDManager to initialize the parameters
* @param dataType the datatype of the parameters
* @param inputShapes the shapes of the inputs to the block
- * @return the shapes of the outputs of the block
*/
- Shape[] initialize(NDManager manager, DataType dataType, Shape... inputShapes);
+ void initialize(NDManager manager, DataType dataType, Shape... inputShapes);
/**
* Returns a boolean whether the block is initialized.
@@ -232,25 +241,13 @@ default NDList forward(
*/
ParameterList getParameters();
- /**
- * Returns the shape of the specified direct parameter of this block given the shapes of the
- * input to the block.
- *
- * @param name the name of the parameter
- * @param inputShapes the shapes of the input to the block
- * @return the shape of the parameter specified
- * @throws IllegalArgumentException if the parameter name specified is invalid
- */
- Shape getParameterShape(String name, Shape[] inputShapes);
-
/**
* Returns the expected output shapes of the block for the specified input shapes.
*
- * @param manager an NDManager
* @param inputShapes the shapes of the inputs
* @return the expected output shapes of the block
*/
- Shape[] getOutputShapes(NDManager manager, Shape[] inputShapes);
+ Shape[] getOutputShapes(Shape[] inputShapes);
/**
* Writes the parameters of the block to the given outputStream.
diff --git a/api/src/main/java/ai/djl/nn/BlockFactory.java b/api/src/main/java/ai/djl/nn/BlockFactory.java
new file mode 100644
index 00000000000..c6747b0fe64
--- /dev/null
+++ b/api/src/main/java/ai/djl/nn/BlockFactory.java
@@ -0,0 +1,34 @@
+/*
+ * Copyright 2021 Amazon.com, Inc. or its affiliates. All Rights Reserved.
+ *
+ * Licensed under the Apache License, Version 2.0 (the "License"). You may not use this file except in compliance
+ * with the License. A copy of the License is located at
+ *
+ * http://aws.amazon.com/apache2.0/
+ *
+ * or in the "license" file accompanying this file. This file is distributed on an "AS IS" BASIS, WITHOUT WARRANTIES
+ * OR CONDITIONS OF ANY KIND, either express or implied. See the License for the specific language governing permissions
+ * and limitations under the License.
+ */
+package ai.djl.nn;
+
+import ai.djl.ndarray.NDManager;
+import ai.djl.repository.zoo.ModelZoo;
+import java.io.Serializable;
+
+/**
+ * Block factory is a component to make standard for block creating and saving procedure. Block
+ * factory design is intended to bypass the serialization of the blocks. This class can be used by
+ * {@link ModelZoo} or DJL Serving to recover the block to its uninitialized states. User should
+ * combine this method with the block.loadParameter to get the block with all parameters.
+ */
+public interface BlockFactory extends Serializable {
+
+ /**
+ * Constructs the uninitialized block.
+ *
+ * @param manager the manager to assign to block
+ * @return the uninitialized block
+ */
+ Block newBlock(NDManager manager);
+}
diff --git a/api/src/main/java/ai/djl/nn/LambdaBlock.java b/api/src/main/java/ai/djl/nn/LambdaBlock.java
index 939e0e09b29..1b6fc0d3bc5 100644
--- a/api/src/main/java/ai/djl/nn/LambdaBlock.java
+++ b/api/src/main/java/ai/djl/nn/LambdaBlock.java
@@ -70,11 +70,11 @@ protected NDList forwardInternal(
/** {@inheritDoc} */
@Override
- public Shape[] getOutputShapes(NDManager manager, Shape[] inputShapes) {
- try (NDManager subManager = manager.newSubManager()) {
+ public Shape[] getOutputShapes(Shape[] inputShapes) {
+ try (NDManager manager = NDManager.newBaseManager()) {
NDList input = new NDList(inputShapes.length);
for (Shape shape : inputShapes) {
- input.add(subManager.zeros(shape));
+ input.add(manager.zeros(shape));
}
NDList output = lambda.apply(input);
Shape[] outputShapes = new Shape[output.size()];
diff --git a/api/src/main/java/ai/djl/nn/ParallelBlock.java b/api/src/main/java/ai/djl/nn/ParallelBlock.java
index 92ce9f6349e..9d04569a63f 100644
--- a/api/src/main/java/ai/djl/nn/ParallelBlock.java
+++ b/api/src/main/java/ai/djl/nn/ParallelBlock.java
@@ -149,16 +149,16 @@ public void initializeChildBlocks(NDManager manager, DataType dataType, Shape...
/** {@inheritDoc} */
@Override
- public Shape[] getOutputShapes(NDManager manager, Shape[] inputShapes) {
+ public Shape[] getOutputShapes(Shape[] inputShapes) {
Preconditions.checkArgument(!children.isEmpty(), "The parallel block is empty");
- try (NDManager subManager = manager.newSubManager()) {
+ try (NDManager manager = NDManager.newBaseManager()) {
List inputs = new ArrayList<>();
for (Block block : children.values()) {
- Shape[] shapes = block.getOutputShapes(manager, inputShapes);
+ Shape[] shapes = block.getOutputShapes(inputShapes);
NDList output = new NDList(shapes.length);
for (Shape shape : shapes) {
- output.add(subManager.create(shape));
+ output.add(manager.create(shape));
}
inputs.add(output);
}
diff --git a/api/src/main/java/ai/djl/nn/Parameter.java b/api/src/main/java/ai/djl/nn/Parameter.java
index 7d3c2954cde..369111057c7 100644
--- a/api/src/main/java/ai/djl/nn/Parameter.java
+++ b/api/src/main/java/ai/djl/nn/Parameter.java
@@ -19,6 +19,7 @@
import ai.djl.ndarray.types.Shape;
import ai.djl.ndarray.types.SparseFormat;
import ai.djl.training.initializer.Initializer;
+import ai.djl.training.initializer.XavierInitializer;
import java.io.DataInputStream;
import java.io.DataOutputStream;
import java.io.IOException;
@@ -42,62 +43,23 @@ public class Parameter implements AutoCloseable {
private String id;
private String name;
- private Block block;
- private ParameterType type;
- private DataType mandatoryDataType;
+ private Shape shape;
+ private Type type;
private Initializer initializer;
private NDArray array;
private boolean requiresGrad;
private SparseFormat gradientFormat;
- /**
- * Creates a {@code Parameter} with the given name, and parameter type, and associated with the
- * given {@link Block}.
- *
- * @param name the name of the {@code Parameter}
- * @param block the block with which this {@code Parameter} is associated
- * @param type the type of this {@code Parameter}
- */
- public Parameter(String name, Block block, ParameterType type) {
- this(name, block, type, true, SparseFormat.DENSE);
- }
-
- /**
- * Creates a {@code Parameter} with the given name, and parameter type, and associated with the
- * given {@link Block}.
- *
- * @param name the name of the {@code Parameter}
- * @param block the block with which this {@code Parameter} is associated
- * @param type the type of this {@code Parameter}
- * @param requiresGrad whether this {@code Parameter} needs to compute gradients
- */
- public Parameter(String name, Block block, ParameterType type, boolean requiresGrad) {
- this(name, block, type, requiresGrad, SparseFormat.DENSE);
- }
-
- /**
- * Creates a {@code Parameter} with the given name, and parameter type, and associated with the
- * given {@link Block}.
- *
- * @param name the name of the {@code Parameter}
- * @param block the block with which this {@code Parameter} is associated
- * @param type the type of this {@code Parameter}
- * @param requireGrad whether this {@code Parameter} needs to compute gradients
- * @param gradientFormat the {@link SparseFormat} of the gradient array
- */
- public Parameter(
- String name,
- Block block,
- ParameterType type,
- boolean requireGrad,
- SparseFormat gradientFormat) {
+ Parameter(Builder builder) {
this.id = UUID.randomUUID().toString();
- this.name = name;
- this.block = block;
- this.type = type;
- this.requiresGrad = requireGrad;
- this.initializer = type.getInitializer();
- this.gradientFormat = gradientFormat;
+ this.name = builder.name;
+ this.shape = builder.shape;
+ this.type = builder.type;
+ this.array = builder.array;
+ this.requiresGrad = builder.requiresGrad;
+ this.initializer =
+ (builder.initializer != null) ? builder.initializer : type.getInitializer();
+ this.gradientFormat = builder.gradientFormat;
}
/**
@@ -123,7 +85,7 @@ public String getName() {
*
* @return the type of this {@code Parameter}
*/
- public ParameterType getType() {
+ public Type getType() {
return type;
}
@@ -133,10 +95,26 @@ public ParameterType getType() {
* @param array the {@link NDArray} that contains values of this {@code Parameter}
*/
public void setArray(NDArray array) {
+ if (shape != null) {
+ throw new IllegalStateException("array has been set! Use either setArray or setShape");
+ }
this.array = array;
+ shape = array.getShape();
array.setName(name);
}
+ /**
+ * Sets the shape of this {@code Parameter}.
+ *
+ * @param shape the shape of this {@code Parameter}
+ */
+ public void setShape(Shape shape) {
+ if (array != null) {
+ throw new IllegalStateException("array has been set! Use either setArray or setShape");
+ }
+ this.shape = shape;
+ }
+
/**
* Gets the values of this {@code Parameter} as an {@link NDArray}.
*
@@ -158,15 +136,6 @@ public boolean requireGradient() {
return requiresGrad;
}
- /**
- * Sets the mandatory data type for this {@code Parameter}.
- *
- * @param mandatoryDataType the mandatory data type for this {@code Parameter}
- */
- public void setMandatoryDataType(DataType mandatoryDataType) {
- this.mandatoryDataType = mandatoryDataType;
- }
-
/**
* Checks if this {@code Parameter} is initialized.
*
@@ -181,12 +150,9 @@ public boolean isInitialized() {
* flag is true, sets the initializer regardless.
*
* @param initializer the initializer to be set
- * @param overwrite if true, set the initializer regardless of whether its already set or not
*/
- public void setInitializer(Initializer initializer, boolean overwrite) {
- if (overwrite || this.initializer == null) {
- this.initializer = initializer;
- }
+ public void setInitializer(Initializer initializer) {
+ this.initializer = initializer;
}
/**
@@ -195,17 +161,12 @@ public void setInitializer(Initializer initializer, boolean overwrite) {
*
* @param manager an NDManager to create the arrays
* @param dataType the datatype of the {@code Parameter}
- * @param inputShapes the expected input shapes
*/
- public void initialize(NDManager manager, DataType dataType, Shape[] inputShapes) {
+ public void initialize(NDManager manager, DataType dataType) {
Objects.requireNonNull(initializer, "No initializer has been set");
+ Objects.requireNonNull(shape, "No parameter shape has been set");
if (!isInitialized()) {
- Shape shape = block.getParameterShape(name, inputShapes);
- array =
- initializer.initialize(
- manager,
- shape,
- mandatoryDataType == null ? dataType : mandatoryDataType);
+ array = initializer.initialize(manager, shape, dataType);
array.setName(name);
}
@@ -266,6 +227,8 @@ public void load(NDManager manager, DataInputStream dis)
}
array = manager.decode(dis);
+ // set the shape of the parameter and prepare() can be skipped
+ shape = array.getShape();
}
/** {@inheritDoc} */
@@ -276,4 +239,141 @@ public void close() {
array = null;
}
}
+
+ /**
+ * Creates a builder to build a {@code Parameter}.
+ *
+ *
The methods start with {@code set} are required fields, and {@code opt} for optional
+ * fields.
+ *
+ * @return a new builder
+ */
+ public static Parameter.Builder builder() {
+ return new Parameter.Builder();
+ }
+
+ /** Enumerates the types of {@link Parameter}. */
+ public enum Type {
+ WEIGHT(
+ new XavierInitializer(
+ XavierInitializer.RandomType.GAUSSIAN, XavierInitializer.FactorType.IN, 2)),
+ BIAS(Initializer.ZEROS),
+ GAMMA(Initializer.ONES),
+ BETA(Initializer.ZEROS),
+ RUNNING_MEAN(Initializer.ZEROS),
+ RUNNING_VAR(Initializer.ONES),
+ OTHER(null);
+
+ private final transient Initializer initializer;
+
+ Type(Initializer initializer) {
+ this.initializer = initializer;
+ }
+
+ /**
+ * Gets the {@link Initializer} of this {@code ParameterType}.
+ *
+ * @return the {@link Initializer} of this {@code ParameterType}
+ */
+ public Initializer getInitializer() {
+ return initializer;
+ }
+ }
+
+ /** A Builder to construct a {@code Parameter}. */
+ public static final class Builder {
+ String name;
+ Shape shape;
+ Type type;
+ Initializer initializer;
+ NDArray array;
+ boolean requiresGrad = true;
+ SparseFormat gradientFormat;
+
+ /**
+ * Sets the name of the {@code Parameter}.
+ *
+ * @param name the name of the {@code Parameter}
+ * @return this {@code Parameter}
+ */
+ public Builder setName(String name) {
+ this.name = name;
+ return this;
+ }
+
+ /**
+ * Sets the {@code Type} of the {@code Parameter}.
+ *
+ * @param type the {@code Type} of the {@code Parameter}
+ * @return this {@code Parameter}
+ */
+ public Builder setType(Type type) {
+ this.type = type;
+ return this;
+ }
+
+ /**
+ * Sets the shape of the {@code Parameter}.
+ *
+ * @param shape the shape of the {@code Parameter}
+ * @return this {@code Parameter}
+ */
+ public Builder optShape(Shape shape) {
+ this.shape = shape;
+ return this;
+ }
+
+ /**
+ * Sets the Initializer of the {@code Parameter}.
+ *
+ * @param initializer the Initializer of the {@code Parameter}
+ * @return this {@code Parameter}
+ */
+ public Builder optInitializer(Initializer initializer) {
+ this.initializer = initializer;
+ return this;
+ }
+
+ /**
+ * Sets the array of the {@code Parameter}.
+ *
+ * @param array the array of the {@code Parameter}
+ * @return this {@code Parameter}
+ */
+ public Builder optArray(NDArray array) {
+ this.array = array;
+ return this;
+ }
+
+ /**
+ * Sets if the {@code Parameter} requires gradient.
+ *
+ * @param requiresGrad if the {@code Parameter} requires gradient
+ * @return this {@code Parameter}
+ */
+ public Builder optRequiresGrad(boolean requiresGrad) {
+ this.requiresGrad = requiresGrad;
+ return this;
+ }
+
+ /**
+ * Sets the {@link SparseFormat} of the {@code Parameter}.
+ *
+ * @param gradientFormat the {@link SparseFormat} of the {@code Parameter}
+ * @return this {@code Parameter}
+ */
+ public Builder optGradientFormat(SparseFormat gradientFormat) {
+ this.gradientFormat = gradientFormat;
+ return this;
+ }
+
+ /**
+ * Builds a {@code Parameter} instance.
+ *
+ * @return the {@code Parameter} instance
+ */
+ public Parameter build() {
+ return new Parameter(this);
+ }
+ }
}
diff --git a/api/src/main/java/ai/djl/nn/ParameterType.java b/api/src/main/java/ai/djl/nn/ParameterType.java
deleted file mode 100644
index dde3cb23ab8..00000000000
--- a/api/src/main/java/ai/djl/nn/ParameterType.java
+++ /dev/null
@@ -1,41 +0,0 @@
-/*
- * Copyright 2019 Amazon.com, Inc. or its affiliates. All Rights Reserved.
- *
- * Licensed under the Apache License, Version 2.0 (the "License"). You may not use this file except in compliance
- * with the License. A copy of the License is located at
- *
- * http://aws.amazon.com/apache2.0/
- *
- * or in the "license" file accompanying this file. This file is distributed on an "AS IS" BASIS, WITHOUT WARRANTIES
- * OR CONDITIONS OF ANY KIND, either express or implied. See the License for the specific language governing permissions
- * and limitations under the License.
- */
-package ai.djl.nn;
-
-import ai.djl.training.initializer.Initializer;
-
-/** Enumerates the types of {@link Parameter}. */
-public enum ParameterType {
- WEIGHT(null),
- BIAS(Initializer.ZEROS),
- GAMMA(Initializer.ONES),
- BETA(Initializer.ZEROS),
- RUNNING_MEAN(Initializer.ZEROS),
- RUNNING_VAR(Initializer.ONES),
- OTHER(null);
-
- private final transient Initializer initializer;
-
- ParameterType(Initializer initializer) {
- this.initializer = initializer;
- }
-
- /**
- * Gets the {@link Initializer} of this {@code ParameterType}.
- *
- * @return the {@link Initializer} of this {@code ParameterType}
- */
- public Initializer getInitializer() {
- return initializer;
- }
-}
diff --git a/api/src/main/java/ai/djl/nn/SequentialBlock.java b/api/src/main/java/ai/djl/nn/SequentialBlock.java
index d0c6f168fb8..8908c1ad6c8 100644
--- a/api/src/main/java/ai/djl/nn/SequentialBlock.java
+++ b/api/src/main/java/ai/djl/nn/SequentialBlock.java
@@ -154,19 +154,20 @@ protected NDList forwardInternal(
public void initializeChildBlocks(NDManager manager, DataType dataType, Shape... inputShapes) {
Shape[] shapes = inputShapes;
for (Block child : getChildren().values()) {
- shapes = child.initialize(manager, dataType, shapes);
+ child.initialize(manager, dataType, shapes);
+ shapes = child.getOutputShapes(shapes);
}
}
/** {@inheritDoc} */
@Override
- public Shape[] getOutputShapes(NDManager manager, Shape[] inputs) {
+ public Shape[] getOutputShapes(Shape[] inputs) {
if (children.isEmpty()) {
throw new IllegalArgumentException("The sequential block is empty");
}
Shape[] current = inputs;
for (Block block : children.values()) {
- current = block.getOutputShapes(manager, current);
+ current = block.getOutputShapes(current);
}
return current;
}
diff --git a/api/src/main/java/ai/djl/nn/SymbolBlock.java b/api/src/main/java/ai/djl/nn/SymbolBlock.java
index b9e2ec73568..dca341e1eed 100644
--- a/api/src/main/java/ai/djl/nn/SymbolBlock.java
+++ b/api/src/main/java/ai/djl/nn/SymbolBlock.java
@@ -12,6 +12,7 @@
*/
package ai.djl.nn;
+import ai.djl.ndarray.NDManager;
import ai.djl.ndarray.types.Shape;
import ai.djl.util.PairList;
@@ -21,6 +22,16 @@
*/
public interface SymbolBlock extends Block {
+ /**
+ * Creates an empty SymbolBlock instance.
+ *
+ * @param manager the manager to be applied in the SymbolBlock
+ * @return a new Model instance
+ */
+ static SymbolBlock newInstance(NDManager manager) {
+ return manager.getEngine().newSymbolBlock(manager);
+ }
+
/** Removes the last block in the symbolic graph. */
default void removeLastBlock() {
throw new UnsupportedOperationException("not supported");
diff --git a/api/src/main/java/ai/djl/nn/convolutional/Convolution.java b/api/src/main/java/ai/djl/nn/convolutional/Convolution.java
index 6334935804c..1d211f1d903 100644
--- a/api/src/main/java/ai/djl/nn/convolutional/Convolution.java
+++ b/api/src/main/java/ai/djl/nn/convolutional/Convolution.java
@@ -16,13 +16,11 @@
import ai.djl.MalformedModelException;
import ai.djl.ndarray.NDArray;
import ai.djl.ndarray.NDList;
-import ai.djl.ndarray.NDManager;
import ai.djl.ndarray.types.LayoutType;
import ai.djl.ndarray.types.Shape;
import ai.djl.nn.AbstractBlock;
import ai.djl.nn.Block;
import ai.djl.nn.Parameter;
-import ai.djl.nn.ParameterType;
import ai.djl.training.ParameterStore;
import ai.djl.util.PairList;
import java.io.DataInputStream;
@@ -102,13 +100,17 @@ public Convolution(ConvolutionBuilder> builder) {
weight =
addParameter(
- new Parameter("weight", this, ParameterType.WEIGHT),
- (inputShapes) ->
- new Shape(filters, inputShapes[0].get(1)).addAll(kernelShape));
+ Parameter.builder()
+ .setName("weight")
+ .setType(Parameter.Type.WEIGHT)
+ .build());
if (includeBias) {
bias =
addParameter(
- new Parameter("bias", this, ParameterType.BIAS), new Shape(filters));
+ Parameter.builder()
+ .setName("bias")
+ .setType(Parameter.Type.BIAS)
+ .build());
}
}
@@ -149,15 +151,24 @@ protected NDList forwardInternal(
/** {@inheritDoc} */
@Override
- protected void beforeInitialize(Shape[] inputs) {
- super.beforeInitialize(inputs);
- Shape inputShape = inputs[0];
- Block.validateLayout(getExpectedLayout(), inputShape.getLayout());
+ protected void beforeInitialize(Shape... inputShapes) {
+ super.beforeInitialize(inputShapes);
+ Block.validateLayout(getExpectedLayout(), inputShapes[0].getLayout());
}
/** {@inheritDoc} */
@Override
- public Shape[] getOutputShapes(NDManager manager, Shape[] inputs) {
+ protected void prepare(Shape[] inputs) {
+ long inputChannel = inputs[0].get(1);
+ weight.setShape(new Shape(filters, inputChannel / groups).addAll(kernelShape));
+ if (bias != null) {
+ bias.setShape(new Shape(filters));
+ }
+ }
+
+ /** {@inheritDoc} */
+ @Override
+ public Shape[] getOutputShapes(Shape[] inputs) {
long[] shape = new long[numDimensions()];
shape[0] = inputs[0].get(0);
shape[1] = filters;
diff --git a/api/src/main/java/ai/djl/nn/convolutional/Deconvolution.java b/api/src/main/java/ai/djl/nn/convolutional/Deconvolution.java
index 890a4db9eff..10059208a5c 100644
--- a/api/src/main/java/ai/djl/nn/convolutional/Deconvolution.java
+++ b/api/src/main/java/ai/djl/nn/convolutional/Deconvolution.java
@@ -16,13 +16,11 @@
import ai.djl.MalformedModelException;
import ai.djl.ndarray.NDArray;
import ai.djl.ndarray.NDList;
-import ai.djl.ndarray.NDManager;
import ai.djl.ndarray.types.LayoutType;
import ai.djl.ndarray.types.Shape;
import ai.djl.nn.AbstractBlock;
import ai.djl.nn.Block;
import ai.djl.nn.Parameter;
-import ai.djl.nn.ParameterType;
import ai.djl.training.ParameterStore;
import ai.djl.util.PairList;
import java.io.DataInputStream;
@@ -78,13 +76,17 @@ public Deconvolution(DeconvolutionBuilder> builder) {
weight =
addParameter(
- new Parameter("weight", this, ParameterType.WEIGHT),
- (inputShapes) ->
- new Shape(filters, inputShapes[0].get(1)).addAll(kernelShape));
+ Parameter.builder()
+ .setName("weight")
+ .setType(Parameter.Type.WEIGHT)
+ .build());
if (includeBias) {
bias =
addParameter(
- new Parameter("bias", this, ParameterType.BIAS), new Shape(filters));
+ Parameter.builder()
+ .setName("bias")
+ .setType(Parameter.Type.BIAS)
+ .build());
}
}
@@ -126,15 +128,24 @@ protected NDList forwardInternal(
/** {@inheritDoc} */
@Override
- protected void beforeInitialize(Shape[] inputs) {
- super.beforeInitialize(inputs);
- Shape inputShape = inputs[0];
- Block.validateLayout(getExpectedLayout(), inputShape.getLayout());
+ protected void beforeInitialize(Shape... inputShapes) {
+ super.beforeInitialize(inputShapes);
+ Block.validateLayout(getExpectedLayout(), inputShapes[0].getLayout());
}
/** {@inheritDoc} */
@Override
- public Shape[] getOutputShapes(NDManager manager, Shape[] inputs) {
+ protected void prepare(Shape[] inputs) {
+ long inputChannel = inputs[0].get(1);
+ weight.setShape(new Shape(filters, inputChannel / groups).addAll(kernelShape));
+ if (bias != null) {
+ bias.setShape(new Shape(filters));
+ }
+ }
+
+ /** {@inheritDoc} */
+ @Override
+ public Shape[] getOutputShapes(Shape[] inputs) {
long[] shape = new long[numDimensions()];
shape[0] = inputs[0].get(0);
shape[1] = filters;
diff --git a/api/src/main/java/ai/djl/nn/core/ConstantEmbedding.java b/api/src/main/java/ai/djl/nn/core/ConstantEmbedding.java
index e2dceddcef4..ce5c486d701 100644
--- a/api/src/main/java/ai/djl/nn/core/ConstantEmbedding.java
+++ b/api/src/main/java/ai/djl/nn/core/ConstantEmbedding.java
@@ -57,7 +57,7 @@ protected NDList forwardInternal(
/** {@inheritDoc} */
@Override
- public Shape[] getOutputShapes(NDManager manager, Shape[] inputShapes) {
+ public Shape[] getOutputShapes(Shape[] inputShapes) {
return new Shape[] {inputShapes[0].addAll(embedding.getShape())};
}
diff --git a/api/src/main/java/ai/djl/nn/core/Embedding.java b/api/src/main/java/ai/djl/nn/core/Embedding.java
index 48736aaa8e1..2e65295c1f7 100644
--- a/api/src/main/java/ai/djl/nn/core/Embedding.java
+++ b/api/src/main/java/ai/djl/nn/core/Embedding.java
@@ -23,7 +23,6 @@
import ai.djl.nn.AbstractBlock;
import ai.djl.nn.Block;
import ai.djl.nn.Parameter;
-import ai.djl.nn.ParameterType;
import ai.djl.training.ParameterStore;
import ai.djl.util.PairList;
import java.io.DataInputStream;
@@ -56,8 +55,11 @@ protected Embedding(BaseBuilder baseBuilder) {
sparseFormat = baseBuilder.sparseFormat;
embedding =
addParameter(
- new Parameter("embedding", this, ParameterType.WEIGHT, true, sparseFormat),
- (inputShapes) -> new Shape(numEmbeddings, embeddingSize));
+ Parameter.builder()
+ .setName("embedding")
+ .setType(Parameter.Type.WEIGHT)
+ .optGradientFormat(sparseFormat)
+ .build());
if (baseBuilder.fallthrough != null && baseBuilder.defaultItem != null) {
throw new IllegalArgumentException(
"You can not specify both a fallthrough and a defaultItem");
@@ -93,15 +95,25 @@ public Embedding(NDArray embedding, SparseFormat format) {
this.sparseFormat = format;
this.embedding =
addParameter(
- new Parameter("embedding", this, ParameterType.WEIGHT, true, sparseFormat),
- (inputShapes) -> new Shape(numEmbeddings, embeddingSize));
+ Parameter.builder()
+ .setName("embedding")
+ .setType(Parameter.Type.WEIGHT)
+ .optGradientFormat(sparseFormat)
+ .build());
this.embedding.setArray(embedding);
inputShapes = new Shape[] {new Shape(-1)};
}
/** {@inheritDoc} */
@Override
- public Shape[] getOutputShapes(NDManager manager, Shape[] inputShapes) {
+ public void prepare(Shape[] inputShapes) {
+ // numItems will be adjusted by embedding array or fallthroughEmbedding
+ embedding.setShape(new Shape(numEmbeddings, embeddingSize));
+ }
+
+ /** {@inheritDoc} */
+ @Override
+ public Shape[] getOutputShapes(Shape[] inputShapes) {
return new Shape[] {inputShapes[0].addAll(new Shape(embeddingSize))};
}
diff --git a/api/src/main/java/ai/djl/nn/core/Linear.java b/api/src/main/java/ai/djl/nn/core/Linear.java
index 34a8c020a18..79459348fb2 100644
--- a/api/src/main/java/ai/djl/nn/core/Linear.java
+++ b/api/src/main/java/ai/djl/nn/core/Linear.java
@@ -16,12 +16,10 @@
import ai.djl.MalformedModelException;
import ai.djl.ndarray.NDArray;
import ai.djl.ndarray.NDList;
-import ai.djl.ndarray.NDManager;
import ai.djl.ndarray.types.Shape;
import ai.djl.nn.AbstractBlock;
import ai.djl.nn.Block;
import ai.djl.nn.Parameter;
-import ai.djl.nn.ParameterType;
import ai.djl.training.ParameterStore;
import ai.djl.util.PairList;
import ai.djl.util.Preconditions;
@@ -59,14 +57,19 @@ public class Linear extends AbstractBlock {
Linear(Builder builder) {
super(VERSION);
units = builder.units;
- // "inputFeatures" is only known after "beforeInitialize" is called, hence we need
- // a callback, even if we do not used the callback parameter
weight =
addParameter(
- new Parameter("weight", this, ParameterType.WEIGHT),
- inputShapes -> new Shape(units, inputFeatures));
+ Parameter.builder()
+ .setName("weight")
+ .setType(Parameter.Type.WEIGHT)
+ .build());
if (builder.bias) {
- bias = addParameter(new Parameter("bias", this, ParameterType.BIAS), new Shape(units));
+ bias =
+ addParameter(
+ Parameter.builder()
+ .setName("bias")
+ .setType(Parameter.Type.BIAS)
+ .build());
}
}
@@ -86,8 +89,8 @@ protected NDList forwardInternal(
/** {@inheritDoc} */
@Override
- public Shape[] getOutputShapes(NDManager manager, Shape[] inputs) {
- return new Shape[] {inputShape.addAll(new Shape(units))};
+ public Shape[] getOutputShapes(Shape[] inputs) {
+ return new Shape[] {inputs[0].slice(0, inputs[0].dimension() - 1).add(units)};
}
/** {@inheritDoc} */
@@ -99,13 +102,24 @@ public PairList describeInput() {
/** {@inheritDoc} */
@Override
- public void beforeInitialize(Shape[] inputShapes) {
+ protected void beforeInitialize(Shape... inputShapes) {
super.beforeInitialize(inputShapes);
+ Preconditions.checkArgument(inputShapes.length == 1, "Linear block only support 1 input");
Shape input = inputShapes[0];
inputFeatures = input.get(input.dimension() - 1);
inputShape = input.slice(0, input.dimension() - 1);
}
+ /** {@inheritDoc} */
+ @Override
+ public void prepare(Shape[] inputShapes) {
+ Shape input = inputShapes[0];
+ weight.setShape(new Shape(units, input.get(input.dimension() - 1)));
+ if (bias != null) {
+ bias.setShape(new Shape(units));
+ }
+ }
+
/** {@inheritDoc} */
@Override
protected void saveMetadata(DataOutputStream os) throws IOException {
diff --git a/api/src/main/java/ai/djl/nn/core/Prelu.java b/api/src/main/java/ai/djl/nn/core/Prelu.java
index abb268f9446..ced9d709e87 100644
--- a/api/src/main/java/ai/djl/nn/core/Prelu.java
+++ b/api/src/main/java/ai/djl/nn/core/Prelu.java
@@ -15,12 +15,10 @@
import ai.djl.MalformedModelException;
import ai.djl.ndarray.NDArray;
import ai.djl.ndarray.NDList;
-import ai.djl.ndarray.NDManager;
import ai.djl.ndarray.internal.NDArrayEx;
import ai.djl.ndarray.types.Shape;
import ai.djl.nn.AbstractBlock;
import ai.djl.nn.Parameter;
-import ai.djl.nn.ParameterType;
import ai.djl.training.ParameterStore;
import ai.djl.util.PairList;
import java.io.DataInputStream;
@@ -44,7 +42,13 @@ public class Prelu extends AbstractBlock {
/** Creates a Parametric ReLU Block. */
public Prelu() {
super(VERSION);
- alpha = addParameter(new Parameter("alpha", this, ParameterType.OTHER), new Shape());
+ alpha =
+ addParameter(
+ Parameter.builder()
+ .setName("alpha")
+ .setType(Parameter.Type.WEIGHT)
+ .optShape(new Shape())
+ .build());
}
/** {@inheritDoc} */
@@ -61,7 +65,7 @@ protected NDList forwardInternal(
/** {@inheritDoc} */
@Override
- public Shape[] getOutputShapes(NDManager manager, Shape[] inputs) {
+ public Shape[] getOutputShapes(Shape[] inputs) {
return new Shape[] {inputs[0]};
}
diff --git a/api/src/main/java/ai/djl/nn/norm/BatchNorm.java b/api/src/main/java/ai/djl/nn/norm/BatchNorm.java
index 7f5f243646b..dc93360811a 100644
--- a/api/src/main/java/ai/djl/nn/norm/BatchNorm.java
+++ b/api/src/main/java/ai/djl/nn/norm/BatchNorm.java
@@ -16,12 +16,10 @@
import ai.djl.MalformedModelException;
import ai.djl.ndarray.NDArray;
import ai.djl.ndarray.NDList;
-import ai.djl.ndarray.NDManager;
import ai.djl.ndarray.internal.NDArrayEx;
import ai.djl.ndarray.types.Shape;
import ai.djl.nn.AbstractBlock;
import ai.djl.nn.Parameter;
-import ai.djl.nn.ParameterType;
import ai.djl.training.ParameterStore;
import ai.djl.util.PairList;
import java.io.DataInputStream;
@@ -86,26 +84,37 @@ public class BatchNorm extends AbstractBlock {
momentum = builder.momentum;
center = builder.center;
scale = builder.scale;
- // When creating parameters we use a callback as "inChannels" is set before initialization,
- // it is not known yet.
+
// make gamma trainable if scale
gamma =
addParameter(
- new Parameter("gamma", this, ParameterType.GAMMA, scale),
- (inputShapes) -> new Shape(inChannels));
+ Parameter.builder()
+ .setName("gamma")
+ .setType(Parameter.Type.GAMMA)
+ .optRequiresGrad(scale)
+ .build());
// make beta trainable if center
beta =
addParameter(
- new Parameter("beta", this, ParameterType.BETA, center),
- (inputShapes) -> new Shape(inChannels));
+ Parameter.builder()
+ .setName("beta")
+ .setType(Parameter.Type.BETA)
+ .optRequiresGrad(center)
+ .build());
runningMean =
addParameter(
- new Parameter("runningMean", this, ParameterType.RUNNING_MEAN, false),
- (inputShapes) -> new Shape(inChannels));
+ Parameter.builder()
+ .setName("runningMean")
+ .setType(Parameter.Type.RUNNING_MEAN)
+ .optRequiresGrad(false)
+ .build());
runningVar =
addParameter(
- new Parameter("runningVar", this, ParameterType.RUNNING_VAR, false),
- (inputShapes) -> new Shape(inChannels));
+ Parameter.builder()
+ .setName("runningVar")
+ .setType(Parameter.Type.RUNNING_VAR)
+ .optRequiresGrad(false)
+ .build());
}
/** {@inheritDoc} */
@@ -135,17 +144,26 @@ protected NDList forwardInternal(
/** {@inheritDoc} */
@Override
- public Shape[] getOutputShapes(NDManager manager, Shape[] inputShapes) {
+ public Shape[] getOutputShapes(Shape[] inputShapes) {
return new Shape[] {inputShapes[0]};
}
/** {@inheritDoc} */
@Override
- public void beforeInitialize(Shape[] inputShapes) {
+ protected void beforeInitialize(Shape... inputShapes) {
super.beforeInitialize(inputShapes);
inChannels = inputShapes[0].size(axis);
}
+ /** {@inheritDoc} */
+ @Override
+ public void prepare(Shape[] inputShapes) {
+ gamma.setShape(new Shape(inChannels));
+ beta.setShape(new Shape(inChannels));
+ runningMean.setShape(new Shape(inChannels));
+ runningVar.setShape(new Shape(inChannels));
+ }
+
/** {@inheritDoc} */
@Override
protected void saveMetadata(DataOutputStream os) throws IOException {
diff --git a/api/src/main/java/ai/djl/nn/norm/Dropout.java b/api/src/main/java/ai/djl/nn/norm/Dropout.java
index 7a44c954288..20bcd201138 100644
--- a/api/src/main/java/ai/djl/nn/norm/Dropout.java
+++ b/api/src/main/java/ai/djl/nn/norm/Dropout.java
@@ -15,7 +15,6 @@
import ai.djl.MalformedModelException;
import ai.djl.ndarray.NDArray;
import ai.djl.ndarray.NDList;
-import ai.djl.ndarray.NDManager;
import ai.djl.ndarray.internal.NDArrayEx;
import ai.djl.ndarray.types.Shape;
import ai.djl.nn.AbstractBlock;
@@ -76,7 +75,7 @@ protected NDList forwardInternal(
/** {@inheritDoc} */
@Override
- public Shape[] getOutputShapes(NDManager manager, Shape[] inputShapes) {
+ public Shape[] getOutputShapes(Shape[] inputShapes) {
return new Shape[] {inputShapes[0]};
}
diff --git a/api/src/main/java/ai/djl/nn/recurrent/RecurrentBlock.java b/api/src/main/java/ai/djl/nn/recurrent/RecurrentBlock.java
index 1251c32ee49..c51620f5dc5 100644
--- a/api/src/main/java/ai/djl/nn/recurrent/RecurrentBlock.java
+++ b/api/src/main/java/ai/djl/nn/recurrent/RecurrentBlock.java
@@ -13,13 +13,13 @@
package ai.djl.nn.recurrent;
import ai.djl.MalformedModelException;
-import ai.djl.ndarray.NDManager;
import ai.djl.ndarray.types.LayoutType;
import ai.djl.ndarray.types.Shape;
import ai.djl.nn.AbstractBlock;
import ai.djl.nn.Block;
import ai.djl.nn.Parameter;
-import ai.djl.nn.ParameterType;
+import ai.djl.nn.ParameterList;
+import ai.djl.util.Pair;
import java.io.DataInputStream;
import java.io.IOException;
@@ -67,7 +67,7 @@ public RecurrentBlock(BaseBuilder> builder) {
bidirectional = builder.bidirectional;
returnState = builder.returnState;
- ParameterType[] parameterTypes = {ParameterType.WEIGHT, ParameterType.BIAS};
+ Parameter.Type[] parameterTypes = {Parameter.Type.WEIGHT, Parameter.Type.BIAS};
String[] directions = {"l"};
if (builder.bidirectional) {
directions = new String[] {"l", "r"};
@@ -75,12 +75,13 @@ public RecurrentBlock(BaseBuilder> builder) {
String[] gateStrings = {"i2h", "h2h"};
for (int i = 0; i < numLayers; i++) {
- for (ParameterType parameterType : parameterTypes) {
+ for (Parameter.Type parameterType : parameterTypes) {
for (String direction : directions) {
for (String gateString : gateStrings) {
String name =
direction + '_' + i + '_' + gateString + '_' + parameterType.name();
- addParameter(new Parameter(name, this, parameterType));
+ addParameter(
+ Parameter.builder().setName(name).setType(parameterType).build());
}
}
}
@@ -89,7 +90,7 @@ public RecurrentBlock(BaseBuilder> builder) {
/** {@inheritDoc} */
@Override
- public Shape[] getOutputShapes(NDManager manager, Shape[] inputs) {
+ public Shape[] getOutputShapes(Shape[] inputs) {
Shape inputShape = inputs[0];
Shape outputShape =
new Shape(inputShape.get(0), inputShape.get(1), stateSize * getNumDirections());
@@ -109,31 +110,34 @@ public Shape[] getOutputShapes(NDManager manager, Shape[] inputs) {
/** {@inheritDoc} */
@Override
- public void beforeInitialize(Shape[] inputs) {
- super.beforeInitialize(inputs);
- Shape inputShape = inputs[0];
- Block.validateLayout(EXPECTED_LAYOUT, inputShape.getLayout());
+ protected void beforeInitialize(Shape... inputShapes) {
+ super.beforeInitialize(inputShapes);
+ Block.validateLayout(EXPECTED_LAYOUT, inputShapes[0].getLayout());
}
/** {@inheritDoc} */
@Override
- public Shape getParameterShape(String name, Shape[] inputShapes) {
- int layer = Integer.parseInt(name.split("_")[1]);
- Shape shape = inputShapes[0];
- long inputs = shape.get(2);
- if (layer > 0) {
- inputs = stateSize * getNumDirections();
- }
- if (name.contains("BIAS")) {
- return new Shape(gates * stateSize);
- }
- if (name.contains("i2h")) {
- return new Shape(gates * stateSize, inputs);
- }
- if (name.contains("h2h")) {
- return new Shape(gates * stateSize, stateSize);
+ public void prepare(Shape[] inputs) {
+ Shape inputShape = inputs[0];
+ ParameterList parameters = getDirectParameters();
+ for (Pair pair : parameters) {
+ String name = pair.getKey();
+ Parameter parameter = pair.getValue();
+ int layer = Integer.parseInt(name.split("_")[1]);
+ long inputSize = inputShape.get(2);
+ if (layer > 0) {
+ inputSize = stateSize * getNumDirections();
+ }
+ if (name.contains("BIAS")) {
+ parameter.setShape(new Shape(gates * stateSize));
+ } else if (name.contains("i2h")) {
+ parameter.setShape(new Shape(gates * stateSize, inputSize));
+ } else if (name.contains("h2h")) {
+ parameter.setShape(new Shape(gates * stateSize, stateSize));
+ } else {
+ throw new IllegalArgumentException("Invalid parameter name");
+ }
}
- throw new IllegalArgumentException("Invalid parameter name");
}
/** {@inheritDoc} */
diff --git a/api/src/main/java/ai/djl/nn/transformer/BertBlock.java b/api/src/main/java/ai/djl/nn/transformer/BertBlock.java
index 066fc0c4229..868f6ace5fc 100644
--- a/api/src/main/java/ai/djl/nn/transformer/BertBlock.java
+++ b/api/src/main/java/ai/djl/nn/transformer/BertBlock.java
@@ -22,7 +22,6 @@
import ai.djl.nn.Activation;
import ai.djl.nn.Block;
import ai.djl.nn.Parameter;
-import ai.djl.nn.ParameterType;
import ai.djl.nn.core.Linear;
import ai.djl.nn.norm.BatchNorm;
import ai.djl.nn.norm.Dropout;
@@ -77,8 +76,12 @@ private BertBlock(Builder builder) {
// embedding for the position
this.positionEmebdding =
addParameter(
- new Parameter(PARAM_POSITION_EMBEDDING, this, ParameterType.WEIGHT),
- new Shape(builder.maxSequenceLength, builder.embeddingSize));
+ Parameter.builder()
+ .setName(PARAM_POSITION_EMBEDDING)
+ .setType(Parameter.Type.WEIGHT)
+ .optShape(
+ new Shape(builder.maxSequenceLength, builder.embeddingSize))
+ .build());
// embedding for the input types
this.typeEmbedding =
addChildBlock(
@@ -153,7 +156,7 @@ public int getTypeDictionarySize() {
/** {@inheritDoc} */
@Override
- public Shape[] getOutputShapes(NDManager manager, Shape[] inputShapes) {
+ public Shape[] getOutputShapes(Shape[] inputShapes) {
long batch = inputShapes[0].get(0);
long seqLength = inputShapes[0].get(1);
return new Shape[] {
@@ -164,11 +167,12 @@ public Shape[] getOutputShapes(NDManager manager, Shape[] inputShapes) {
/** {@inheritDoc} */
@Override
public void initializeChildBlocks(NDManager manager, DataType dataType, Shape... inputShapes) {
- beforeInitialize(inputShapes);
+ super.beforeInitialize(inputShapes);
inputNames = Arrays.asList("tokenIds", "typeIds", "masks");
Shape[] tokenShape = {inputShapes[0]};
Shape[] typeShape = {inputShapes[1]};
- Shape[] embeddingOutput = this.tokenEmbedding.initialize(manager, dataType, tokenShape);
+ this.tokenEmbedding.initialize(manager, dataType, tokenShape);
+ Shape[] embeddingOutput = this.tokenEmbedding.getOutputShapes(tokenShape);
this.typeEmbedding.initialize(manager, dataType, typeShape);
this.embeddingNorm.initialize(manager, dataType, embeddingOutput);
this.embeddingDropout.initialize(manager, dataType, embeddingOutput);
@@ -209,7 +213,8 @@ protected NDList forwardInternal(
NDArray typeIds = inputs.get(1);
// Third are the masks for the input
NDArray masks = inputs.get(2);
- MemoryScope initScope = MemoryScope.from(tokenIds).add(typeIds, masks);
+ NDManager initScope = NDManager.from(tokenIds);
+ initScope.tempAttachAll(inputs);
// Create embeddings for inputs
NDArray embeddedTokens =
tokenEmbedding.forward(ps, new NDList(tokenIds), training).singletonOrThrow();
@@ -237,16 +242,15 @@ protected NDList forwardInternal(
.mul(-100000f); // turn 1s (original 0s) into -100000
// Run through all transformer blocks
NDList lastOutput = dropoutEmbedding;
- initScope
- .remove(tokenIds, typeIds, masks)
- .waitToRead(dropoutEmbedding)
- .waitToRead(offsetMask)
- .close();
+ initScope.ret(lastOutput);
+ initScope.ret(offsetMask);
+ initScope.close();
for (final TransformerEncoderBlock block : transformerEncoderBlocks) {
NDList input = new NDList(lastOutput.head(), offsetMask);
- MemoryScope innerScope = MemoryScope.from(input);
- lastOutput = block.forward(ps, input, training);
- innerScope.remove(offsetMask).waitToRead(lastOutput).close();
+ try (NDManager innerScope = NDManager.from(input)) {
+ innerScope.tempAttachAll(input);
+ lastOutput = innerScope.ret(block.forward(ps, input, training));
+ }
}
// We also return the pooled output - this is an additional fully connected layer
// only applied to the first token, assumed to be the CLS token to be used for training
diff --git a/api/src/main/java/ai/djl/nn/transformer/BertMaskedLanguageModelBlock.java b/api/src/main/java/ai/djl/nn/transformer/BertMaskedLanguageModelBlock.java
index ab9a7216803..4c52e1e5f0a 100644
--- a/api/src/main/java/ai/djl/nn/transformer/BertMaskedLanguageModelBlock.java
+++ b/api/src/main/java/ai/djl/nn/transformer/BertMaskedLanguageModelBlock.java
@@ -19,7 +19,6 @@
import ai.djl.ndarray.types.Shape;
import ai.djl.nn.AbstractBlock;
import ai.djl.nn.Parameter;
-import ai.djl.nn.ParameterType;
import ai.djl.nn.core.Linear;
import ai.djl.nn.norm.BatchNorm;
import ai.djl.training.ParameterStore;
@@ -59,8 +58,11 @@ public BertMaskedLanguageModelBlock(
this.sequenceNorm = addChildBlock("sequenceNorm", BatchNorm.builder().optAxis(1).build());
this.dictionaryBias =
addParameter(
- new Parameter("dictionaryBias", this, ParameterType.BIAS),
- new Shape(bertBlock.getTokenDictionarySize()));
+ Parameter.builder()
+ .setName("dictionaryBias")
+ .setType(Parameter.Type.BIAS)
+ .optShape(new Shape(bertBlock.getTokenDictionarySize()))
+ .build());
this.hiddenActivation = hiddenActivation;
}
@@ -112,35 +114,37 @@ protected NDList forwardInternal(
NDArray sequenceOutput = inputs.get(0); // (B, S, E)
NDArray maskedIndices = inputs.get(1); // (B, I)
NDArray embeddingTable = inputs.get(2); // (D, E)
- MemoryScope scope = MemoryScope.from(sequenceOutput).add(maskedIndices);
- NDArray gatheredTokens = gatherFromIndices(sequenceOutput, maskedIndices); // (B * I, E)
- NDArray projectedTokens =
- hiddenActivation.apply(
- sequenceProjection
- .forward(ps, new NDList(gatheredTokens), training)
- .head()); // (B * I, E)
- NDArray normalizedTokens =
- sequenceNorm
- .forward(ps, new NDList(projectedTokens), training)
- .head(); // (B * I, E)
- // raw logits for each position to correspond to an entry in the embedding table
- NDArray embeddingTransposed = embeddingTable.transpose();
- embeddingTransposed.attach(gatheredTokens.getManager());
- NDArray logits = normalizedTokens.dot(embeddingTransposed); // (B * I, D)
- // we add an offset for each dictionary entry
- NDArray logitsWithBias =
- logits.add(ps.getValue(dictionaryBias, logits.getDevice(), training)); // (B * I, D)
- // now we apply log Softmax to get proper log probabilities
- NDArray logProbs = logitsWithBias.logSoftmax(1); // (B * I, D)
-
- scope.remove(sequenceOutput, maskedIndices).waitToRead(logProbs).close();
+ try (NDManager scope = NDManager.from(sequenceOutput)) {
+ scope.tempAttachAll(sequenceOutput, maskedIndices);
+ NDArray gatheredTokens = gatherFromIndices(sequenceOutput, maskedIndices); // (B * I, E)
+ NDArray projectedTokens =
+ hiddenActivation.apply(
+ sequenceProjection
+ .forward(ps, new NDList(gatheredTokens), training)
+ .head()); // (B * I, E)
+ NDArray normalizedTokens =
+ sequenceNorm
+ .forward(ps, new NDList(projectedTokens), training)
+ .head(); // (B * I, E)
+ // raw logits for each position to correspond to an entry in the embedding table
+ NDArray embeddingTransposed = embeddingTable.transpose();
+ embeddingTransposed.attach(gatheredTokens.getManager());
+ NDArray logits = normalizedTokens.dot(embeddingTransposed); // (B * I, D)
+ // we add an offset for each dictionary entry
+ NDArray logitsWithBias =
+ logits.add(
+ ps.getValue(
+ dictionaryBias, logits.getDevice(), training)); // (B * I, D)
+ // now we apply log Softmax to get proper log probabilities
+ NDArray logProbs = logitsWithBias.logSoftmax(1); // (B * I, D)
- return new NDList(logProbs);
+ return scope.ret(new NDList(logProbs));
+ }
}
/** {@inheritDoc} */
@Override
- public Shape[] getOutputShapes(final NDManager manager, final Shape[] inputShapes) {
+ public Shape[] getOutputShapes(final Shape[] inputShapes) {
int batchSize = (int) inputShapes[0].get(0);
int indexCount = (int) inputShapes[1].get(1);
int dictionarySize = (int) inputShapes[2].get(0);
diff --git a/api/src/main/java/ai/djl/nn/transformer/BertMaskedLanguageModelLoss.java b/api/src/main/java/ai/djl/nn/transformer/BertMaskedLanguageModelLoss.java
index 203fa1cf857..dc640e3d171 100644
--- a/api/src/main/java/ai/djl/nn/transformer/BertMaskedLanguageModelLoss.java
+++ b/api/src/main/java/ai/djl/nn/transformer/BertMaskedLanguageModelLoss.java
@@ -14,6 +14,7 @@
import ai.djl.ndarray.NDArray;
import ai.djl.ndarray.NDList;
+import ai.djl.ndarray.NDManager;
import ai.djl.ndarray.types.DataType;
import ai.djl.training.loss.Loss;
@@ -40,29 +41,30 @@ public BertMaskedLanguageModelLoss(int labelIdx, int maskIdx, int logProbsIdx) {
@Override
public NDArray evaluate(NDList labels, NDList predictions) {
- MemoryScope scope = MemoryScope.from(labels).add(predictions);
+ try (NDManager scope = NDManager.from(labels)) {
+ scope.tempAttachAll(labels, predictions);
- NDArray logProbs = predictions.get(logProbsIdx); // (B * I, D)
- int dictionarySize = (int) logProbs.getShape().get(1);
- NDArray targetIds = labels.get(labelIdx).flatten(); // (B * I)
- NDArray mask = labels.get(maskIdx).flatten().toType(DataType.FLOAT32, false); // (B * I)
- NDArray targetOneHots = targetIds.oneHot(dictionarySize);
- // Multiplying log_probs and one_hot_labels leaves the log probabilities of the correct
- // entries.
- // By summing we get the total predicition quality. We want to minimize the error,
- // so we negate the value - as we have logarithms, probability = 1 means log(prob) = 0,
- // the less sure we are the smaller the log value.
- NDArray perExampleLoss = logProbs.mul(targetOneHots).sum(new int[] {1}).mul(-1);
- // Multiplying log_probs and one_hot_labels leaves the log probabilities of the correct
- // entries.
- // By summing we get the total prediction quality.
- NDArray numerator = perExampleLoss.mul(mask).sum();
- // We normalize the loss by the actual number of predictions we had to make
- NDArray denominator = mask.sum().add(1e-5f);
- NDArray result = numerator.div(denominator);
+ NDArray logProbs = predictions.get(logProbsIdx); // (B * I, D)
+ int dictionarySize = (int) logProbs.getShape().get(1);
+ NDArray targetIds = labels.get(labelIdx).flatten(); // (B * I)
+ NDArray mask = labels.get(maskIdx).flatten().toType(DataType.FLOAT32, false); // (B * I)
+ NDArray targetOneHots = targetIds.oneHot(dictionarySize);
+ // Multiplying log_probs and one_hot_labels leaves the log probabilities of the correct
+ // entries.
+ // By summing we get the total predicition quality. We want to minimize the error,
+ // so we negate the value - as we have logarithms, probability = 1 means log(prob) = 0,
+ // the less sure we are the smaller the log value.
+ NDArray perExampleLoss = logProbs.mul(targetOneHots).sum(new int[] {1}).mul(-1);
+ // Multiplying log_probs and one_hot_labels leaves the log probabilities of the correct
+ // entries.
+ // By summing we get the total prediction quality.
+ NDArray numerator = perExampleLoss.mul(mask).sum();
+ // We normalize the loss by the actual number of predictions we had to make
+ NDArray denominator = mask.sum().add(1e-5f);
+ NDArray result = numerator.div(denominator);
- scope.remove(labels, predictions).waitToRead(result).close();
- return result;
+ return scope.ret(result);
+ }
}
/**
@@ -73,19 +75,19 @@ public NDArray evaluate(NDList labels, NDList predictions) {
* @return the percentage of correctly predicted masked tokens
*/
public NDArray accuracy(NDList labels, NDList predictions) {
- MemoryScope scope = MemoryScope.from(labels).add(predictions);
+ try (NDManager scope = NDManager.from(labels)) {
+ scope.tempAttachAll(labels, predictions);
- NDArray mask = labels.get(maskIdx).flatten(); // (B * I)
- NDArray targetIds = labels.get(labelIdx).flatten(); // (B * I)
- NDArray logProbs = predictions.get(logProbsIdx); // (B * I, D)
- NDArray predictedIs = logProbs.argMax(1).toType(DataType.INT32, false); // (B * I)
- NDArray equal = predictedIs.eq(targetIds).mul(mask);
- NDArray equalCount = equal.sum().toType(DataType.FLOAT32, false);
- NDArray count = mask.sum().toType(DataType.FLOAT32, false);
- NDArray result = equalCount.div(count);
+ NDArray mask = labels.get(maskIdx).flatten(); // (B * I)
+ NDArray targetIds = labels.get(labelIdx).flatten(); // (B * I)
+ NDArray logProbs = predictions.get(logProbsIdx); // (B * I, D)
+ NDArray predictedIs = logProbs.argMax(1).toType(DataType.INT32, false); // (B * I)
+ NDArray equal = predictedIs.eq(targetIds).mul(mask);
+ NDArray equalCount = equal.sum().toType(DataType.FLOAT32, false);
+ NDArray count = mask.sum().toType(DataType.FLOAT32, false);
+ NDArray result = equalCount.div(count);
- scope.remove(labels, predictions).waitToRead(result);
-
- return result;
+ return scope.ret(result);
+ }
}
}
diff --git a/api/src/main/java/ai/djl/nn/transformer/BertNextSentenceBlock.java b/api/src/main/java/ai/djl/nn/transformer/BertNextSentenceBlock.java
index 40f9a717b65..aac91ab5745 100644
--- a/api/src/main/java/ai/djl/nn/transformer/BertNextSentenceBlock.java
+++ b/api/src/main/java/ai/djl/nn/transformer/BertNextSentenceBlock.java
@@ -53,7 +53,7 @@ protected NDList forwardInternal(
/** {@inheritDoc} */
@Override
- public Shape[] getOutputShapes(NDManager manager, Shape[] inputShapes) {
+ public Shape[] getOutputShapes(Shape[] inputShapes) {
return new Shape[] {new Shape(inputShapes[0].get(0), 2)};
}
}
diff --git a/api/src/main/java/ai/djl/nn/transformer/BertNextSentenceLoss.java b/api/src/main/java/ai/djl/nn/transformer/BertNextSentenceLoss.java
index 0916e096c1c..b11e2828d82 100644
--- a/api/src/main/java/ai/djl/nn/transformer/BertNextSentenceLoss.java
+++ b/api/src/main/java/ai/djl/nn/transformer/BertNextSentenceLoss.java
@@ -14,6 +14,7 @@
import ai.djl.ndarray.NDArray;
import ai.djl.ndarray.NDList;
+import ai.djl.ndarray.NDManager;
import ai.djl.ndarray.types.DataType;
import ai.djl.training.loss.Loss;
@@ -38,20 +39,21 @@ public BertNextSentenceLoss(int labelIdx, int nextSentencePredictionIdx) {
@Override
public NDArray evaluate(NDList labels, NDList predictions) {
- MemoryScope scope = MemoryScope.from(labels).add(predictions);
- NDArray label = labels.get(labelIdx).toType(DataType.FLOAT32, false);
- // predictions are log(softmax)
- NDArray logPredictions = predictions.get(nextSentencePredictionIdx);
- NDArray oneHotLabels = label.oneHot(2);
- // we use negative log likelihood as loss: log(softmax) turns high confidence into
- // negative values near one, low confidence into negative values near -inf,
- // negating gives almost 0 for high confidence and near +inf for very low confidence
- NDArray logPredictionForLabels = oneHotLabels.mul(logPredictions);
- NDArray summedPredictions = logPredictionForLabels.sum(new int[] {1});
- NDArray perExampleLoss = summedPredictions.mul(-1f);
- NDArray result = perExampleLoss.mean();
- scope.remove(labels, predictions).waitToRead(result).close();
- return result;
+ try (NDManager scope = NDManager.from(labels)) {
+ scope.tempAttachAll(labels, predictions);
+ NDArray label = labels.get(labelIdx).toType(DataType.FLOAT32, false);
+ // predictions are log(softmax)
+ NDArray logPredictions = predictions.get(nextSentencePredictionIdx);
+ NDArray oneHotLabels = label.oneHot(2);
+ // we use negative log likelihood as loss: log(softmax) turns high confidence into
+ // negative values near one, low confidence into negative values near -inf,
+ // negating gives almost 0 for high confidence and near +inf for very low confidence
+ NDArray logPredictionForLabels = oneHotLabels.mul(logPredictions);
+ NDArray summedPredictions = logPredictionForLabels.sum(new int[] {1});
+ NDArray perExampleLoss = summedPredictions.mul(-1f);
+ NDArray result = perExampleLoss.mean();
+ return scope.ret(result);
+ }
}
/**
@@ -62,15 +64,16 @@ public NDArray evaluate(NDList labels, NDList predictions) {
* @return the fraction of correct predictions.
*/
public NDArray accuracy(NDList labels, NDList predictions) {
- MemoryScope scope = MemoryScope.from(labels).add(predictions);
- NDArray label = labels.get(labelIdx);
- NDArray predictionLogProbs = predictions.get(nextSentencePredictionIdx);
- // predictions are log(softmax) -> highest confidence is highest (negative) value near 0
- NDArray prediction = predictionLogProbs.argMax(1).toType(DataType.INT32, false);
- NDArray equalCount = label.eq(prediction).sum().toType(DataType.FLOAT32, false);
- NDArray result = equalCount.div(label.getShape().size());
- scope.remove(labels, predictions).waitToRead(result).close();
+ try (NDManager scope = NDManager.from(labels)) {
+ scope.tempAttachAll(labels, predictions);
+ NDArray label = labels.get(labelIdx);
+ NDArray predictionLogProbs = predictions.get(nextSentencePredictionIdx);
+ // predictions are log(softmax) -> highest confidence is highest (negative) value near 0
+ NDArray prediction = predictionLogProbs.argMax(1).toType(DataType.INT32, false);
+ NDArray equalCount = label.eq(prediction).sum().toType(DataType.FLOAT32, false);
+ NDArray result = equalCount.div(label.getShape().size());
- return result;
+ return scope.ret(result);
+ }
}
}
diff --git a/api/src/main/java/ai/djl/nn/transformer/BertPretrainingBlock.java b/api/src/main/java/ai/djl/nn/transformer/BertPretrainingBlock.java
index 2478811d037..5e60f28c25c 100644
--- a/api/src/main/java/ai/djl/nn/transformer/BertPretrainingBlock.java
+++ b/api/src/main/java/ai/djl/nn/transformer/BertPretrainingBlock.java
@@ -51,7 +51,8 @@ public BertPretrainingBlock(final BertBlock.Builder builder) {
@Override
public void initializeChildBlocks(NDManager manager, DataType dataType, Shape... inputShapes) {
inputNames = Arrays.asList("tokenIds", "typeIds", "sequenceMasks", "maskedIndices");
- Shape[] bertOutputShapes = bertBlock.initialize(manager, dataType, inputShapes);
+ bertBlock.initialize(manager, dataType, inputShapes);
+ Shape[] bertOutputShapes = bertBlock.getOutputShapes(inputShapes);
Shape embeddedSequence = bertOutputShapes[0];
Shape pooledOutput = bertOutputShapes[1];
Shape maskedIndices = inputShapes[2];
@@ -69,35 +70,36 @@ protected NDList forwardInternal(
NDArray typeIds = inputs.get(1);
NDArray sequenceMasks = inputs.get(2);
NDArray maskedIndices = inputs.get(3);
- MemoryScope scope = MemoryScope.from(tokenIds).add(typeIds, sequenceMasks, maskedIndices);
- // run the core bert model
- NDList bertResult =
- bertBlock.forward(ps, new NDList(tokenIds, typeIds, sequenceMasks), training);
- NDArray embeddedSequence = bertResult.get(0);
- NDArray pooledOutput = bertResult.get(1);
- // apply pooled output to the classifier
- NDArray nextSentenceProbabilities =
- nsBlock.forward(ps, new NDList(pooledOutput), training).singletonOrThrow();
- // de-mask masked tokens
- NDArray embeddingTable =
- bertBlock.getTokenEmbedding().getValue(ps, embeddedSequence.getDevice(), training);
- NDArray logProbs =
- mlBlock.forward(
- ps,
- new NDList(embeddedSequence, maskedIndices, embeddingTable),
- training)
- .singletonOrThrow();
+ try (NDManager scope = NDManager.from(tokenIds)) {
+ scope.tempAttachAll(inputs);
+ // run the core bert model
+ NDList bertResult =
+ bertBlock.forward(ps, new NDList(tokenIds, typeIds, sequenceMasks), training);
+ NDArray embeddedSequence = bertResult.get(0);
+ NDArray pooledOutput = bertResult.get(1);
+ // apply pooled output to the classifier
+ NDArray nextSentenceProbabilities =
+ nsBlock.forward(ps, new NDList(pooledOutput), training).singletonOrThrow();
+ // de-mask masked tokens
+ NDArray embeddingTable =
+ bertBlock
+ .getTokenEmbedding()
+ .getValue(ps, embeddedSequence.getDevice(), training);
+ NDArray logProbs =
+ mlBlock.forward(
+ ps,
+ new NDList(embeddedSequence, maskedIndices, embeddingTable),
+ training)
+ .singletonOrThrow();
- scope.remove(tokenIds, typeIds, sequenceMasks, maskedIndices)
- .waitToRead(nextSentenceProbabilities, logProbs)
- .close();
- // return the next sentence & masked language result to apply the loss to
- return new NDList(nextSentenceProbabilities, logProbs);
+ // return the next sentence & masked language result to apply the loss to
+ return scope.ret(new NDList(nextSentenceProbabilities, logProbs));
+ }
}
/** {@inheritDoc} */
@Override
- public Shape[] getOutputShapes(NDManager manager, Shape[] inputShapes) {
+ public Shape[] getOutputShapes(Shape[] inputShapes) {
long batchSize = inputShapes[0].get(0);
long maskedIndexCount = inputShapes[3].get(1);
return new Shape[] {
diff --git a/api/src/main/java/ai/djl/nn/transformer/IdEmbedding.java b/api/src/main/java/ai/djl/nn/transformer/IdEmbedding.java
index 4aa6484f766..2b3c52d3526 100644
--- a/api/src/main/java/ai/djl/nn/transformer/IdEmbedding.java
+++ b/api/src/main/java/ai/djl/nn/transformer/IdEmbedding.java
@@ -21,7 +21,6 @@
import ai.djl.nn.AbstractBlock;
import ai.djl.nn.Block;
import ai.djl.nn.Parameter;
-import ai.djl.nn.ParameterType;
import ai.djl.training.ParameterStore;
import ai.djl.util.PairList;
import java.util.Arrays;
@@ -47,13 +46,16 @@ private IdEmbedding(Builder builder) {
this.embeddingSize = builder.embeddingSize;
this.embedding =
addParameter(
- new Parameter(EMBEDDING_PARAM_NAME, this, ParameterType.WEIGHT),
- new Shape(dictionarySize, embeddingSize));
+ Parameter.builder()
+ .setName(EMBEDDING_PARAM_NAME)
+ .setType(Parameter.Type.WEIGHT)
+ .optShape(new Shape(dictionarySize, embeddingSize))
+ .build());
}
/** {@inheritDoc} */
@Override
- public Shape[] getOutputShapes(NDManager manager, Shape[] inputShapes) {
+ public Shape[] getOutputShapes(Shape[] inputShapes) {
return new Shape[] {inputShapes[0].addAll(new Shape(embeddingSize))};
}
diff --git a/api/src/main/java/ai/djl/nn/transformer/MemoryScope.java b/api/src/main/java/ai/djl/nn/transformer/MemoryScope.java
deleted file mode 100644
index e1bf4ca159a..00000000000
--- a/api/src/main/java/ai/djl/nn/transformer/MemoryScope.java
+++ /dev/null
@@ -1,168 +0,0 @@
-/*
- * Copyright 2020 Amazon.com, Inc. or its affiliates. All Rights Reserved.
- *
- * Licensed under the Apache License, Version 2.0 (the "License"). You may not use this file except in compliance
- * with the License. A copy of the License is located at
- *
- * http://aws.amazon.com/apache2.0/
- *
- * or in the "license" file accompanying this file. This file is distributed on an "AS IS" BASIS, WITHOUT WARRANTIES
- * OR CONDITIONS OF ANY KIND, either express or implied. See the License for the specific language governing permissions
- * and limitations under the License.
- */
-package ai.djl.nn.transformer;
-
-import ai.djl.ndarray.LazyNDArray;
-import ai.djl.ndarray.NDArray;
-import ai.djl.ndarray.NDList;
-import ai.djl.ndarray.NDManager;
-
-/**
- * Helper class for more complicated memory management scenarios. Allows to avoid boilerplate for
- * memory handling. Makes sure the sub NDManager used is connected to the correct GPU to avoid
- * crashes.
- */
-public final class MemoryScope implements AutoCloseable {
-
- private NDManager parentManager;
- private NDManager subManager;
-
- private MemoryScope(NDManager parentManager, NDManager subManager) {
- this.parentManager = parentManager;
- this.subManager = subManager;
- }
-
- /**
- * Adds all arrays in the given lists to this memory scope.
- *
- * @param lists the lists whose arrays to add to this scope, may be empty
- * @return this scope
- */
- public MemoryScope add(NDList... lists) {
- for (NDList list : lists) {
- list.attach(subManager);
- }
- return this;
- }
-
- /**
- * Adds the given arrays to this scopes sub manager.
- *
- * @param arrays the arrays to add
- * @return this scope
- */
- public MemoryScope add(NDArray... arrays) {
- for (NDArray array : arrays) {
- array.attach(subManager);
- }
- return this;
- }
-
- /**
- * Remove the given arrays from this scope and attach them back to this scopes parent NDManager.
- *
- * @param lists the lists containing the arrays to remove
- * @return this scope
- */
- public MemoryScope remove(NDList... lists) {
- for (NDList list : lists) {
- list.attach(parentManager);
- }
- return this;
- }
-
- /**
- * Remove the given arrays from this scope and attach them back to this scopes parent NDManager.
- *
- * @param arrays arrays to remove
- * @return this scope
- */
- public MemoryScope remove(NDArray... arrays) {
- for (NDArray array : arrays) {
- array.attach(parentManager);
- }
- return this;
- }
-
- /**
- * Returns the NDManager used to manage this scopes resources.
- *
- * @return the NDManager used to manage this scopes resources
- */
- public NDManager getScopeManager() {
- return subManager;
- }
-
- /**
- * Waits for all given arrays to be ready to read, i.e. waits for pending computations that
- * write to them, then removes them from this scope.
- *
- * @param arrays arrays to wait for
- * @return this scope
- */
- public MemoryScope waitToRead(NDArray... arrays) {
- for (NDArray array : arrays) {
- if (array instanceof LazyNDArray) {
- LazyNDArray lazyNDArray = (LazyNDArray) array;
- lazyNDArray.waitToRead();
- }
- remove(array);
- }
- return this;
- }
-
- /**
- * Waits for all arrays in all given lists to be ready to be read, i.e. waits for pending
- * computations that write to them, then removes them from this scope.
- *
- * @param lists may be empty
- * @return this scope
- */
- public MemoryScope waitToRead(NDList... lists) {
- for (NDList list : lists) {
- if (list != null) {
- for (NDArray array : list) {
- waitToRead(array);
- }
- }
- }
- return this;
- }
-
- /**
- * Closes this scope by closing the sub manager used to manage it. This causes all arrays still
- * attached to this scope to be closed as well.
- */
- @Override
- public void close() {
- subManager.close();
- }
-
- /**
- * Creates a new memory scope for the device of the given array and adds the array.
- *
- * @param ndArray an array
- * @return a new memory scrope containing the array
- */
- public static MemoryScope from(final NDArray ndArray) {
- return new MemoryScope(
- ndArray.getManager(),
- ndArray.getManager().newSubManager(ndArray.getDevice()))
- .add(ndArray);
- }
-
- /**
- * Creates a new memory scope that fits the device of the first array in the given list, adds
- * all arrays in the given list.
- *
- * @param list a list of arrays, must not be empty
- * @return a new memory scope
- */
- public static MemoryScope from(final NDList list) {
- NDArray ndArray = list.head();
- return new MemoryScope(
- ndArray.getManager(),
- ndArray.getManager().newSubManager(ndArray.getDevice()))
- .add(list);
- }
-}
diff --git a/api/src/main/java/ai/djl/nn/transformer/PointwiseFeedForwardBlock.java b/api/src/main/java/ai/djl/nn/transformer/PointwiseFeedForwardBlock.java
index 10d4aa20b40..9d3367f9cf5 100644
--- a/api/src/main/java/ai/djl/nn/transformer/PointwiseFeedForwardBlock.java
+++ b/api/src/main/java/ai/djl/nn/transformer/PointwiseFeedForwardBlock.java
@@ -32,8 +32,6 @@ public class PointwiseFeedForwardBlock extends AbstractBlock {
private static final byte VERSION = 1;
- private Shape outputShape;
-
/**
* Creates a pointwise feed-forward block.
*
@@ -49,7 +47,7 @@ public PointwiseFeedForwardBlock(
super(VERSION);
// add hidden layers with activation
int count = 0;
- for (final int hiddenSize : hiddenSizes) {
+ for (int hiddenSize : hiddenSizes) {
addChildBlock(
"linear_" + count, Linear.builder().optBias(true).setUnits(hiddenSize).build());
addChildBlock("activation_" + count, new LambdaBlock(activationFunction));
@@ -61,8 +59,11 @@ public PointwiseFeedForwardBlock(
/** {@inheritDoc} */
@Override
- public Shape[] getOutputShapes(NDManager manager, Shape[] inputShapes) {
- return new Shape[] {outputShape};
+ public Shape[] getOutputShapes(Shape[] inputShapes) {
+ for (Block child : children.values()) {
+ inputShapes = child.getOutputShapes(inputShapes);
+ }
+ return inputShapes;
}
/** {@inheritDoc} */
@@ -75,16 +76,16 @@ public void initializeChildBlocks(NDManager manager, DataType dataType, Shape...
}
// Now that we know the input shape, we can determine the reshape necessary
// to shape the input and re-shape the output
- final Shape inputShape = inputShapes[0];
+ Shape inputShape = inputShapes[0];
if (inputShape.dimension() < 2) {
throw new IllegalArgumentException(
"Pointwise feed forward blocks need an input of at least dimension 2.");
}
Shape lastShape = inputShape;
for (Block child : children.values()) {
- lastShape = child.initialize(manager, dataType, lastShape)[0];
+ child.initialize(manager, dataType, lastShape);
+ lastShape = getOutputShapes(new Shape[] {lastShape})[0];
}
- outputShape = lastShape;
}
/** {@inheritDoc} */
diff --git a/api/src/main/java/ai/djl/nn/transformer/ScaledDotProductAttentionBlock.java b/api/src/main/java/ai/djl/nn/transformer/ScaledDotProductAttentionBlock.java
index ffee4df219c..fa293f4b37a 100644
--- a/api/src/main/java/ai/djl/nn/transformer/ScaledDotProductAttentionBlock.java
+++ b/api/src/main/java/ai/djl/nn/transformer/ScaledDotProductAttentionBlock.java
@@ -146,7 +146,7 @@ public Linear getResultProjection() {
/** {@inheritDoc} */
@Override
- public Shape[] getOutputShapes(NDManager manager, Shape[] inputShapes) {
+ public Shape[] getOutputShapes(Shape[] inputShapes) {
// Return shape is the shape of the query. For 2 or less inputs we have self-attention, i.e.
// the shape of the output is the shape of the input
if (inputShapes.length == 1 || inputShapes.length == 2) {
diff --git a/api/src/main/java/ai/djl/nn/transformer/TransformerEncoderBlock.java b/api/src/main/java/ai/djl/nn/transformer/TransformerEncoderBlock.java
index 83fb87446e9..4cbabecada7 100644
--- a/api/src/main/java/ai/djl/nn/transformer/TransformerEncoderBlock.java
+++ b/api/src/main/java/ai/djl/nn/transformer/TransformerEncoderBlock.java
@@ -82,7 +82,7 @@ public TransformerEncoderBlock(
/** {@inheritDoc} */
@Override
- public Shape[] getOutputShapes(NDManager manager, Shape[] inputShapes) {
+ public Shape[] getOutputShapes(Shape[] inputShapes) {
return inputShapes;
}
diff --git a/api/src/main/java/ai/djl/training/DefaultTrainingConfig.java b/api/src/main/java/ai/djl/training/DefaultTrainingConfig.java
index be9f7bbfec6..b9580914201 100644
--- a/api/src/main/java/ai/djl/training/DefaultTrainingConfig.java
+++ b/api/src/main/java/ai/djl/training/DefaultTrainingConfig.java
@@ -13,23 +13,23 @@
package ai.djl.training;
import ai.djl.Device;
+import ai.djl.nn.Parameter;
import ai.djl.training.evaluator.Evaluator;
import ai.djl.training.initializer.Initializer;
-import ai.djl.training.initializer.XavierInitializer;
-import ai.djl.training.initializer.XavierInitializer.FactorType;
-import ai.djl.training.initializer.XavierInitializer.RandomType;
import ai.djl.training.listener.TrainingListener;
import ai.djl.training.loss.Loss;
import ai.djl.training.optimizer.Adam;
import ai.djl.training.optimizer.Optimizer;
+import ai.djl.util.PairList;
import java.util.ArrayList;
import java.util.Arrays;
import java.util.List;
+import java.util.function.Predicate;
/** {@code DefaultTrainingConfig} is an implementation of the {@link TrainingConfig} interface. */
public class DefaultTrainingConfig implements TrainingConfig {
- private Initializer initializer;
+ private PairList> initializers = new PairList<>();
private Optimizer optimizer;
private Device[] devices;
private Loss loss;
@@ -38,15 +38,12 @@ public class DefaultTrainingConfig implements TrainingConfig {
/**
* Creates an instance of {@code DefaultTrainingConfig} with the given {@link Loss}. {@code
- * DefaultTrainingConfig} creates a default {@link TrainingConfig} with the {@link
- * XavierInitializer} as initialiser, {@link Adam} as optimiser, and the given {@link Loss}. The
- * evaluators and listeners are left to the user's discretion.
+ * DefaultTrainingConfig} creates a default {@link TrainingConfig}, {@link Adam} as optimiser,
+ * and the given {@link Loss}. The evaluators and listeners are left to the user's discretion.
*
* @param loss the loss to use for training
*/
public DefaultTrainingConfig(Loss loss) {
- // Defaults to initializer defined in https://arxiv.org/abs/1502.01852
- this.initializer = new XavierInitializer(RandomType.GAUSSIAN, FactorType.IN, 2);
optimizer = Adam.builder().build();
this.loss = loss;
evaluators = new ArrayList<>();
@@ -58,10 +55,38 @@ public DefaultTrainingConfig(Loss loss) {
* href="https://arxiv.org/abs/1502.01852">paper).
*
* @param initializer the initialer to use for the parameters
+ * @param type the {@link Parameter.Type} of the parameters
* @return this {@code DefaultTrainingConfig}
*/
- public DefaultTrainingConfig optInitializer(Initializer initializer) {
- this.initializer = initializer;
+ public DefaultTrainingConfig optInitializer(Initializer initializer, Parameter.Type type) {
+ initializers.add(initializer, parameter -> parameter.getType().equals(type));
+ return this;
+ }
+
+ /**
+ * Sets the {@link Initializer} to use for the parameters (default from paper).
+ *
+ * @param initializer the initialer to use for the parameters
+ * @param name the name of the parameter
+ * @return this {@code DefaultTrainingConfig}
+ */
+ public DefaultTrainingConfig optInitializer(Initializer initializer, String name) {
+ initializers.add(initializer, parameter -> parameter.getName().equals(name));
+ return this;
+ }
+
+ /**
+ * Sets the {@link Initializer} to use for the parameters (default from paper).
+ *
+ * @param initializer the initialer to use for the parameters
+ * @param predicate the predicate to identify parameter
+ * @return this {@code DefaultTrainingConfig}
+ */
+ public DefaultTrainingConfig optInitializer(
+ Initializer initializer, Predicate predicate) {
+ initializers.add(initializer, predicate);
return this;
}
@@ -120,8 +145,8 @@ public Device[] getDevices() {
/** {@inheritDoc} */
@Override
- public Initializer getInitializer() {
- return initializer;
+ public PairList> getInitializers() {
+ return initializers;
}
/** {@inheritDoc} */
diff --git a/api/src/main/java/ai/djl/training/TrainingConfig.java b/api/src/main/java/ai/djl/training/TrainingConfig.java
index 46748232035..0a4b5928266 100644
--- a/api/src/main/java/ai/djl/training/TrainingConfig.java
+++ b/api/src/main/java/ai/djl/training/TrainingConfig.java
@@ -13,12 +13,15 @@
package ai.djl.training;
import ai.djl.Device;
+import ai.djl.nn.Parameter;
import ai.djl.training.evaluator.Evaluator;
import ai.djl.training.initializer.Initializer;
import ai.djl.training.listener.TrainingListener;
import ai.djl.training.loss.Loss;
import ai.djl.training.optimizer.Optimizer;
+import ai.djl.util.PairList;
import java.util.List;
+import java.util.function.Predicate;
/**
* An interface that is responsible for holding the configuration required by {@link Trainer}.
@@ -64,11 +67,11 @@ public interface TrainingConfig {
Device[] getDevices();
/**
- * Gets the {@link Initializer} to initialize the parameters of the model.
+ * Gets a list of {@link Initializer} and Predicate to initialize the parameters of the model.
*
* @return an {@link Initializer}
*/
- Initializer getInitializer();
+ PairList> getInitializers();
/**
* Gets the {@link Optimizer} to use during training.
diff --git a/api/src/main/java/ai/djl/training/initializer/XavierInitializer.java b/api/src/main/java/ai/djl/training/initializer/XavierInitializer.java
index 64ee6279f71..8cf76c7b8f0 100644
--- a/api/src/main/java/ai/djl/training/initializer/XavierInitializer.java
+++ b/api/src/main/java/ai/djl/training/initializer/XavierInitializer.java
@@ -80,7 +80,7 @@ public XavierInitializer(RandomType randomType, FactorType factorType, float mag
/** Creates a new instance of {@code XavierInitializer}. */
public XavierInitializer() {
- this(RandomType.UNIFORM, FactorType.AVG, 3f);
+ this(RandomType.UNIFORM, FactorType.AVG, 6f);
}
/** {@inheritDoc} */
diff --git a/api/src/main/java/ai/djl/training/listener/MemoryTrainingListener.java b/api/src/main/java/ai/djl/training/listener/MemoryTrainingListener.java
index 829b0d9994b..5cb6303840c 100644
--- a/api/src/main/java/ai/djl/training/listener/MemoryTrainingListener.java
+++ b/api/src/main/java/ai/djl/training/listener/MemoryTrainingListener.java
@@ -53,6 +53,10 @@ public MemoryTrainingListener() {}
/**
* Constructs a {@link MemoryTrainingListener} that outputs data in the given directory.
*
+ *
If an output directory is provided, the file "$outputDir/memory.log" will be created after
+ * training with the memory usage results. The log file consists of heap bytes, non-heap bytes,
+ * cpu percentage and rss bytes consumption along with the timestamps.
+ *
* @param outputDir the directory to output the tracked memory data in
*/
public MemoryTrainingListener(String outputDir) {
@@ -81,7 +85,9 @@ public void onTrainingEnd(Trainer trainer) {
}
/**
- * Collect memory information.
+ * Collects memory information. In order to collect metrics, the {@link Trainer} must set
+ * metrics. Monitor the metrics by enabling the following flag in the command line arguments:
+ * -Dcollect-memory=true
*
* @param metrics {@link Metrics} to store memory information
*/
diff --git a/api/src/main/java/ai/djl/training/listener/SaveModelTrainingListener.java b/api/src/main/java/ai/djl/training/listener/SaveModelTrainingListener.java
index 1ae2916aea2..0f128031e8d 100644
--- a/api/src/main/java/ai/djl/training/listener/SaveModelTrainingListener.java
+++ b/api/src/main/java/ai/djl/training/listener/SaveModelTrainingListener.java
@@ -108,7 +108,8 @@ public void setOverrideModelName(String overrideModelName) {
}
/**
- * Returns the checkpoint frequency (or -1 for no checkpointing).
+ * Returns the checkpoint frequency (or -1 for no checkpointing) in {@link
+ * SaveModelTrainingListener}.
*
* @return the checkpoint frequency (or -1 for no checkpointing)
*/
@@ -117,7 +118,7 @@ public int getCheckpoint() {
}
/**
- * Sets the checkpoint frequency.
+ * Sets the checkpoint frequency in {@link SaveModelTrainingListener}.
*
* @param checkpoint how many epochs between checkpoints (or -1 for no checkpoints)
*/
diff --git a/api/src/test/java/ai/djl/nn/convolutional/ShapeUtils.java b/api/src/test/java/ai/djl/nn/convolutional/ShapeUtils.java
index abd5e9ec2a2..562dd3a0de9 100644
--- a/api/src/test/java/ai/djl/nn/convolutional/ShapeUtils.java
+++ b/api/src/test/java/ai/djl/nn/convolutional/ShapeUtils.java
@@ -31,7 +31,7 @@ private ShapeUtils() {}
* @return the corresponding output shape for the provided input
*/
public static Shape outputShapeForBlock(NDManager manager, Block block, Shape inputShape) {
- Shape[] outputs = block.getOutputShapes(manager, new Shape[] {inputShape});
+ Shape[] outputs = block.getOutputShapes(new Shape[] {inputShape});
return outputs[0];
}
diff --git a/basicdataset/src/test/java/ai/djl/basicdataset/AirfoilRandomAccessTest.java b/basicdataset/src/test/java/ai/djl/basicdataset/AirfoilRandomAccessTest.java
index 3efdd00af77..dc8028c5c92 100644
--- a/basicdataset/src/test/java/ai/djl/basicdataset/AirfoilRandomAccessTest.java
+++ b/basicdataset/src/test/java/ai/djl/basicdataset/AirfoilRandomAccessTest.java
@@ -17,6 +17,7 @@
import ai.djl.ndarray.NDList;
import ai.djl.ndarray.NDManager;
import ai.djl.nn.Blocks;
+import ai.djl.nn.Parameter;
import ai.djl.training.DefaultTrainingConfig;
import ai.djl.training.Trainer;
import ai.djl.training.TrainingConfig;
@@ -48,7 +49,7 @@ public class AirfoilRandomAccessTest {
public void testAirfoilRemote() throws IOException, TranslateException {
TrainingConfig config =
new DefaultTrainingConfig(Loss.softmaxCrossEntropyLoss())
- .optInitializer(Initializer.ONES);
+ .optInitializer(Initializer.ONES, Parameter.Type.WEIGHT);
try (Model model = Model.newInstance("model")) {
model.setBlock(Blocks.identityBlock());
diff --git a/basicdataset/src/test/java/ai/djl/basicdataset/AmesRandomAccessTest.java b/basicdataset/src/test/java/ai/djl/basicdataset/AmesRandomAccessTest.java
index 20bf7f8ac56..716f04c578f 100644
--- a/basicdataset/src/test/java/ai/djl/basicdataset/AmesRandomAccessTest.java
+++ b/basicdataset/src/test/java/ai/djl/basicdataset/AmesRandomAccessTest.java
@@ -17,6 +17,7 @@
import ai.djl.ndarray.NDList;
import ai.djl.ndarray.NDManager;
import ai.djl.nn.Blocks;
+import ai.djl.nn.Parameter;
import ai.djl.training.DefaultTrainingConfig;
import ai.djl.training.Trainer;
import ai.djl.training.TrainingConfig;
@@ -48,7 +49,7 @@ public class AmesRandomAccessTest {
public void testAmesRandomAccessRemote() throws IOException, TranslateException {
TrainingConfig config =
new DefaultTrainingConfig(Loss.softmaxCrossEntropyLoss())
- .optInitializer(Initializer.ONES);
+ .optInitializer(Initializer.ONES, Parameter.Type.WEIGHT);
try (Model model = Model.newInstance("model")) {
model.setBlock(Blocks.identityBlock());
diff --git a/basicdataset/src/test/java/ai/djl/basicdataset/PikachuTest.java b/basicdataset/src/test/java/ai/djl/basicdataset/PikachuTest.java
index 75bcb3e914a..063ec07fb13 100644
--- a/basicdataset/src/test/java/ai/djl/basicdataset/PikachuTest.java
+++ b/basicdataset/src/test/java/ai/djl/basicdataset/PikachuTest.java
@@ -16,6 +16,7 @@
import ai.djl.basicdataset.cv.PikachuDetection;
import ai.djl.ndarray.types.Shape;
import ai.djl.nn.Blocks;
+import ai.djl.nn.Parameter;
import ai.djl.training.DefaultTrainingConfig;
import ai.djl.training.Trainer;
import ai.djl.training.TrainingConfig;
@@ -42,7 +43,7 @@ public void testPikachuRemote() throws IOException, TranslateException {
.build();
TrainingConfig config =
new DefaultTrainingConfig(Loss.softmaxCrossEntropyLoss())
- .optInitializer(new NormalInitializer(0.01f));
+ .optInitializer(new NormalInitializer(0.01f), Parameter.Type.WEIGHT);
try (Model model = Model.newInstance("model")) {
model.setBlock(Blocks.identityBlock());
try (Trainer trainer = model.newTrainer(config)) {
diff --git a/central/src/main/java/ai/djl/serving/central/HttpStaticFileServerInitializer.java b/central/src/main/java/ai/djl/serving/central/HttpStaticFileServerInitializer.java
index e54a1303280..5a7ef1fc1b8 100644
--- a/central/src/main/java/ai/djl/serving/central/HttpStaticFileServerInitializer.java
+++ b/central/src/main/java/ai/djl/serving/central/HttpStaticFileServerInitializer.java
@@ -13,6 +13,7 @@
package ai.djl.serving.central;
import ai.djl.serving.central.handler.HttpStaticFileServerHandler;
+import ai.djl.serving.central.handler.ModelDownloadHandler;
import ai.djl.serving.central.handler.ModelMetaDataHandler;
import io.netty.channel.ChannelInitializer;
import io.netty.channel.ChannelPipeline;
@@ -54,6 +55,7 @@ public void initChannel(SocketChannel ch) {
pipeline.addLast(new HttpServerCodec());
pipeline.addLast(new HttpObjectAggregator(65536));
pipeline.addLast(new ChunkedWriteHandler());
+ pipeline.addLast(new ModelDownloadHandler());
pipeline.addLast(new ModelMetaDataHandler());
pipeline.addLast(new HttpStaticFileServerHandler());
}
diff --git a/central/src/main/java/ai/djl/serving/central/handler/ModelDownloadHandler.java b/central/src/main/java/ai/djl/serving/central/handler/ModelDownloadHandler.java
new file mode 100644
index 00000000000..d6daf2944d6
--- /dev/null
+++ b/central/src/main/java/ai/djl/serving/central/handler/ModelDownloadHandler.java
@@ -0,0 +1,81 @@
+/*
+ * Copyright 2021 Amazon.com, Inc. or its affiliates. All Rights Reserved.
+ *
+ * Licensed under the Apache License, Version 2.0 (the "License"). You may not use this file except in compliance
+ * with the License. A copy of the License is located at
+ *
+ * http://aws.amazon.com/apache2.0/
+ *
+ * or in the "license" file accompanying this file. This file is distributed on an "AS IS" BASIS, WITHOUT WARRANTIES
+ * OR CONDITIONS OF ANY KIND, either express or implied. See the License for the specific language governing permissions
+ * and limitations under the License.
+ */
+package ai.djl.serving.central.handler;
+
+import ai.djl.repository.zoo.ModelNotFoundException;
+import ai.djl.serving.central.http.BadRequestException;
+import ai.djl.serving.central.responseencoder.HttpRequestResponse;
+import ai.djl.serving.central.utils.ModelUri;
+import ai.djl.serving.central.utils.NettyUtils;
+import io.netty.channel.ChannelHandlerContext;
+import io.netty.channel.SimpleChannelInboundHandler;
+import io.netty.handler.codec.http.FullHttpRequest;
+import io.netty.handler.codec.http.QueryStringDecoder;
+import java.io.IOException;
+import java.util.Collections;
+import java.util.concurrent.CompletableFuture;
+
+/**
+ * A handler to handle download requests from the ModelView.
+ *
+ * @author anfee1@morgan.edu
+ */
+public class ModelDownloadHandler extends SimpleChannelInboundHandler {
+
+ HttpRequestResponse jsonResponse;
+
+ /** Constructs a ModelDownloadHandler. */
+ public ModelDownloadHandler() {
+ jsonResponse = new HttpRequestResponse();
+ }
+
+ /**
+ * Handles the deployment request by forwarding the request to the serving-instance.
+ *
+ * @param ctx the context
+ * @param request the full request
+ */
+ @Override
+ protected void channelRead0(ChannelHandlerContext ctx, FullHttpRequest request)
+ throws IOException, ModelNotFoundException {
+ QueryStringDecoder decoder = new QueryStringDecoder(request.uri());
+ String modelName = NettyUtils.getParameter(decoder, "modelName", null);
+ String modelGroupId = NettyUtils.getParameter(decoder, "groupId", null);
+ String modelArtifactId = NettyUtils.getParameter(decoder, "artifactId", null);
+ CompletableFuture.supplyAsync(
+ () -> {
+ try {
+ if (modelName != null) {
+ return ModelUri.uriFinder(
+ modelArtifactId, modelGroupId, modelName);
+ } else {
+ throw new BadRequestException("modelName is mandatory.");
+ }
+
+ } catch (IOException | ModelNotFoundException ex) {
+ throw new IllegalArgumentException(ex.getMessage(), ex);
+ }
+ })
+ .exceptionally((ex) -> Collections.emptyMap())
+ .thenAccept(uriMap -> jsonResponse.sendAsJson(ctx, request, uriMap));
+ }
+
+ /** {@inheritDoc} */
+ @Override
+ public boolean acceptInboundMessage(Object msg) {
+ FullHttpRequest request = (FullHttpRequest) msg;
+
+ String uri = request.uri();
+ return uri.startsWith("/serving/models?");
+ }
+}
diff --git a/central/src/main/java/ai/djl/serving/central/http/BadRequestException.java b/central/src/main/java/ai/djl/serving/central/http/BadRequestException.java
new file mode 100644
index 00000000000..c4905078b63
--- /dev/null
+++ b/central/src/main/java/ai/djl/serving/central/http/BadRequestException.java
@@ -0,0 +1,40 @@
+/*
+ * Copyright 2020 Amazon.com, Inc. or its affiliates. All Rights Reserved.
+ *
+ * Licensed under the Apache License, Version 2.0 (the "License"). You may not use this file except in compliance
+ * with the License. A copy of the License is located at
+ *
+ * http://aws.amazon.com/apache2.0/
+ *
+ * or in the "license" file accompanying this file. This file is distributed on an "AS IS" BASIS, WITHOUT WARRANTIES
+ * OR CONDITIONS OF ANY KIND, either express or implied. See the License for the specific language governing permissions
+ * and limitations under the License.
+ */
+package ai.djl.serving.central.http;
+
+/** Thrown when a bad HTTP request is received. */
+public class BadRequestException extends IllegalArgumentException {
+
+ static final long serialVersionUID = 1L;
+
+ /**
+ * Constructs an {@code BadRequestException} with the specified detail message.
+ *
+ * @param message The detail message (which is saved for later retrieval by the {@link
+ * #getMessage()} method)
+ */
+ public BadRequestException(String message) {
+ super(message);
+ }
+
+ /**
+ * Constructs an {@code BadRequestException} with the specified detail message and a root cause.
+ *
+ * @param message The detail message (which is saved for later retrieval by the {@link
+ * #getMessage()} method)
+ * @param cause root cause
+ */
+ public BadRequestException(String message, Throwable cause) {
+ super(message, cause);
+ }
+}
diff --git a/central/src/main/java/ai/djl/serving/central/http/package-info.java b/central/src/main/java/ai/djl/serving/central/http/package-info.java
new file mode 100644
index 00000000000..fb26e5c6c82
--- /dev/null
+++ b/central/src/main/java/ai/djl/serving/central/http/package-info.java
@@ -0,0 +1,14 @@
+/*
+ * Copyright 2021 Amazon.com, Inc. or its affiliates. All Rights Reserved.
+ *
+ * Licensed under the Apache License, Version 2.0 (the "License"). You may not use this file except in compliance
+ * with the License. A copy of the License is located at
+ *
+ * http://aws.amazon.com/apache2.0/
+ *
+ * or in the "license" file accompanying this file. This file is distributed on an "AS IS" BASIS, WITHOUT WARRANTIES
+ * OR CONDITIONS OF ANY KIND, either express or implied. See the License for the specific language governing permissions
+ * and limitations under the License.
+ */
+/** Contains HTTP codes. */
+package ai.djl.serving.central.http;
diff --git a/central/src/main/java/ai/djl/serving/central/responseencoder/HttpRequestResponse.java b/central/src/main/java/ai/djl/serving/central/responseencoder/HttpRequestResponse.java
new file mode 100644
index 00000000000..59e97dffdfd
--- /dev/null
+++ b/central/src/main/java/ai/djl/serving/central/responseencoder/HttpRequestResponse.java
@@ -0,0 +1,123 @@
+/*
+ * Copyright 2021 Amazon.com, Inc. or its affiliates. All Rights Reserved.
+ *
+ * Licensed under the Apache License, Version 2.0 (the "License"). You may not use this file except in compliance
+ * with the License. A copy of the License is located at
+ *
+ * http://aws.amazon.com/apache2.0/
+ *
+ * or in the "license" file accompanying this file. This file is distributed on an "AS IS" BASIS, WITHOUT WARRANTIES
+ * OR CONDITIONS OF ANY KIND, either express or implied. See the License for the specific language governing permissions
+ * and limitations under the License.
+ */
+package ai.djl.serving.central.responseencoder;
+
+import ai.djl.modality.Classifications;
+import ai.djl.modality.Classifications.ClassificationsSerializer;
+import ai.djl.modality.cv.output.DetectedObjects;
+import ai.djl.repository.Metadata;
+import com.google.gson.Gson;
+import com.google.gson.GsonBuilder;
+import com.google.gson.JsonPrimitive;
+import com.google.gson.JsonSerializer;
+import io.netty.buffer.ByteBuf;
+import io.netty.channel.ChannelFuture;
+import io.netty.channel.ChannelFutureListener;
+import io.netty.channel.ChannelHandlerContext;
+import io.netty.handler.codec.http.DefaultFullHttpResponse;
+import io.netty.handler.codec.http.FullHttpRequest;
+import io.netty.handler.codec.http.FullHttpResponse;
+import io.netty.handler.codec.http.HttpHeaderNames;
+import io.netty.handler.codec.http.HttpHeaderValues;
+import io.netty.handler.codec.http.HttpResponseStatus;
+import io.netty.handler.codec.http.HttpUtil;
+import io.netty.handler.codec.http.HttpVersion;
+import io.netty.util.CharsetUtil;
+import java.lang.reflect.Modifier;
+
+/**
+ * Serialize to json and send the response to the client.
+ *
+ * @author erik.bamberg@web.de
+ */
+public class HttpRequestResponse {
+
+ private static final Gson GSON_WITH_TRANSIENT_FIELDS =
+ new GsonBuilder()
+ .setDateFormat("yyyy-MM-dd'T'HH:mm:ss.SSS'Z'")
+ .setPrettyPrinting()
+ .excludeFieldsWithModifiers(Modifier.STATIC)
+ .registerTypeAdapter(Classifications.class, new ClassificationsSerializer())
+ .registerTypeAdapter(DetectedObjects.class, new ClassificationsSerializer())
+ .registerTypeAdapter(Metadata.class, new MetaDataSerializer())
+ .registerTypeAdapter(
+ Double.class,
+ (JsonSerializer)
+ (src, t, ctx) -> {
+ long v = src.longValue();
+ if (src.equals(Double.valueOf(String.valueOf(v)))) {
+ return new JsonPrimitive(v);
+ }
+ return new JsonPrimitive(src);
+ })
+ .create();
+
+ /**
+ * send a response to the client.
+ *
+ * @param ctx channel context
+ * @param request full request
+ * @param entity the response
+ */
+ public void sendAsJson(ChannelHandlerContext ctx, FullHttpRequest request, Object entity) {
+
+ String serialized = GSON_WITH_TRANSIENT_FIELDS.toJson(entity);
+ ByteBuf buffer = ctx.alloc().buffer(serialized.length());
+ buffer.writeCharSequence(serialized, CharsetUtil.UTF_8);
+
+ FullHttpResponse response =
+ new DefaultFullHttpResponse(HttpVersion.HTTP_1_1, HttpResponseStatus.OK, buffer);
+ response.headers().set(HttpHeaderNames.CONTENT_TYPE, "application/json; charset=UTF-8");
+ boolean keepAlive = HttpUtil.isKeepAlive(request);
+ this.sendAndCleanupConnection(ctx, response, keepAlive);
+ }
+
+ /**
+ * send content of a ByteBuffer as response to the client.
+ *
+ * @param ctx channel context
+ * @param buffer response buffer
+ */
+ public void sendByteBuffer(ChannelHandlerContext ctx, ByteBuf buffer) {
+
+ FullHttpResponse response =
+ new DefaultFullHttpResponse(HttpVersion.HTTP_1_1, HttpResponseStatus.OK, buffer);
+ response.headers().set(HttpHeaderNames.CONTENT_TYPE, "application/json; charset=UTF-8");
+ this.sendAndCleanupConnection(ctx, response, false);
+ }
+
+ /**
+ * If Keep-Alive is disabled, attaches "Connection: close" header to the response and closes the
+ * connection after the response being sent.
+ *
+ * @param ctx context
+ * @param response full response
+ * @param keepAlive is alive or not
+ */
+ private void sendAndCleanupConnection(
+ ChannelHandlerContext ctx, FullHttpResponse response, boolean keepAlive) {
+ HttpUtil.setContentLength(response, response.content().readableBytes());
+ if (!keepAlive) {
+ // We're going to close the connection as soon as the response is sent,
+ // so we should also make it clear for the client.
+ response.headers().set(HttpHeaderNames.CONNECTION, HttpHeaderValues.CLOSE);
+ }
+
+ ChannelFuture flushPromise = ctx.writeAndFlush(response);
+
+ if (!keepAlive) {
+ // Close the connection as soon as the response is sent.
+ flushPromise.addListener(ChannelFutureListener.CLOSE);
+ }
+ }
+}
diff --git a/central/src/main/java/ai/djl/serving/central/utils/ModelUri.java b/central/src/main/java/ai/djl/serving/central/utils/ModelUri.java
new file mode 100644
index 00000000000..b1bc9a6a691
--- /dev/null
+++ b/central/src/main/java/ai/djl/serving/central/utils/ModelUri.java
@@ -0,0 +1,71 @@
+/*
+ * Copyright 2021 Amazon.com, Inc. or its affiliates. All Rights Reserved.
+ *
+ * Licensed under the Apache License, Version 2.0 (the "License"). You may not use this file except in compliance
+ * with the License. A copy of the License is located at
+ *
+ * http://aws.amazon.com/apache2.0/
+ *
+ * or in the "license" file accompanying this file. This file is distributed on an "AS IS" BASIS, WITHOUT WARRANTIES
+ * OR CONDITIONS OF ANY KIND, either express or implied. See the License for the specific language governing permissions
+ * and limitations under the License.
+ */
+package ai.djl.serving.central.utils;
+
+import ai.djl.Application;
+import ai.djl.repository.Artifact;
+import ai.djl.repository.zoo.Criteria;
+import ai.djl.repository.zoo.ModelNotFoundException;
+import ai.djl.repository.zoo.ModelZoo;
+import java.io.IOException;
+import java.net.URI;
+import java.util.List;
+import java.util.Map;
+import java.util.concurrent.ConcurrentHashMap;
+
+/** A class to find the URIs when given a model name. */
+public final class ModelUri {
+
+ // TODO: Use the artifact repository to create base URI
+ private static URI base = URI.create("https://mlrepo.djl.ai/");
+
+ private ModelUri() {}
+
+ /**
+ * Takes in a model name, artifactId, and groupId to return a Map of download URIs.
+ *
+ * @param artifactId is the artifactId of the model
+ * @param groupId is the groupId of the model
+ * @param name is the name of the model
+ * @return a map of download URIs
+ * @throws IOException if the uri could not be found
+ * @throws ModelNotFoundException if Model can not be found
+ */
+ public static Map uriFinder(String artifactId, String groupId, String name)
+ throws IOException, ModelNotFoundException {
+ Criteria, ?> criteria =
+ Criteria.builder()
+ .optModelName(name)
+ .optGroupId(groupId)
+ .optArtifactId(artifactId)
+ .build();
+ Map> models = ModelZoo.listModels(criteria);
+ Map uris = new ConcurrentHashMap<>();
+ models.forEach(
+ (app, list) -> {
+ list.forEach(
+ artifact -> {
+ for (Map.Entry entry :
+ artifact.getFiles().entrySet()) {
+ URI fileUri = URI.create(entry.getValue().getUri());
+ URI baseUri = artifact.getMetadata().getRepositoryUri();
+ if (!fileUri.isAbsolute()) {
+ fileUri = base.resolve(baseUri).resolve(fileUri);
+ }
+ uris.put(entry.getKey(), fileUri);
+ }
+ });
+ });
+ return uris;
+ }
+}
diff --git a/central/src/main/java/ai/djl/serving/central/utils/NettyUtils.java b/central/src/main/java/ai/djl/serving/central/utils/NettyUtils.java
new file mode 100644
index 00000000000..ce0e3623cf5
--- /dev/null
+++ b/central/src/main/java/ai/djl/serving/central/utils/NettyUtils.java
@@ -0,0 +1,109 @@
+/*
+ * Copyright 2020 Amazon.com, Inc. or its affiliates. All Rights Reserved.
+ *
+ * Licensed under the Apache License, Version 2.0 (the "License"). You may not use this file except in compliance
+ * with the License. A copy of the License is located at
+ *
+ * http://aws.amazon.com/apache2.0/
+ *
+ * or in the "license" file accompanying this file. This file is distributed on an "AS IS" BASIS, WITHOUT WARRANTIES
+ * OR CONDITIONS OF ANY KIND, either express or implied. See the License for the specific language governing permissions
+ * and limitations under the License.
+ */
+package ai.djl.serving.central.utils;
+
+import ai.djl.modality.Input;
+import io.netty.buffer.ByteBuf;
+import io.netty.handler.codec.http.QueryStringDecoder;
+import io.netty.handler.codec.http.multipart.Attribute;
+import io.netty.handler.codec.http.multipart.FileUpload;
+import io.netty.handler.codec.http.multipart.InterfaceHttpData;
+import java.io.IOException;
+import java.nio.charset.StandardCharsets;
+import java.util.List;
+
+/** A utility class that handling Netty request and response. */
+public final class NettyUtils {
+
+ private NettyUtils() {}
+
+ /**
+ * Returns the bytes for the specified {@code ByteBuf}.
+ *
+ * @param buf the {@code ByteBuf} to read
+ * @return the bytes for the specified {@code ByteBuf}
+ */
+ public static byte[] getBytes(ByteBuf buf) {
+ if (buf.hasArray()) {
+ return buf.array();
+ }
+
+ byte[] ret = new byte[buf.readableBytes()];
+ int readerIndex = buf.readerIndex();
+ buf.getBytes(readerIndex, ret);
+ return ret;
+ }
+
+ /**
+ * Reads the parameter's value for the key from the uri.
+ *
+ * @param decoder the {@code QueryStringDecoder} parsed from uri
+ * @param key the parameter key
+ * @param def the default value
+ * @return the parameter's value
+ */
+ public static String getParameter(QueryStringDecoder decoder, String key, String def) {
+ List param = decoder.parameters().get(key);
+ if (param != null && !param.isEmpty()) {
+ return param.get(0);
+ }
+ return def;
+ }
+
+ /**
+ * Read the parameter's integer value for the key from the uri.
+ *
+ * @param decoder the {@code QueryStringDecoder} parsed from uri
+ * @param key the parameter key
+ * @param def the default value
+ * @return the parameter's integer value
+ * @throws NumberFormatException exception is thrown when the parameter-value is not numeric.
+ */
+ public static int getIntParameter(QueryStringDecoder decoder, String key, int def) {
+ String value = getParameter(decoder, key, null);
+ if (value == null || value.isEmpty()) {
+ return def;
+ }
+ return Integer.parseInt(value);
+ }
+
+ /**
+ * Parses form data and added to the {@link Input} object.
+ *
+ * @param data the form data
+ * @param input the {@link Input} object to be added to
+ */
+ public static void addFormData(InterfaceHttpData data, Input input) {
+ if (data == null) {
+ return;
+ }
+ try {
+ String name = data.getName();
+ switch (data.getHttpDataType()) {
+ case Attribute:
+ Attribute attribute = (Attribute) data;
+ input.addData(name, attribute.getValue().getBytes(StandardCharsets.UTF_8));
+ break;
+ case FileUpload:
+ FileUpload fileUpload = (FileUpload) data;
+ input.addData(name, getBytes(fileUpload.getByteBuf()));
+ break;
+ default:
+ throw new IllegalArgumentException(
+ "Except form field, but got " + data.getHttpDataType());
+ }
+ } catch (IOException e) {
+ throw new AssertionError(e);
+ }
+ }
+}
diff --git a/central/src/main/java/ai/djl/serving/central/utils/package-info.java b/central/src/main/java/ai/djl/serving/central/utils/package-info.java
new file mode 100644
index 00000000000..8bee987b03f
--- /dev/null
+++ b/central/src/main/java/ai/djl/serving/central/utils/package-info.java
@@ -0,0 +1,14 @@
+/*
+ * Copyright 2021 Amazon.com, Inc. or its affiliates. All Rights Reserved.
+ *
+ * Licensed under the Apache License, Version 2.0 (the "License"). You may not use this file except in compliance
+ * with the License. A copy of the License is located at
+ *
+ * http://aws.amazon.com/apache2.0/
+ *
+ * or in the "license" file accompanying this file. This file is distributed on an "AS IS" BASIS, WITHOUT WARRANTIES
+ * OR CONDITIONS OF ANY KIND, either express or implied. See the License for the specific language governing permissions
+ * and limitations under the License.
+ */
+/** Contains utility classes that hand response and requests. */
+package ai.djl.serving.central.utils;
diff --git a/central/src/main/webapp/components/DownloadButtons.jsx b/central/src/main/webapp/components/DownloadButtons.jsx
new file mode 100644
index 00000000000..97374cf0e5d
--- /dev/null
+++ b/central/src/main/webapp/components/DownloadButtons.jsx
@@ -0,0 +1,46 @@
+import React, { Component, useState, useEffect, useRef } from "react";
+import Button from '@material-ui/core/Button';
+import ReactDOM from 'react-dom';
+
+import { makeStyles } from '@material-ui/core/styles';
+import axios from 'axios'
+
+
+const useFetch = (model) => {
+ const [data, setData] = useState([]);
+
+ useEffect(() => {
+ async function fetchData() {
+
+ axios.get("http://"+window.location.host+"/serving/models?modelName="+model.name+"&artifactId="+model.metadata.artifactId+"&groupId="+model.metadata.groupId)
+ .then(function(response) {
+ let appdata = Object.keys(response.data).map(function(key) {
+ return {
+ key: key,
+ link: response.data[key]
+ };
+ });
+ setData(appdata);
+ console.log(appdata)
+ })
+ }
+ fetchData();
+ }, [model.modelName,model.metadata.artifactId,model.metadata.groupId]);
+
+ return data;
+};
+
+
+
+export default function ModelDownloadButtons(props) {
+ const modelUris = useFetch(props.model);
+ return (
+ <>
+ {Object.keys(modelUris).map((keys) => (
+
+
+ )
+ )}
+ >
+ );
+}
diff --git a/central/src/main/webapp/components/ModelView.jsx b/central/src/main/webapp/components/ModelView.jsx
index 3cfeb6d0f51..f5db8853ae9 100644
--- a/central/src/main/webapp/components/ModelView.jsx
+++ b/central/src/main/webapp/components/ModelView.jsx
@@ -19,6 +19,7 @@ import Chip from '@material-ui/core/Chip';
import Divider from '@material-ui/core/Divider';
import DynForm from './DynForm';
+import ModelDownloadButtons from './DownloadButtons';
import axios from 'axios'
@@ -186,6 +187,7 @@ export default function ModelView(props) {
+
@@ -250,6 +252,9 @@ export default function ModelView(props) {
:
}
+
+
+
diff --git a/dlr/dlr-engine/src/main/java/ai/djl/dlr/engine/DlrEngine.java b/dlr/dlr-engine/src/main/java/ai/djl/dlr/engine/DlrEngine.java
index 7a05b901a2d..f0975ff8296 100644
--- a/dlr/dlr-engine/src/main/java/ai/djl/dlr/engine/DlrEngine.java
+++ b/dlr/dlr-engine/src/main/java/ai/djl/dlr/engine/DlrEngine.java
@@ -19,6 +19,7 @@
import ai.djl.engine.Engine;
import ai.djl.engine.EngineException;
import ai.djl.ndarray.NDManager;
+import ai.djl.nn.SymbolBlock;
import ai.djl.training.GradientCollector;
/**
@@ -46,6 +47,9 @@ static Engine newInstance() {
}
private Engine getAlternativeEngine() {
+ if (Boolean.getBoolean("ai.djl.dlr.disable_alternative")) {
+ return null;
+ }
if (alternativeEngine == null) {
Engine engine = Engine.getInstance();
if (engine.getRank() < getRank()) {
@@ -80,6 +84,12 @@ public boolean hasCapability(String capability) {
return false;
}
+ /** {@inheritDoc} */
+ @Override
+ public SymbolBlock newSymbolBlock(NDManager manager) {
+ throw new UnsupportedOperationException("DLR does not support empty SymbolBlock");
+ }
+
/** {@inheritDoc} */
@Override
public Model newModel(String name, Device device) {
diff --git a/dlr/dlr-engine/src/main/java/ai/djl/dlr/engine/DlrNDArray.java b/dlr/dlr-engine/src/main/java/ai/djl/dlr/engine/DlrNDArray.java
index 8cb8fa653cc..59ab1683913 100644
--- a/dlr/dlr-engine/src/main/java/ai/djl/dlr/engine/DlrNDArray.java
+++ b/dlr/dlr-engine/src/main/java/ai/djl/dlr/engine/DlrNDArray.java
@@ -45,7 +45,7 @@ public class DlrNDArray implements NDArrayAdapter {
this.data = data;
this.shape = shape;
uid = UUID.randomUUID().toString();
- manager.attach(uid, this);
+ manager.attachInternal(uid, this);
}
/** {@inheritDoc} */
@@ -94,31 +94,26 @@ public Shape getShape() {
/** {@inheritDoc} */
@Override
- public NDManager attach(NDManager manager) {
+ public void attach(NDManager manager) {
detach();
- NDManager original = this.manager;
this.manager = (DlrNDManager) manager;
- manager.attach(getUid(), this);
- return original;
- }
-
- /** {@inheritDoc} */
- @Override
- public void detach() {
- manager.detach(getUid());
- manager = DlrNDManager.getSystemManager();
+ manager.attachInternal(getUid(), this);
}
/** {@inheritDoc} */
@Override
- public boolean hasGradient() {
- return false;
+ public void tempAttach(NDManager manager) {
+ detach();
+ NDManager original = this.manager;
+ this.manager = (DlrNDManager) manager;
+ manager.tempAttachInternal(original, getUid(), this);
}
/** {@inheritDoc} */
@Override
- public NDArray stopGradient() {
- throw new UnsupportedOperationException("Not supported for DLR");
+ public void detach() {
+ manager.detachInternal(getUid());
+ manager = DlrNDManager.getSystemManager();
}
/** {@inheritDoc} */
diff --git a/dlr/dlr-engine/src/main/java/ai/djl/dlr/engine/DlrNDManager.java b/dlr/dlr-engine/src/main/java/ai/djl/dlr/engine/DlrNDManager.java
index f879c907cc3..ec87ba6675d 100644
--- a/dlr/dlr-engine/src/main/java/ai/djl/dlr/engine/DlrNDManager.java
+++ b/dlr/dlr-engine/src/main/java/ai/djl/dlr/engine/DlrNDManager.java
@@ -54,7 +54,7 @@ public ByteBuffer allocateDirect(int capacity) {
@Override
public DlrNDManager newSubManager(Device dev) {
DlrNDManager manager = new DlrNDManager(this, dev);
- attach(manager.uid, manager);
+ attachInternal(manager.uid, manager);
return manager;
}
@@ -105,11 +105,11 @@ private static final class SystemManager extends DlrNDManager {
/** {@inheritDoc} */
@Override
- public void attach(String resourceId, AutoCloseable resource) {}
+ public void attachInternal(String resourceId, AutoCloseable resource) {}
/** {@inheritDoc} */
@Override
- public void detach(String resourceId) {}
+ public void detachInternal(String resourceId) {}
/** {@inheritDoc} */
@Override
diff --git a/docs/development/profiler.md b/docs/development/profiler.md
new file mode 100644
index 00000000000..3a02ae8f0e5
--- /dev/null
+++ b/docs/development/profiler.md
@@ -0,0 +1,62 @@
+## Profiler (Experimental)
+
+Currently, DJL supports experimental profilers for developers that
+investigate the performance of operator execution as well as memory consumption.
+The profilers are from engines directly and DJL just expose them.
+So different engines have different APIs and produce different output format.
+We are still working in progress on the feature.
+In the future, we are considering to design a unified APIs and output unified format.
+
+### MXNet
+
+By setting the following environment variable, it generates `profile.json` after executing the code.
+
+```
+export MXNET_PROFILER_AUTOSTART=1
+```
+
+You can view it in a browser using trace consumer like `chrome://tracing `. Here is a snapshot that shows the sample output.
+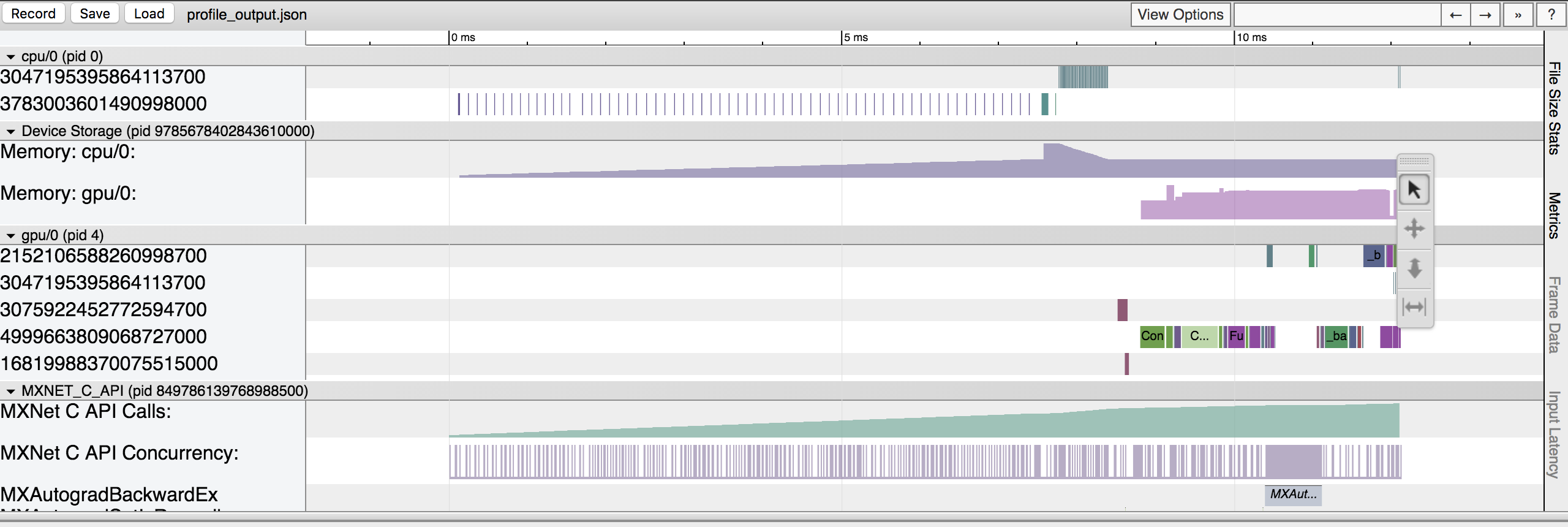
+
+### PyTorch
+
+DJL have integrated PyTorch C++ profiler API and expose `JniUtils.startProfile` and `JniUtils.stopProfile(outputFile)` Java APIs.
+`JniUtils.startProfile` takes `useCuda(boolean)`, `recordShape(boolean)` and `profileMemory(boolean)` arguments respectively.
+`useCuda` indicates if profiler enables timing of CUDA events using the cudaEvent API.
+`recordShape` indicates if information about input dimensions will be collected or not.
+`profileMemory` indicates if profiler report memory usage or not.
+`JniUtils.stopProfile` takes a outputFile of String type.
+
+Wrap the code snippet you want to profile in between `JniUtils.startProfile` and `JniUtils.stopProfile`.
+Here is an example.
+
+```
+try (ZooModel model = ModelZoo.loadModel(criteria)) {
+ try (Predictor predictor = model.newPredictor()) {
+ Image image = ImageFactory.getInstance()
+ .fromNDArray(manager.zeros(new Shape(3, 224, 224), DataType.UINT8));
+
+ JniUtils.startProfile(false, true, true);
+ predictor.predict(image);
+ JniUtils.stopProfile(outputFile);
+ } catch (TranslateException e) {
+ e.printStackTrace();
+}
+```
+
+The output format is composed of operator execution record.
+Each record contains `name`(operator name), `dur`(time duration), `shape`(input shapes), `cpu mem`(cpu memory footprint).
+
+```
+{
+ "name": "aten::empty",
+ "ph": "X",
+ "ts": 24528.313000,
+ "dur": 5.246000,
+ "tid": 1,
+ "pid": "CPU Functions",
+ "shape": [[], [], [], [], [], []],
+ "cpu mem": "0 b",
+ "args": {}
+}
+```
diff --git a/docs/mkdocs.yml b/docs/mkdocs.yml
index 5699539d81a..92b01552145 100644
--- a/docs/mkdocs.yml
+++ b/docs/mkdocs.yml
@@ -76,6 +76,7 @@ nav:
- 'docs/development/memory_management.md'
- 'docs/development/inference_performance_optimization.md'
- 'docs/development/benchmark_with_djl.md'
+ - 'docs/development/profiler.md'
- DJL Community:
- 'docs/forums.md'
- 'leaders.md'
@@ -83,6 +84,7 @@ nav:
- Overview: 'mxnet/README.md'
- Import Gluon Model: 'docs/mxnet/how_to_convert_your_model_to_symbol.md'
- Load a MXNet Model: 'jupyter/load_mxnet_model.ipynb'
+ - Backend Optimizer for MXNet: 'docs/mxnet/mxnet_backend_optimizer.md'
- Modules:
- MXNet Engine: 'mxnet/mxnet-engine/README.md'
- MXNet Model Zoo: 'mxnet/mxnet-model-zoo/README.md'
@@ -109,7 +111,12 @@ nav:
- TensorFlow Model Zoo: 'tensorflow/tensorflow-model-zoo/README.md'
- PaddlePaddle:
- Overview: 'paddlepaddle/README.md'
- - Load a PaddlePaddle Model: 'jupyter/paddlepaddle/face_mask_detection_paddlepaddle.ipynb'
+ - Facemask detection using PaddlePaddle:
+ - English: 'jupyter/paddlepaddle/face_mask_detection_paddlepaddle.ipynb'
+ - 中文: 'jupyter/paddlepaddle/face_mask_detection_paddlepaddle_zh.ipynb'
+ - PaddleOCR example:
+ - English: 'jupyter/paddlepaddle/paddle_ocr_java.ipynb'
+ - 中文: 'jupyter/paddlepaddle/paddle_ocr_java_zh.ipynb'
- Modules:
- PaddlePaddle Engine: 'paddlepaddle/paddlepaddle-engine/README.md'
- PaddlePaddle Model Zoo: 'paddlepaddle/paddlepaddle-model-zoo/README.md'
diff --git a/docs/mxnet/mxnet_backend_optimizer.md b/docs/mxnet/mxnet_backend_optimizer.md
new file mode 100644
index 00000000000..e6e80423fbf
--- /dev/null
+++ b/docs/mxnet/mxnet_backend_optimizer.md
@@ -0,0 +1,33 @@
+# Custom backend optimizer support on Apache MXNet
+
+Apache MXNet currently implemented a method that allowing third-party
+backend optimizer to accelerate the inference result. DJL currently
+also exposed this functionality through the `MxOptimizeFor` option
+of the Criteria.
+
+```
+.optOption("MxOptimizeFor", "optimizer_name")
+```
+
+After a name is passed, DJL will try to find the party library from
+ the environment variable called `MXNET_EXTRA_LIBRARY_PATH`. Users are required to
+set this environment variable to locate the library. After that, you should see the messages from the inference to see if the library is enabled.
+
+Here is a list of supporting backend optimizers:
+
+## AWS [Elastic Inference Accelerator](https://docs.aws.amazon.com/elastic-inference/latest/developerguide/what-is-ei.html) (EIA)
+
+Currently, you can use EIA library for DJL on all EI enabled instance.
+
+You can follow the instruction to start your EI application with DJL:
+
+```
+> https://docs.aws.amazon.com/elastic-inference/latest/developerguide/ei-mxnet.html
+```
+
+Currently, the EI logging is disabled. For debugging purpose, you can enable that through
+setting the `MXNET_EXTRA_LIBRARY_VERBOSE` environment variable:
+
+```
+export MXNET_EXTRA_LIBRARY_VERBOSE=true
+```
diff --git a/examples/src/main/java/ai/djl/examples/training/TrainBertOnCode.java b/examples/src/main/java/ai/djl/examples/training/TrainBertOnCode.java
index 53eca2e14b4..7d1cda2adc2 100644
--- a/examples/src/main/java/ai/djl/examples/training/TrainBertOnCode.java
+++ b/examples/src/main/java/ai/djl/examples/training/TrainBertOnCode.java
@@ -20,6 +20,7 @@
import ai.djl.ndarray.NDList;
import ai.djl.ndarray.NDManager;
import ai.djl.ndarray.types.Shape;
+import ai.djl.nn.Parameter;
import ai.djl.nn.transformer.BertBlock;
import ai.djl.nn.transformer.BertPretrainingBlock;
import ai.djl.nn.transformer.BertPretrainingLoss;
@@ -135,7 +136,8 @@ private static Model createBertPretrainingModel(Dictionary dictionary) {
model.setBlock(
new BertPretrainingBlock(
BERT_BUILDER.setTokenDictionarySize(dictionary.tokens.size())));
- model.getBlock().setInitializer(new TruncatedNormalInitializer(0.02f));
+ model.getBlock()
+ .setInitializer(new TruncatedNormalInitializer(0.02f), Parameter.Type.WEIGHT);
return model;
}
diff --git a/examples/src/main/java/ai/djl/examples/training/TrainMnistWithLSTM.java b/examples/src/main/java/ai/djl/examples/training/TrainMnistWithLSTM.java
index 13ce0f2f742..76078106a22 100644
--- a/examples/src/main/java/ai/djl/examples/training/TrainMnistWithLSTM.java
+++ b/examples/src/main/java/ai/djl/examples/training/TrainMnistWithLSTM.java
@@ -31,7 +31,6 @@
import ai.djl.training.dataset.Dataset;
import ai.djl.training.dataset.RandomAccessDataset;
import ai.djl.training.evaluator.Accuracy;
-import ai.djl.training.initializer.XavierInitializer;
import ai.djl.training.listener.SaveModelTrainingListener;
import ai.djl.training.listener.TrainingListener;
import ai.djl.training.loss.Loss;
@@ -119,7 +118,6 @@ public static DefaultTrainingConfig setupTrainingConfig(Arguments arguments) {
return new DefaultTrainingConfig(Loss.softmaxCrossEntropyLoss())
.addEvaluator(new Accuracy())
- .optInitializer(new XavierInitializer())
.optDevices(Device.getDevices(arguments.getMaxGpus()))
.addTrainingListeners(TrainingListener.Defaults.logging(outputDir))
.addTrainingListeners(listener);
diff --git a/extensions/fasttext/src/main/java/ai/djl/fasttext/FtTrainingConfig.java b/extensions/fasttext/src/main/java/ai/djl/fasttext/FtTrainingConfig.java
index b1631b6b73f..b68fc81e1c7 100644
--- a/extensions/fasttext/src/main/java/ai/djl/fasttext/FtTrainingConfig.java
+++ b/extensions/fasttext/src/main/java/ai/djl/fasttext/FtTrainingConfig.java
@@ -13,15 +13,18 @@
package ai.djl.fasttext;
import ai.djl.Device;
+import ai.djl.nn.Parameter;
import ai.djl.training.TrainingConfig;
import ai.djl.training.evaluator.Evaluator;
import ai.djl.training.initializer.Initializer;
import ai.djl.training.listener.TrainingListener;
import ai.djl.training.loss.Loss;
import ai.djl.training.optimizer.Optimizer;
+import ai.djl.util.PairList;
import java.nio.file.Path;
import java.util.ArrayList;
import java.util.List;
+import java.util.function.Predicate;
/** An interface that is responsible for holding the configuration required by fastText training. */
public class FtTrainingConfig implements TrainingConfig {
@@ -247,7 +250,7 @@ public Device[] getDevices() {
/** {@inheritDoc} */
@Override
- public Initializer getInitializer() {
+ public PairList> getInitializers() {
return null;
}
diff --git a/gradle.properties b/gradle.properties
index cbc4bbf41c5..7b7b13cab0d 100644
--- a/gradle.properties
+++ b/gradle.properties
@@ -13,7 +13,7 @@ pytorch_version=1.7.1
tensorflow_version=2.3.1
tflite_version=2.4.1
dlr_version=1.6.0
-onnxruntime_version=1.5.2
+onnxruntime_version=1.7.0
paddlepaddle_version=2.0.0
sentencepiece_version=0.1.92
fasttext_version=0.9.2
diff --git a/integration/src/main/java/ai/djl/integration/tests/modality/cv/SingleShotDetectionTest.java b/integration/src/main/java/ai/djl/integration/tests/modality/cv/SingleShotDetectionTest.java
index f105d46c8cd..2f6e41cda36 100644
--- a/integration/src/main/java/ai/djl/integration/tests/modality/cv/SingleShotDetectionTest.java
+++ b/integration/src/main/java/ai/djl/integration/tests/modality/cv/SingleShotDetectionTest.java
@@ -20,7 +20,6 @@
import ai.djl.nn.Block;
import ai.djl.nn.SequentialBlock;
import ai.djl.training.ParameterStore;
-import ai.djl.training.initializer.XavierInitializer;
import java.util.ArrayList;
import java.util.Arrays;
import java.util.List;
@@ -31,41 +30,34 @@ public class SingleShotDetectionTest {
@Test
public void testClassPredictorBlocks() {
- try (NDManager manager = NDManager.newBaseManager()) {
- Block block = SingleShotDetection.getClassPredictionBlock(5, 10);
- Assert.assertEquals(
- block.getOutputShapes(manager, new Shape[] {new Shape(2, 8, 20, 20)})[0],
- new Shape(2, 55, 20, 20));
- block = SingleShotDetection.getClassPredictionBlock(3, 10);
- Assert.assertEquals(
- block.getOutputShapes(manager, new Shape[] {new Shape(2, 16, 10, 10)})[0],
- new Shape(2, 33, 10, 10));
- }
+ Block block = SingleShotDetection.getClassPredictionBlock(5, 10);
+ Assert.assertEquals(
+ block.getOutputShapes(new Shape[] {new Shape(2, 8, 20, 20)})[0],
+ new Shape(2, 55, 20, 20));
+ block = SingleShotDetection.getClassPredictionBlock(3, 10);
+ Assert.assertEquals(
+ block.getOutputShapes(new Shape[] {new Shape(2, 16, 10, 10)})[0],
+ new Shape(2, 33, 10, 10));
}
@Test
public void testAnchorPredictorBlocks() {
- try (NDManager manager = NDManager.newBaseManager()) {
- Block block = SingleShotDetection.getAnchorPredictionBlock(5);
- Assert.assertEquals(
- block.getOutputShapes(manager, new Shape[] {new Shape(2, 8, 20, 20)})[0],
- new Shape(2, 20, 20, 20));
- block = SingleShotDetection.getClassPredictionBlock(3, 10);
- Assert.assertEquals(
- block.getOutputShapes(manager, new Shape[] {new Shape(2, 16, 10, 10)})[0],
- new Shape(2, 33, 10, 10));
- }
+ Block block = SingleShotDetection.getAnchorPredictionBlock(5);
+ Assert.assertEquals(
+ block.getOutputShapes(new Shape[] {new Shape(2, 8, 20, 20)})[0],
+ new Shape(2, 20, 20, 20));
+ block = SingleShotDetection.getClassPredictionBlock(3, 10);
+ Assert.assertEquals(
+ block.getOutputShapes(new Shape[] {new Shape(2, 16, 10, 10)})[0],
+ new Shape(2, 33, 10, 10));
}
@Test
public void testDownSamplingBlock() {
- try (NDManager manager = NDManager.newBaseManager()) {
- Block sequentialBlock = SingleShotDetection.getDownSamplingBlock(10);
- Assert.assertEquals(
- sequentialBlock
- .getOutputShapes(manager, new Shape[] {new Shape(2, 3, 20, 20)})[0],
- new Shape(2, 10, 10, 10));
- }
+ Block sequentialBlock = SingleShotDetection.getDownSamplingBlock(10);
+ Assert.assertEquals(
+ sequentialBlock.getOutputShapes(new Shape[] {new Shape(2, 3, 20, 20)})[0],
+ new Shape(2, 10, 10, 10));
}
@Test
@@ -97,7 +89,6 @@ public void testSingleShotDetectionShape() {
.setSizes(sizes)
.setBaseNetwork(block)
.build();
- ssd.setInitializer(new XavierInitializer());
ssd.initialize(manager, DataType.FLOAT32, new Shape(32, 3, 256, 256));
ParameterStore ps = new ParameterStore(manager, false);
NDList output =
@@ -105,8 +96,7 @@ public void testSingleShotDetectionShape() {
Assert.assertEquals(output.get(0).getShape(), new Shape(1, 5444, 4));
Assert.assertEquals(output.get(1).getShape(), new Shape(32, 5444, 2));
Assert.assertEquals(output.get(2).getShape(), new Shape(32, 21776));
- Shape[] outputShapes =
- ssd.getOutputShapes(manager, new Shape[] {new Shape(32, 3, 256, 256)});
+ Shape[] outputShapes = ssd.getOutputShapes(new Shape[] {new Shape(32, 3, 256, 256)});
Assert.assertEquals(outputShapes[0], new Shape(1, 5444, 4));
Assert.assertEquals(outputShapes[1], new Shape(32, 5444, 2));
Assert.assertEquals(outputShapes[2], new Shape(32, 21776));
diff --git a/integration/src/main/java/ai/djl/integration/tests/modality/nlp/SimpleTextEncoderTest.java b/integration/src/main/java/ai/djl/integration/tests/modality/nlp/SimpleTextEncoderTest.java
index 131df85c53e..cc6d43853c8 100644
--- a/integration/src/main/java/ai/djl/integration/tests/modality/nlp/SimpleTextEncoderTest.java
+++ b/integration/src/main/java/ai/djl/integration/tests/modality/nlp/SimpleTextEncoderTest.java
@@ -23,7 +23,6 @@
import ai.djl.ndarray.types.Shape;
import ai.djl.nn.recurrent.LSTM;
import ai.djl.training.ParameterStore;
-import ai.djl.training.initializer.XavierInitializer;
import java.util.Arrays;
import org.testng.Assert;
import org.testng.annotations.Test;
@@ -50,7 +49,6 @@ public void testEncoder() {
.optReturnState(true)
.build());
try (NDManager manager = NDManager.newBaseManager(TestUtils.getDevices()[0])) {
- encoder.setInitializer(new XavierInitializer());
encoder.initialize(manager, DataType.FLOAT32, new Shape(4, 7));
NDList output =
encoder.forward(
diff --git a/integration/src/main/java/ai/djl/integration/tests/model_zoo/classification/AlexNetTest.java b/integration/src/main/java/ai/djl/integration/tests/model_zoo/classification/AlexNetTest.java
index f956bfb46bc..ae0ea251e24 100644
--- a/integration/src/main/java/ai/djl/integration/tests/model_zoo/classification/AlexNetTest.java
+++ b/integration/src/main/java/ai/djl/integration/tests/model_zoo/classification/AlexNetTest.java
@@ -159,7 +159,7 @@ public void testOutputShapes() {
Shape currentShape = x.getShape();
Block alexNet = AlexNet.builder().build();
- alexNet.setInitializer(Initializer.ONES);
+ alexNet.setInitializer(Initializer.ONES, Parameter.Type.WEIGHT);
alexNet.initialize(manager, DataType.FLOAT32, currentShape);
Map shapeMap = new ConcurrentHashMap<>();
@@ -169,7 +169,7 @@ public void testOutputShapes() {
alexNet.getChildren()
.get(i)
.getValue()
- .getOutputShapes(manager, new Shape[] {currentShape});
+ .getOutputShapes(new Shape[] {currentShape});
currentShape = newShape[0];
shapeMap.put(alexNet.getChildren().get(i).getKey(), currentShape);
}
@@ -188,7 +188,7 @@ public void testForwardMethod() {
Block alexNet = AlexNet.builder().build();
int batchSize = 1;
NDArray x = manager.ones(new Shape(batchSize, 1, 224, 224));
- alexNet.setInitializer(Initializer.ONES);
+ alexNet.setInitializer(Initializer.ONES, Parameter.Type.WEIGHT);
alexNet.initialize(manager, DataType.FLOAT32, x.getShape());
NDArray xHat =
alexNet.forward(new ParameterStore(manager, true), new NDList(x), false)
diff --git a/integration/src/main/java/ai/djl/integration/tests/model_zoo/classification/GoogLeNetTest.java b/integration/src/main/java/ai/djl/integration/tests/model_zoo/classification/GoogLeNetTest.java
index a149b7e40e3..4befb517a2f 100644
--- a/integration/src/main/java/ai/djl/integration/tests/model_zoo/classification/GoogLeNetTest.java
+++ b/integration/src/main/java/ai/djl/integration/tests/model_zoo/classification/GoogLeNetTest.java
@@ -100,7 +100,7 @@ public void testOutputShapes() {
Shape currentShape = x.getShape();
Block googLeNet = GoogLeNet.builder().build();
- googLeNet.setInitializer(Initializer.ONES);
+ googLeNet.setInitializer(Initializer.ONES, Parameter.Type.WEIGHT);
googLeNet.initialize(manager, DataType.FLOAT32, currentShape);
Map shapeMap = new ConcurrentHashMap<>();
@@ -111,7 +111,7 @@ public void testOutputShapes() {
.getChildren()
.get(i)
.getValue()
- .getOutputShapes(manager, new Shape[] {currentShape});
+ .getOutputShapes(new Shape[] {currentShape});
currentShape = newShape[0];
shapeMap.put(googLeNet.getChildren().get(i).getKey(), currentShape);
}
@@ -130,7 +130,7 @@ public void testForwardMethod() {
Block googLeNet = GoogLeNet.builder().build();
int batchSize = 1;
NDArray x = manager.ones(new Shape(batchSize, 1, 28, 28));
- googLeNet.setInitializer(Initializer.ONES);
+ googLeNet.setInitializer(Initializer.ONES, Parameter.Type.WEIGHT);
googLeNet.initialize(manager, DataType.FLOAT32, x.getShape());
NDArray xHat =
googLeNet
diff --git a/integration/src/main/java/ai/djl/integration/tests/model_zoo/classification/LeNetTest.java b/integration/src/main/java/ai/djl/integration/tests/model_zoo/classification/LeNetTest.java
index 39744eeefe6..6160ed5f7e4 100644
--- a/integration/src/main/java/ai/djl/integration/tests/model_zoo/classification/LeNetTest.java
+++ b/integration/src/main/java/ai/djl/integration/tests/model_zoo/classification/LeNetTest.java
@@ -137,7 +137,7 @@ public void testOutputShapes() {
Shape currentShape = x.getShape();
Block leNet = LeNet.builder().build();
- leNet.setInitializer(Initializer.ONES);
+ leNet.setInitializer(Initializer.ONES, Parameter.Type.WEIGHT);
leNet.initialize(manager, DataType.FLOAT32, currentShape);
Map shapeMap = new ConcurrentHashMap<>();
@@ -147,7 +147,7 @@ public void testOutputShapes() {
leNet.getChildren()
.get(i)
.getValue()
- .getOutputShapes(manager, new Shape[] {currentShape});
+ .getOutputShapes(new Shape[] {currentShape});
currentShape = newShape[0];
shapeMap.put(leNet.getChildren().get(i).getKey(), currentShape);
}
@@ -165,7 +165,7 @@ public void testForwardMethod() {
Block leNet = LeNet.builder().build();
int batchSize = 1;
NDArray x = manager.ones(new Shape(batchSize, 1, 28, 28));
- leNet.setInitializer(Initializer.ONES);
+ leNet.setInitializer(Initializer.ONES, Parameter.Type.WEIGHT);
leNet.initialize(manager, DataType.FLOAT32, x.getShape());
NDArray xHat =
leNet.forward(new ParameterStore(manager, true), new NDList(x), true)
diff --git a/integration/src/main/java/ai/djl/integration/tests/model_zoo/classification/NiNTest.java b/integration/src/main/java/ai/djl/integration/tests/model_zoo/classification/NiNTest.java
index cac77221d69..f8544debc70 100644
--- a/integration/src/main/java/ai/djl/integration/tests/model_zoo/classification/NiNTest.java
+++ b/integration/src/main/java/ai/djl/integration/tests/model_zoo/classification/NiNTest.java
@@ -152,17 +152,14 @@ public void testOutputShapes() {
Shape currentShape = x.getShape();
Block nin = NiN.builder().build();
- nin.setInitializer(Initializer.ONES);
+ nin.setInitializer(Initializer.ONES, Parameter.Type.WEIGHT);
nin.initialize(manager, DataType.FLOAT32, currentShape);
Map shapeMap = new ConcurrentHashMap<>();
for (int i = 0; i < nin.getChildren().size(); i++) {
Shape[] newShape =
- nin.getChildren()
- .get(i)
- .getValue()
- .getOutputShapes(manager, new Shape[] {currentShape});
+ nin.getChildren().get(i).getValue().getOutputShapes(new Shape[] {currentShape});
currentShape = newShape[0];
shapeMap.put(nin.getChildren().get(i).getKey(), currentShape);
}
@@ -180,7 +177,7 @@ public void testForwardMethod() {
Block nin = NiN.builder().build();
int batchSize = 1;
NDArray x = manager.ones(new Shape(batchSize, 1, 224, 224));
- nin.setInitializer(Initializer.ONES);
+ nin.setInitializer(Initializer.ONES, Parameter.Type.WEIGHT);
nin.initialize(manager, DataType.FLOAT32, x.getShape());
NDArray xHat =
nin.forward(new ParameterStore(manager, true), new NDList(x), false)
diff --git a/integration/src/main/java/ai/djl/integration/tests/model_zoo/classification/ResnetTest.java b/integration/src/main/java/ai/djl/integration/tests/model_zoo/classification/ResnetTest.java
index fd50e39943f..21c6779f36f 100644
--- a/integration/src/main/java/ai/djl/integration/tests/model_zoo/classification/ResnetTest.java
+++ b/integration/src/main/java/ai/djl/integration/tests/model_zoo/classification/ResnetTest.java
@@ -57,7 +57,7 @@ public void testTrain() {
TrainingConfig config =
new DefaultTrainingConfig(Loss.softmaxCrossEntropyLoss())
.optDevices(Device.getDevices(2))
- .optInitializer(Initializer.ONES);
+ .optInitializer(Initializer.ONES, Parameter.Type.WEIGHT);
Block resNet50 =
ResNetV1.builder()
@@ -123,7 +123,7 @@ public void testLoadTrain()
TrainingConfig config =
new DefaultTrainingConfig(Loss.l1Loss())
.optDevices(Device.getDevices(2))
- .optInitializer(Initializer.ONES);
+ .optInitializer(Initializer.ONES, Parameter.Type.WEIGHT);
try (Trainer trainer = model.newTrainer(config)) {
int batchSize = 2;
Shape inputShape = new Shape(batchSize, 3, 32, 32);
@@ -131,8 +131,7 @@ public void testLoadTrain()
trainer.initialize(inputShape);
NDManager manager = trainer.getManager();
- Shape[] outputShape =
- model.getBlock().getOutputShapes(manager, new Shape[] {inputShape});
+ Shape[] outputShape = model.getBlock().getOutputShapes(new Shape[] {inputShape});
NDArray data = manager.ones(new Shape(batchSize, 3, 32, 32));
NDArray label = manager.ones(outputShape[0]);
diff --git a/integration/src/main/java/ai/djl/integration/tests/model_zoo/classification/SqueezenetTest.java b/integration/src/main/java/ai/djl/integration/tests/model_zoo/classification/SqueezenetTest.java
index 267bd41cf80..114907144aa 100644
--- a/integration/src/main/java/ai/djl/integration/tests/model_zoo/classification/SqueezenetTest.java
+++ b/integration/src/main/java/ai/djl/integration/tests/model_zoo/classification/SqueezenetTest.java
@@ -40,7 +40,7 @@ public void testTrain() {
TrainingConfig config =
new DefaultTrainingConfig(Loss.softmaxCrossEntropyLoss())
.optDevices(Device.getDevices(2))
- .optInitializer(Initializer.ONES);
+ .optInitializer(Initializer.ONES, Parameter.Type.WEIGHT);
Block squeezeNet = SqueezeNet.squeezenet(10);
try (Model model = Model.newInstance("squeezenet")) {
model.setBlock(squeezeNet);
diff --git a/integration/src/main/java/ai/djl/integration/tests/model_zoo/classification/VGGTest.java b/integration/src/main/java/ai/djl/integration/tests/model_zoo/classification/VGGTest.java
index b8520edd8f9..5c22bacecf1 100644
--- a/integration/src/main/java/ai/djl/integration/tests/model_zoo/classification/VGGTest.java
+++ b/integration/src/main/java/ai/djl/integration/tests/model_zoo/classification/VGGTest.java
@@ -107,17 +107,14 @@ public void testOutputShapes() {
Shape currentShape = x.getShape();
Block vgg = VGG.builder().build();
- vgg.setInitializer(Initializer.ONES);
+ vgg.setInitializer(Initializer.ONES, Parameter.Type.WEIGHT);
vgg.initialize(manager, DataType.FLOAT32, currentShape);
Map shapeMap = new ConcurrentHashMap<>();
for (int i = 0; i < vgg.getChildren().size(); i++) {
Shape[] newShape =
- vgg.getChildren()
- .get(i)
- .getValue()
- .getOutputShapes(manager, new Shape[] {currentShape});
+ vgg.getChildren().get(i).getValue().getOutputShapes(new Shape[] {currentShape});
currentShape = newShape[0];
shapeMap.put(vgg.getChildren().get(i).getKey(), currentShape);
}
@@ -137,8 +134,9 @@ public void testForwardMethod() {
Block vgg = VGG.builder().build();
int batchSize = 1;
NDArray x = manager.ones(new Shape(batchSize, 1, 224, 224));
- vgg.setInitializer(Initializer.ONES);
+ vgg.setInitializer(Initializer.ONES, Parameter.Type.WEIGHT);
vgg.initialize(manager, DataType.FLOAT32, x.getShape());
+
NDArray xHat =
vgg.forward(new ParameterStore(manager, true), new NDList(x), false)
.singletonOrThrow();
diff --git a/integration/src/main/java/ai/djl/integration/tests/ndarray/NDArrayCreationOpTest.java b/integration/src/main/java/ai/djl/integration/tests/ndarray/NDArrayCreationOpTest.java
index 090e96c4b7d..9a020f41259 100644
--- a/integration/src/main/java/ai/djl/integration/tests/ndarray/NDArrayCreationOpTest.java
+++ b/integration/src/main/java/ai/djl/integration/tests/ndarray/NDArrayCreationOpTest.java
@@ -70,7 +70,7 @@ public void testCreateCSRMatrix() {
long[] indptr = {0, 2, 2, 3};
long[] indices = {0, 2, 1};
NDArray nd = manager.createCSR(buf, indptr, indices, new Shape(3, 4));
- float[] array = nd.toFloatArray();
+ float[] array = nd.toDense().toFloatArray();
Assert.assertEquals(array[0], expected[0]);
Assert.assertEquals(array[2], expected[1]);
Assert.assertEquals(array[9], expected[2]);
@@ -85,7 +85,7 @@ public void testCreateRowSparseMatrix() {
FloatBuffer buf = FloatBuffer.wrap(expected);
long[] indices = {0, 1, 3};
NDArray nd = manager.createRowSparse(buf, new Shape(3, 2), indices, new Shape(4, 2));
- float[] array = nd.toFloatArray();
+ float[] array = nd.toDense().toFloatArray();
Assert.assertEquals(array[0], expected[0]);
Assert.assertEquals(array[1], expected[1]);
Assert.assertEquals(array[2], expected[2]);
diff --git a/integration/src/main/java/ai/djl/integration/tests/ndarray/NDArrayElementArithmeticOpTest.java b/integration/src/main/java/ai/djl/integration/tests/ndarray/NDArrayElementArithmeticOpTest.java
index 8e97ed38b87..2dbfac8ef2e 100644
--- a/integration/src/main/java/ai/djl/integration/tests/ndarray/NDArrayElementArithmeticOpTest.java
+++ b/integration/src/main/java/ai/djl/integration/tests/ndarray/NDArrayElementArithmeticOpTest.java
@@ -18,6 +18,7 @@
import ai.djl.ndarray.NDManager;
import ai.djl.ndarray.types.Shape;
import ai.djl.nn.Blocks;
+import ai.djl.nn.Parameter;
import ai.djl.testing.Assertions;
import ai.djl.training.DefaultTrainingConfig;
import ai.djl.training.GradientCollector;
@@ -139,7 +140,7 @@ public void testAddScalar() {
try (Trainer trainer =
model.newTrainer(
new DefaultTrainingConfig(Loss.l2Loss())
- .optInitializer(Initializer.ONES))) {
+ .optInitializer(Initializer.ONES, Parameter.Type.WEIGHT))) {
try (GradientCollector gradCol = trainer.newGradientCollector()) {
lhs.attachGradient();
result = NDArrays.add(lhs, 2);
@@ -360,7 +361,7 @@ public void testDot() {
try (Trainer trainer =
model.newTrainer(
new DefaultTrainingConfig(Loss.l2Loss())
- .optInitializer(Initializer.ONES))) {
+ .optInitializer(Initializer.ONES, Parameter.Type.WEIGHT))) {
try (GradientCollector gradCol = trainer.newGradientCollector()) {
lhs.attachGradient();
result = NDArrays.dot(lhs, rhs);
diff --git a/integration/src/main/java/ai/djl/integration/tests/nn/BlockCoreTest.java b/integration/src/main/java/ai/djl/integration/tests/nn/BlockCoreTest.java
index b0289856ae9..c133b382327 100644
--- a/integration/src/main/java/ai/djl/integration/tests/nn/BlockCoreTest.java
+++ b/integration/src/main/java/ai/djl/integration/tests/nn/BlockCoreTest.java
@@ -62,7 +62,8 @@ public class BlockCoreTest {
@Test
public void testLinear() throws IOException, MalformedModelException {
TrainingConfig config =
- new DefaultTrainingConfig(Loss.l2Loss()).optInitializer(Initializer.ONES);
+ new DefaultTrainingConfig(Loss.l2Loss())
+ .optInitializer(Initializer.ONES, Parameter.Type.WEIGHT);
long outSize = 3;
Block block = Linear.builder().setUnits(outSize).build();
@@ -124,7 +125,8 @@ public void testLinear() throws IOException, MalformedModelException {
@Test
public void testLinearWithDefinedLayout() throws IOException, MalformedModelException {
TrainingConfig config =
- new DefaultTrainingConfig(Loss.l2Loss()).optInitializer(Initializer.ONES);
+ new DefaultTrainingConfig(Loss.l2Loss())
+ .optInitializer(Initializer.ONES, Parameter.Type.WEIGHT);
long outSize = 3;
Block block = Linear.builder().setUnits(outSize).build();
@@ -176,7 +178,8 @@ public void testLinearWithDefinedLayout() throws IOException, MalformedModelExce
@Test
public void testBatchNorm() throws IOException, MalformedModelException {
TrainingConfig config =
- new DefaultTrainingConfig(Loss.l2Loss()).optInitializer(Initializer.ONES);
+ new DefaultTrainingConfig(Loss.l2Loss())
+ .optInitializer(Initializer.ONES, Parameter.Type.WEIGHT);
Block block = BatchNorm.builder().build();
try (Model model = Model.newInstance("model")) {
@@ -203,7 +206,8 @@ public void testBatchNorm() throws IOException, MalformedModelException {
@Test
public void testDropout() throws IOException, MalformedModelException {
TrainingConfig config =
- new DefaultTrainingConfig(Loss.l2Loss()).optInitializer(Initializer.ONES);
+ new DefaultTrainingConfig(Loss.l2Loss())
+ .optInitializer(Initializer.ONES, Parameter.Type.WEIGHT);
Block block = Dropout.builder().optRate(.5f).build();
try (Model model = Model.newInstance("model")) {
@@ -229,7 +233,8 @@ public void testDropout() throws IOException, MalformedModelException {
@Test
public void testEmbedding() throws IOException, MalformedModelException {
TrainingConfig config =
- new DefaultTrainingConfig(Loss.l2Loss()).optInitializer(Initializer.ONES);
+ new DefaultTrainingConfig(Loss.l2Loss())
+ .optInitializer(Initializer.ONES, Parameter.Type.WEIGHT);
TrainableWordEmbedding block =
TrainableWordEmbedding.builder()
@@ -262,7 +267,8 @@ public void testEmbedding() throws IOException, MalformedModelException {
@Test
public void testConv1d() throws IOException, MalformedModelException {
TrainingConfig config =
- new DefaultTrainingConfig(Loss.l2Loss()).optInitializer(Initializer.ONES);
+ new DefaultTrainingConfig(Loss.l2Loss())
+ .optInitializer(Initializer.ONES, Parameter.Type.WEIGHT);
Block block =
Conv1d.builder().setKernelShape(new Shape(2)).setFilters(1).optBias(false).build();
@@ -283,7 +289,7 @@ public void testConv1d() throws IOException, MalformedModelException {
NDArray out = trainer.forward(new NDList(data)).singletonOrThrow();
Assert.assertEquals(out, expected);
- Shape[] outputShape = block.getOutputShapes(manager, new Shape[] {inputShape});
+ Shape[] outputShape = block.getOutputShapes(new Shape[] {inputShape});
Assert.assertEquals(out.getShape(), outputShape[0]);
testEncode(manager, block);
@@ -294,7 +300,8 @@ public void testConv1d() throws IOException, MalformedModelException {
@Test
public void testConv1dTranspose() throws IOException, MalformedModelException {
TrainingConfig config =
- new DefaultTrainingConfig(Loss.l2Loss()).optInitializer(Initializer.ONES);
+ new DefaultTrainingConfig(Loss.l2Loss())
+ .optInitializer(Initializer.ONES, Parameter.Type.WEIGHT);
Block block =
Conv1dTranspose.builder()
@@ -317,7 +324,7 @@ public void testConv1dTranspose() throws IOException, MalformedModelException {
NDArray out = trainer.forward(new NDList(data)).singletonOrThrow();
Assert.assertEquals(out, expected);
- Shape[] outputShape = block.getOutputShapes(manager, new Shape[] {inputShape});
+ Shape[] outputShape = block.getOutputShapes(new Shape[] {inputShape});
Assert.assertEquals(out.getShape(), outputShape[0]);
testEncode(manager, block);
@@ -328,7 +335,8 @@ public void testConv1dTranspose() throws IOException, MalformedModelException {
@Test
public void testConv2d() throws IOException, MalformedModelException {
TrainingConfig config =
- new DefaultTrainingConfig(Loss.l2Loss()).optInitializer(Initializer.ONES);
+ new DefaultTrainingConfig(Loss.l2Loss())
+ .optInitializer(Initializer.ONES, Parameter.Type.WEIGHT);
Block block = Conv2d.builder().setKernelShape(new Shape(2, 2)).setFilters(1).build();
try (Model model = Model.newInstance("model")) {
@@ -359,7 +367,8 @@ public void testConv2d() throws IOException, MalformedModelException {
@Test
public void testConv2dTranspose() throws IOException, MalformedModelException {
TrainingConfig config =
- new DefaultTrainingConfig(Loss.l2Loss()).optInitializer(Initializer.ONES);
+ new DefaultTrainingConfig(Loss.l2Loss())
+ .optInitializer(Initializer.ONES, Parameter.Type.WEIGHT);
Block block =
Conv2dTranspose.builder().setKernelShape(new Shape(2, 2)).setFilters(1).build();
@@ -396,7 +405,8 @@ public void testConv2dTranspose() throws IOException, MalformedModelException {
@Test
public void testConv3d() throws IOException, MalformedModelException {
TrainingConfig config =
- new DefaultTrainingConfig(Loss.l2Loss()).optInitializer(Initializer.ONES);
+ new DefaultTrainingConfig(Loss.l2Loss())
+ .optInitializer(Initializer.ONES, Parameter.Type.WEIGHT);
Block block = Conv3d.builder().setKernelShape(new Shape(2, 2, 2)).setFilters(1).build();
try (Model model = Model.newInstance("model")) {
@@ -422,8 +432,7 @@ public void testConv3d() throws IOException, MalformedModelException {
NDArray result = trainer.forward(new NDList(data)).singletonOrThrow();
Assert.assertEquals(result, expected);
- Shape[] outputShape =
- block.getOutputShapes(manager, new Shape[] {new Shape(1, 1, 3, 3, 3)});
+ Shape[] outputShape = block.getOutputShapes(new Shape[] {new Shape(1, 1, 3, 3, 3)});
Assert.assertEquals(result.getShape(), outputShape[0]);
testEncode(manager, block);
@@ -437,7 +446,7 @@ public void testRNNTanh() throws IOException, MalformedModelException {
Loss loss = new SoftmaxCrossEntropyLoss("SmCeLoss", 1, -1, false, true);
TrainingConfig config =
new DefaultTrainingConfig(loss)
- .optInitializer(Initializer.ONES)
+ .optInitializer(Initializer.ONES, Parameter.Type.WEIGHT)
.optDevices(TestUtils.getDevices());
Block block =
RNN.builder()
@@ -484,7 +493,7 @@ public void testRNNRelu() throws IOException, MalformedModelException {
Loss loss = new SoftmaxCrossEntropyLoss("SmCeLoss", 1, -1, false, true);
TrainingConfig config =
new DefaultTrainingConfig(loss)
- .optInitializer(Initializer.ONES)
+ .optInitializer(Initializer.ONES, Parameter.Type.WEIGHT)
.optDevices(TestUtils.getDevices());
Block block =
RNN.builder()
@@ -534,7 +543,7 @@ public void testLstm() throws IOException, MalformedModelException {
Loss loss = new SoftmaxCrossEntropyLoss("SmCeLoss", 1, -1, false, true);
TrainingConfig config =
new DefaultTrainingConfig(loss)
- .optInitializer(Initializer.ONES)
+ .optInitializer(Initializer.ONES, Parameter.Type.WEIGHT)
.optDevices(TestUtils.getDevices());
Block block =
LSTM.builder()
@@ -585,7 +594,7 @@ public void testGRU() throws IOException, MalformedModelException {
Loss loss = new SoftmaxCrossEntropyLoss("SmCeLoss", 1, -1, false, true);
TrainingConfig config =
new DefaultTrainingConfig(loss)
- .optInitializer(Initializer.ONES)
+ .optInitializer(Initializer.ONES, Parameter.Type.WEIGHT)
.optDevices(TestUtils.getDevices());
GRU block =
GRU.builder()
@@ -638,7 +647,8 @@ public void testGRU() throws IOException, MalformedModelException {
@Test
public void testSequentialBlock() throws IOException, MalformedModelException {
TrainingConfig config =
- new DefaultTrainingConfig(Loss.l2Loss()).optInitializer(Initializer.ONES);
+ new DefaultTrainingConfig(Loss.l2Loss())
+ .optInitializer(Initializer.ONES, Parameter.Type.WEIGHT);
SequentialBlock block = new SequentialBlock();
block.addSingleton(x -> x.mul(6.5f));
block.add(Linear.builder().setUnits(10).build());
@@ -678,7 +688,8 @@ public void testSequentialBlock() throws IOException, MalformedModelException {
@Test
public void testParallelBlock() throws IOException, MalformedModelException {
TrainingConfig config =
- new DefaultTrainingConfig(Loss.l2Loss()).optInitializer(Initializer.ONES);
+ new DefaultTrainingConfig(Loss.l2Loss())
+ .optInitializer(Initializer.ONES, Parameter.Type.WEIGHT);
ParallelBlock block =
new ParallelBlock(
list ->
diff --git a/integration/src/main/java/ai/djl/integration/tests/nn/BlockFactoryTest.java b/integration/src/main/java/ai/djl/integration/tests/nn/BlockFactoryTest.java
new file mode 100644
index 00000000000..f65e3052be0
--- /dev/null
+++ b/integration/src/main/java/ai/djl/integration/tests/nn/BlockFactoryTest.java
@@ -0,0 +1,116 @@
+/*
+ * Copyright 2021 Amazon.com, Inc. or its affiliates. All Rights Reserved.
+ *
+ * Licensed under the Apache License, Version 2.0 (the "License"). You may not use this file except in compliance
+ * with the License. A copy of the License is located at
+ *
+ * http://aws.amazon.com/apache2.0/
+ *
+ * or in the "license" file accompanying this file. This file is distributed on an "AS IS" BASIS, WITHOUT WARRANTIES
+ * OR CONDITIONS OF ANY KIND, either express or implied. See the License for the specific language governing permissions
+ * and limitations under the License.
+ */
+package ai.djl.integration.tests.nn;
+
+import ai.djl.Application;
+import ai.djl.MalformedModelException;
+import ai.djl.Model;
+import ai.djl.engine.Engine;
+import ai.djl.inference.Predictor;
+import ai.djl.modality.Classifications;
+import ai.djl.modality.cv.Image;
+import ai.djl.ndarray.NDList;
+import ai.djl.ndarray.NDManager;
+import ai.djl.ndarray.types.Shape;
+import ai.djl.nn.Block;
+import ai.djl.nn.BlockFactory;
+import ai.djl.nn.Blocks;
+import ai.djl.nn.SequentialBlock;
+import ai.djl.nn.SymbolBlock;
+import ai.djl.nn.core.Linear;
+import ai.djl.repository.zoo.Criteria;
+import ai.djl.repository.zoo.ModelNotFoundException;
+import ai.djl.repository.zoo.ModelZoo;
+import ai.djl.testing.Assertions;
+import ai.djl.training.ParameterStore;
+import ai.djl.training.util.ProgressBar;
+import ai.djl.translate.NoopTranslator;
+import ai.djl.translate.TranslateException;
+import java.io.ByteArrayInputStream;
+import java.io.ByteArrayOutputStream;
+import java.io.DataInputStream;
+import java.io.DataOutputStream;
+import java.io.IOException;
+import org.testng.annotations.Test;
+
+public class BlockFactoryTest {
+
+ @Test
+ public void testBlockLoadingSaving()
+ throws IOException, ModelNotFoundException, MalformedModelException,
+ TranslateException {
+ TestBlockFactory factory = new TestBlockFactory();
+ Model model = factory.getRemoveLastBlockModel();
+ try (NDManager manager = NDManager.newBaseManager()) {
+ Block block = model.getBlock();
+ block.forward(
+ new ParameterStore(manager, true),
+ new NDList(manager.ones(new Shape(1, 3, 32, 32))),
+ true);
+ ByteArrayOutputStream os = new ByteArrayOutputStream();
+ block.saveParameters(new DataOutputStream(os));
+ ByteArrayInputStream bis = new ByteArrayInputStream(os.toByteArray());
+ Block newBlock = factory.newBlock(manager);
+ newBlock.loadParameters(manager, new DataInputStream(bis));
+ try (Model test = Model.newInstance("test")) {
+ test.setBlock(newBlock);
+ try (Predictor predOrigin =
+ model.newPredictor(new NoopTranslator());
+ Predictor predDest =
+ test.newPredictor(new NoopTranslator())) {
+ NDList input = new NDList(manager.ones(new Shape(1, 3, 32, 32)));
+ NDList originOut = predOrigin.predict(input);
+ NDList destOut = predDest.predict(input);
+ Assertions.assertAlmostEquals(originOut, destOut);
+ }
+ }
+ }
+ }
+
+ static class TestBlockFactory implements BlockFactory {
+
+ private static final long serialVersionUID = 1234567L;
+
+ @Override
+ public Block newBlock(NDManager manager) {
+ SequentialBlock newBlock = new SequentialBlock();
+ newBlock.add(SymbolBlock.newInstance(manager));
+ newBlock.add(Blocks.batchFlattenBlock());
+ newBlock.add(Linear.builder().setUnits(10).build());
+ return newBlock;
+ }
+
+ public Model getRemoveLastBlockModel()
+ throws MalformedModelException, ModelNotFoundException, IOException {
+ String name = Engine.getInstance().getEngineName();
+ Criteria.Builder builder =
+ Criteria.builder()
+ .optApplication(Application.CV.IMAGE_CLASSIFICATION)
+ .setTypes(Image.class, Classifications.class)
+ .optProgress(new ProgressBar())
+ .optArtifactId("resnet")
+ .optEngine(name)
+ .optGroupId("ai.djl." + name.toLowerCase())
+ .optFilter("layers", "50");
+ Model model = ModelZoo.loadModel(builder.build());
+ SequentialBlock newBlock = new SequentialBlock();
+ SymbolBlock block = (SymbolBlock) model.getBlock();
+ block.removeLastBlock();
+ newBlock.add(block);
+ newBlock.add(Blocks.batchFlattenBlock());
+ newBlock.add(Linear.builder().setUnits(10).build());
+ model.setBlock(newBlock);
+ return model;
+ }
+ }
+}
diff --git a/integration/src/main/java/ai/djl/integration/tests/nn/PoolingOperationsTest.java b/integration/src/main/java/ai/djl/integration/tests/nn/PoolingOperationsTest.java
index 6f5f4e1ebc3..44a4e9816dd 100644
--- a/integration/src/main/java/ai/djl/integration/tests/nn/PoolingOperationsTest.java
+++ b/integration/src/main/java/ai/djl/integration/tests/nn/PoolingOperationsTest.java
@@ -18,6 +18,7 @@
import ai.djl.ndarray.NDManager;
import ai.djl.ndarray.index.NDIndex;
import ai.djl.ndarray.types.Shape;
+import ai.djl.nn.Parameter;
import ai.djl.nn.pooling.Pool;
import ai.djl.training.DefaultTrainingConfig;
import ai.djl.training.Trainer;
@@ -29,7 +30,8 @@
public class PoolingOperationsTest {
TrainingConfig config =
- new DefaultTrainingConfig(Loss.l2Loss()).optInitializer(Initializer.ONES);
+ new DefaultTrainingConfig(Loss.l2Loss())
+ .optInitializer(Initializer.ONES, Parameter.Type.WEIGHT);
@Test
public void testMaxPool1d() {
diff --git a/integration/src/main/java/ai/djl/integration/tests/nn/ScaledDotProductAttentionBlockTest.java b/integration/src/main/java/ai/djl/integration/tests/nn/ScaledDotProductAttentionBlockTest.java
index 9595e88ef15..df8a64f4803 100644
--- a/integration/src/main/java/ai/djl/integration/tests/nn/ScaledDotProductAttentionBlockTest.java
+++ b/integration/src/main/java/ai/djl/integration/tests/nn/ScaledDotProductAttentionBlockTest.java
@@ -19,6 +19,7 @@
import ai.djl.ndarray.NDManager;
import ai.djl.ndarray.types.DataType;
import ai.djl.ndarray.types.Shape;
+import ai.djl.nn.Parameter;
import ai.djl.nn.transformer.ScaledDotProductAttentionBlock;
import ai.djl.training.GradientCollector;
import ai.djl.training.ParameterStore;
@@ -752,7 +753,7 @@ public void testMaskedAttention() {
.optAttentionProbsDropoutProb(0.0f)
.build();
- block.setInitializer(new NormalInitializer());
+ block.setInitializer(new NormalInitializer(), Parameter.Type.WEIGHT);
block.getKeyProjection().setInitializer(keyKernelInitializer, "weight");
block.getValueProjection().setInitializer(valueKernelInitializer, "weight");
block.getQueryProjection().setInitializer(queryKernelInitializer, "weight");
diff --git a/integration/src/main/java/ai/djl/integration/tests/training/ActivationTest.java b/integration/src/main/java/ai/djl/integration/tests/training/ActivationTest.java
index 443370b10d8..b3e8502a9ca 100644
--- a/integration/src/main/java/ai/djl/integration/tests/training/ActivationTest.java
+++ b/integration/src/main/java/ai/djl/integration/tests/training/ActivationTest.java
@@ -18,6 +18,7 @@
import ai.djl.ndarray.NDManager;
import ai.djl.ndarray.types.Shape;
import ai.djl.nn.Activation;
+import ai.djl.nn.Parameter;
import ai.djl.testing.Assertions;
import ai.djl.training.DefaultTrainingConfig;
import ai.djl.training.Trainer;
@@ -30,7 +31,8 @@
public class ActivationTest {
TrainingConfig config =
- new DefaultTrainingConfig(Loss.l2Loss()).optInitializer(Initializer.ONES);
+ new DefaultTrainingConfig(Loss.l2Loss())
+ .optInitializer(Initializer.ONES, Parameter.Type.WEIGHT);
@Test
public void testRelu() {
diff --git a/integration/src/main/java/ai/djl/integration/tests/training/BlocksTest.java b/integration/src/main/java/ai/djl/integration/tests/training/BlocksTest.java
index a86705162ef..630ad57d3e6 100644
--- a/integration/src/main/java/ai/djl/integration/tests/training/BlocksTest.java
+++ b/integration/src/main/java/ai/djl/integration/tests/training/BlocksTest.java
@@ -18,6 +18,7 @@
import ai.djl.ndarray.NDManager;
import ai.djl.ndarray.types.Shape;
import ai.djl.nn.Blocks;
+import ai.djl.nn.Parameter;
import ai.djl.testing.Assertions;
import ai.djl.training.DefaultTrainingConfig;
import ai.djl.training.ParameterStore;
@@ -30,7 +31,8 @@
public class BlocksTest {
TrainingConfig config =
- new DefaultTrainingConfig(Loss.l2Loss()).optInitializer(Initializer.ONES);
+ new DefaultTrainingConfig(Loss.l2Loss())
+ .optInitializer(Initializer.ONES, Parameter.Type.WEIGHT);
@Test
public void testFlattenBlock() {
diff --git a/integration/src/main/java/ai/djl/integration/tests/training/DatasetTest.java b/integration/src/main/java/ai/djl/integration/tests/training/DatasetTest.java
index 4b5dd466c31..8d06c85efd8 100644
--- a/integration/src/main/java/ai/djl/integration/tests/training/DatasetTest.java
+++ b/integration/src/main/java/ai/djl/integration/tests/training/DatasetTest.java
@@ -20,6 +20,7 @@
import ai.djl.ndarray.types.DataType;
import ai.djl.ndarray.types.Shape;
import ai.djl.nn.Blocks;
+import ai.djl.nn.Parameter;
import ai.djl.training.DefaultTrainingConfig;
import ai.djl.training.Trainer;
import ai.djl.training.TrainingConfig;
@@ -49,7 +50,8 @@
public class DatasetTest {
private TrainingConfig config =
- new DefaultTrainingConfig(Loss.l2Loss()).optInitializer(Initializer.ONES);
+ new DefaultTrainingConfig(Loss.l2Loss())
+ .optInitializer(Initializer.ONES, Parameter.Type.WEIGHT);
@Test
public void testSequenceSampler() throws IOException, TranslateException {
diff --git a/integration/src/main/java/ai/djl/integration/tests/training/GradientCollectorIntegrationTest.java b/integration/src/main/java/ai/djl/integration/tests/training/GradientCollectorIntegrationTest.java
index 154583e59e3..96680659f78 100644
--- a/integration/src/main/java/ai/djl/integration/tests/training/GradientCollectorIntegrationTest.java
+++ b/integration/src/main/java/ai/djl/integration/tests/training/GradientCollectorIntegrationTest.java
@@ -19,6 +19,7 @@
import ai.djl.ndarray.types.DataType;
import ai.djl.ndarray.types.Shape;
import ai.djl.nn.Blocks;
+import ai.djl.nn.Parameter;
import ai.djl.nn.core.Linear;
import ai.djl.testing.Assertions;
import ai.djl.training.DefaultTrainingConfig;
@@ -49,7 +50,7 @@ public void testAutograd() {
try (Trainer trainer =
model.newTrainer(
new DefaultTrainingConfig(Loss.l2Loss())
- .optInitializer(Initializer.ONES))) {
+ .optInitializer(Initializer.ONES, Parameter.Type.WEIGHT))) {
try (GradientCollector gradCol = trainer.newGradientCollector()) {
NDArray lhs =
manager.create(new float[] {6, -9, -12, 15, 0, 4}, new Shape(2, 3));
@@ -87,7 +88,7 @@ public void testTrain() throws IOException, TranslateException {
TrainingConfig config =
new DefaultTrainingConfig(Loss.l2Loss())
.addTrainingListeners(new EvaluatorTrainingListener())
- .optInitializer(Initializer.ONES)
+ .optInitializer(Initializer.ONES, Parameter.Type.WEIGHT)
.optOptimizer(optimizer);
try (Model model = Model.newInstance("linear")) {
diff --git a/integration/src/main/java/ai/djl/integration/tests/training/ModelTest.java b/integration/src/main/java/ai/djl/integration/tests/training/ModelTest.java
index 2e919ca78d5..6023b1b054f 100644
--- a/integration/src/main/java/ai/djl/integration/tests/training/ModelTest.java
+++ b/integration/src/main/java/ai/djl/integration/tests/training/ModelTest.java
@@ -21,7 +21,6 @@
import ai.djl.nn.convolutional.Conv2d;
import ai.djl.nn.norm.BatchNorm;
import ai.djl.testing.Assertions;
-import ai.djl.training.initializer.XavierInitializer;
import java.io.IOException;
import java.nio.file.Paths;
import org.testng.Assert;
@@ -36,7 +35,6 @@ public void testModelSaveAndLoad() throws IOException, MalformedModelException {
block.add(BatchNorm.builder().build());
try (Model saveModel = Model.newInstance("saveModel");
Model loadModel = Model.newInstance("loadModel")) {
- block.setInitializer(new XavierInitializer());
block.initialize(saveModel.getNDManager(), DataType.FLOAT32, new Shape(1, 3, 32, 32));
ParameterList savedParameters = block.getParameters();
saveModel.setBlock(block);
diff --git a/integration/src/main/java/ai/djl/integration/tests/training/OptimizerTest.java b/integration/src/main/java/ai/djl/integration/tests/training/OptimizerTest.java
index 8e472c626f2..5657f584805 100644
--- a/integration/src/main/java/ai/djl/integration/tests/training/OptimizerTest.java
+++ b/integration/src/main/java/ai/djl/integration/tests/training/OptimizerTest.java
@@ -20,6 +20,7 @@
import ai.djl.ndarray.NDManager;
import ai.djl.ndarray.types.Shape;
import ai.djl.nn.Block;
+import ai.djl.nn.Parameter;
import ai.djl.nn.core.Linear;
import ai.djl.testing.Assertions;
import ai.djl.training.DefaultTrainingConfig;
@@ -46,7 +47,7 @@ public void testSgd() {
Device[] devices = Device.getDevices(1);
TrainingConfig config =
new DefaultTrainingConfig(Loss.l2Loss())
- .optInitializer(Initializer.ONES)
+ .optInitializer(Initializer.ONES, Parameter.Type.WEIGHT)
.optOptimizer(sgd)
.optDevices(devices);
Block block = Linear.builder().setUnits(CHANNELS).build();
@@ -78,7 +79,7 @@ public void testSgdWithMomentum() {
Device[] devices = Device.getDevices(1);
TrainingConfig config =
new DefaultTrainingConfig(Loss.l2Loss())
- .optInitializer(Initializer.ONES)
+ .optInitializer(Initializer.ONES, Parameter.Type.WEIGHT)
.optOptimizer(optim)
.optDevices(devices);
Block block = Linear.builder().setUnits(CHANNELS).build();
@@ -118,7 +119,7 @@ public void testNag() {
Device[] devices = Device.getDevices(1);
TrainingConfig config =
new DefaultTrainingConfig(Loss.l2Loss())
- .optInitializer(Initializer.ONES)
+ .optInitializer(Initializer.ONES, Parameter.Type.WEIGHT)
.optOptimizer(optim)
.optDevices(devices);
Block block = Linear.builder().setUnits(CHANNELS).build();
@@ -147,7 +148,7 @@ public void testAdam() {
Device[] devices = Device.getDevices(1);
TrainingConfig config =
new DefaultTrainingConfig(Loss.l2Loss())
- .optInitializer(Initializer.ONES)
+ .optInitializer(Initializer.ONES, Parameter.Type.WEIGHT)
.optOptimizer(optim)
.optDevices(devices);
Block block = Linear.builder().setUnits(CHANNELS).build();
@@ -176,7 +177,7 @@ public void testAdagrad() {
Device[] devices = Device.getDevices(1);
TrainingConfig config =
new DefaultTrainingConfig(Loss.l2Loss())
- .optInitializer(Initializer.ONES)
+ .optInitializer(Initializer.ONES, Parameter.Type.WEIGHT)
.optOptimizer(optim)
.optDevices(devices);
Block block = Linear.builder().setUnits(CHANNELS).build();
@@ -209,7 +210,7 @@ public void testRMSProp() {
Device[] devices = Device.getDevices(1);
TrainingConfig config =
new DefaultTrainingConfig(Loss.l2Loss())
- .optInitializer(Initializer.ONES)
+ .optInitializer(Initializer.ONES, Parameter.Type.WEIGHT)
.optOptimizer(optim)
.optDevices(devices);
Block block = Linear.builder().setUnits(CHANNELS).build();
@@ -243,7 +244,7 @@ public void testRMSPropAlex() {
Device[] devices = Device.getDevices(1);
TrainingConfig config =
new DefaultTrainingConfig(Loss.l2Loss())
- .optInitializer(Initializer.ONES)
+ .optInitializer(Initializer.ONES, Parameter.Type.WEIGHT)
.optOptimizer(optim)
.optDevices(devices);
Block block = Linear.builder().setUnits(CHANNELS).build();
@@ -273,7 +274,7 @@ public void testAdadelta() {
Device[] devices = Device.getDevices(1);
TrainingConfig config =
new DefaultTrainingConfig(Loss.l2Loss())
- .optInitializer(Initializer.ONES)
+ .optInitializer(Initializer.ONES, Parameter.Type.WEIGHT)
.optOptimizer(optim)
.optDevices(devices);
diff --git a/jupyter/onnxruntime/machine_learning_with_ONNXRuntime.ipynb b/jupyter/onnxruntime/machine_learning_with_ONNXRuntime.ipynb
index 7ec80efabb9..7ab1b7a7106 100644
--- a/jupyter/onnxruntime/machine_learning_with_ONNXRuntime.ipynb
+++ b/jupyter/onnxruntime/machine_learning_with_ONNXRuntime.ipynb
@@ -178,7 +178,7 @@
"metadata": {},
"outputs": [],
"source": [
- "String modelUrl = \"https://mlrepo.djl.ai/model/tabular/random_forest/ai/djl/onnxruntime/iris_flowers/0.0.1/iris_flowers.zip\";\n",
+ "String modelUrl = \"https://mlrepo.djl.ai/model/tabular/softmax_regression/ai/djl/onnxruntime/iris_flowers/0.0.1/iris_flowers.zip\";\n",
"Criteria criteria = Criteria.builder()\n",
" .setTypes(IrisFlower.class, Classifications.class)\n",
" .optModelUrls(modelUrl)\n",
diff --git a/jupyter/paddlepaddle/face_mask_detection_paddlepaddle.ipynb b/jupyter/paddlepaddle/face_mask_detection_paddlepaddle.ipynb
index ef713ea9719..10e15035cb5 100644
--- a/jupyter/paddlepaddle/face_mask_detection_paddlepaddle.ipynb
+++ b/jupyter/paddlepaddle/face_mask_detection_paddlepaddle.ipynb
@@ -8,7 +8,7 @@
"\n",
"In this tutorial, we will be using pretrained PaddlePaddle model from [PaddleHub](https://github.com/PaddlePaddle/PaddleHub/tree/release/v1.5/demo/mask_detection/cpp) to do mask detection on the sample image. To complete this procedure, there are two steps needs to be done:\n",
"\n",
- "- Recognize face on the image (no maater wearing mask or not) using Face object detection model\n",
+ "- Recognize face on the image (no matter wearing mask or not) using Face object detection model\n",
"- classify the face is wearing mask or not\n",
"\n",
"These two steps will involve two paddle models. We will implement the corresponding preprocess and postprocess logic to it.\n",
diff --git a/jupyter/paddlepaddle/face_mask_detection_paddlepaddle_zh.ipynb b/jupyter/paddlepaddle/face_mask_detection_paddlepaddle_zh.ipynb
new file mode 100644
index 00000000000..0d6a9851179
--- /dev/null
+++ b/jupyter/paddlepaddle/face_mask_detection_paddlepaddle_zh.ipynb
@@ -0,0 +1,365 @@
+{
+ "cells": [
+ {
+ "cell_type": "markdown",
+ "metadata": {},
+ "source": [
+ "# 用飛槳+ DJL 實作人臉口罩辨識\n",
+ "在這個教學中我們將會展示利用 PaddleHub 下載預訓練好的 PaddlePaddle 模型並針對範例照片做人臉口罩辨識。這個範例總共會分成兩個步驟:\n",
+ "\n",
+ "- 用臉部檢測模型識別圖片中的人臉(無論是否有戴口罩) \n",
+ "- 確認圖片中的臉是否有戴口罩\n",
+ "\n",
+ "這兩個步驟會包含使用兩個 Paddle 模型,我們會在接下來的內容介紹兩個模型對應需要做的前後處理邏輯\n",
+ "\n",
+ "## 導入相關環境依賴及子類別\n",
+ "在這個例子中的前處理飛槳深度學習引擎需要搭配 DJL 混合模式進行深度學習推理,原因是引擎本身沒有包含 NDArray 操作,因此需要藉用其他引擎的 NDArray 操作能力來完成。這邊我們導入 PyTorch 來做協同的前處理工作:"
+ ]
+ },
+ {
+ "cell_type": "code",
+ "execution_count": null,
+ "metadata": {},
+ "outputs": [],
+ "source": [
+ "// %mavenRepo snapshots https://oss.sonatype.org/content/repositories/snapshots/\n",
+ "\n",
+ "%maven ai.djl:api:0.10.0\n",
+ "%maven ai.djl.paddlepaddle:paddlepaddle-model-zoo:0.10.0\n",
+ "%maven ai.djl.paddlepaddle:paddlepaddle-native-auto:2.0.0\n",
+ "%maven org.slf4j:slf4j-api:1.7.26\n",
+ "%maven org.slf4j:slf4j-simple:1.7.26\n",
+ "\n",
+ "// second engine to do preprocessing and postprocessing\n",
+ "%maven ai.djl.pytorch:pytorch-engine:0.10.0\n",
+ "%maven ai.djl.pytorch:pytorch-native-auto:1.7.1"
+ ]
+ },
+ {
+ "cell_type": "code",
+ "execution_count": null,
+ "metadata": {},
+ "outputs": [],
+ "source": [
+ "import ai.djl.Application;\n",
+ "import ai.djl.MalformedModelException;\n",
+ "import ai.djl.ModelException;\n",
+ "import ai.djl.inference.Predictor;\n",
+ "import ai.djl.modality.Classifications;\n",
+ "import ai.djl.modality.cv.*;\n",
+ "import ai.djl.modality.cv.output.*;\n",
+ "import ai.djl.modality.cv.transform.*;\n",
+ "import ai.djl.modality.cv.translator.ImageClassificationTranslator;\n",
+ "import ai.djl.modality.cv.util.NDImageUtils;\n",
+ "import ai.djl.ndarray.*;\n",
+ "import ai.djl.ndarray.types.Shape;\n",
+ "import ai.djl.repository.zoo.*;\n",
+ "import ai.djl.translate.*;\n",
+ "\n",
+ "import java.io.IOException;\n",
+ "import java.nio.file.*;\n",
+ "import java.util.*;"
+ ]
+ },
+ {
+ "cell_type": "markdown",
+ "metadata": {},
+ "source": [
+ "## 臉部偵測模型\n",
+ "現在我們可以開始處理第一個模型,在將圖片輸入臉部檢測模型前我們必須先做一些預處理:\n",
+ "•\t調整圖片尺寸: 以特定比例縮小圖片\n",
+ "•\t用一個數值對縮小後圖片正規化\n",
+ "對開發者來說好消息是,DJL 提供了 Translator 介面來幫助開發做這樣的預處理. 一個比較粗略的 Translator 架構如下:\n",
+ "\n",
+ "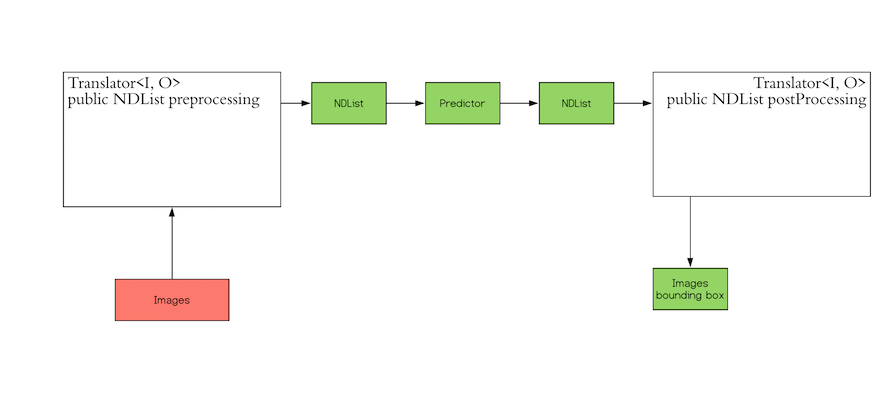\n",
+ "\n",
+ "在接下來的段落,我們會利用一個 FaceTranslator 子類別實作來完成工作\n",
+ "### 預處理\n",
+ "在這個階段我們會讀取一張圖片並且對其做一些事先的預處理,讓我們先示範讀取一張圖片:"
+ ]
+ },
+ {
+ "cell_type": "code",
+ "execution_count": null,
+ "metadata": {},
+ "outputs": [],
+ "source": [
+ "String url = \"https://raw.githubusercontent.com/PaddlePaddle/PaddleHub/release/v1.5/demo/mask_detection/python/images/mask.jpg\";\n",
+ "Image img = ImageFactory.getInstance().fromUrl(url);\n",
+ "img.getWrappedImage();"
+ ]
+ },
+ {
+ "cell_type": "markdown",
+ "metadata": {},
+ "source": [
+ "接著,讓我們試著對圖片做一些預處理的轉換:"
+ ]
+ },
+ {
+ "cell_type": "code",
+ "execution_count": null,
+ "metadata": {},
+ "outputs": [],
+ "source": [
+ "NDList processImageInput(NDManager manager, Image input, float shrink) {\n",
+ " NDArray array = input.toNDArray(manager);\n",
+ " Shape shape = array.getShape();\n",
+ " array = NDImageUtils.resize(\n",
+ " array, (int) (shape.get(1) * shrink), (int) (shape.get(0) * shrink));\n",
+ " array = array.transpose(2, 0, 1).flip(0); // HWC -> CHW BGR -> RGB\n",
+ " NDArray mean = manager.create(new float[] {104f, 117f, 123f}, new Shape(3, 1, 1));\n",
+ " array = array.sub(mean).mul(0.007843f); // normalization\n",
+ " array = array.expandDims(0); // make batch dimension\n",
+ " return new NDList(array);\n",
+ "}\n",
+ "\n",
+ "processImageInput(NDManager.newBaseManager(), img, 0.5f);"
+ ]
+ },
+ {
+ "cell_type": "markdown",
+ "metadata": {},
+ "source": [
+ "如上述所見,我們已經把圖片轉成如下尺寸的 NDArray: (披量, 通道(RGB), 高度, 寬度). 這是物件檢測模型輸入的格式\n",
+ "### 後處理\n",
+ "當我們做後處理時, 模型輸出的格式是 (number_of_boxes, (class_id, probability, xmin, ymin, xmax, ymax)). 我們可以將其存入預先建立好的 DJL 子類別 DetectedObjects 以便做後續操作. 我們假設有一組推論後的輸出是 ((1, 0.99, 0.2, 0.4, 0.5, 0.8)) 並且試著把人像框顯示在圖片上"
+ ]
+ },
+ {
+ "cell_type": "code",
+ "execution_count": null,
+ "metadata": {
+ "scrolled": false
+ },
+ "outputs": [],
+ "source": [
+ "DetectedObjects processImageOutput(NDList list, List className, float threshold) {\n",
+ " NDArray result = list.singletonOrThrow();\n",
+ " float[] probabilities = result.get(\":,1\").toFloatArray();\n",
+ " List names = new ArrayList<>();\n",
+ " List prob = new ArrayList<>();\n",
+ " List boxes = new ArrayList<>();\n",
+ " for (int i = 0; i < probabilities.length; i++) {\n",
+ " if (probabilities[i] >= threshold) {\n",
+ " float[] array = result.get(i).toFloatArray();\n",
+ " names.add(className.get((int) array[0]));\n",
+ " prob.add((double) probabilities[i]);\n",
+ " boxes.add(\n",
+ " new Rectangle(\n",
+ " array[2], array[3], array[4] - array[2], array[5] - array[3]));\n",
+ " }\n",
+ " }\n",
+ " return new DetectedObjects(names, prob, boxes);\n",
+ "}\n",
+ "\n",
+ "NDArray tempOutput = NDManager.newBaseManager().create(new float[]{1f, 0.99f, 0.1f, 0.1f, 0.2f, 0.2f}, new Shape(1, 6));\n",
+ "DetectedObjects testBox = processImageOutput(new NDList(tempOutput), Arrays.asList(\"Not Face\", \"Face\"), 0.7f);\n",
+ "Image newImage = img.duplicate(Image.Type.TYPE_INT_ARGB);\n",
+ "newImage.drawBoundingBoxes(testBox);\n",
+ "newImage.getWrappedImage();"
+ ]
+ },
+ {
+ "cell_type": "markdown",
+ "metadata": {},
+ "source": [
+ "### 生成一個翻譯器並執行推理任務\n",
+ "透過這個步驟,你會理解 DJL 中的前後處理如何運作,現在讓我們把前數的幾個步驟串在一起並對真實圖片進行操作:"
+ ]
+ },
+ {
+ "cell_type": "code",
+ "execution_count": null,
+ "metadata": {},
+ "outputs": [],
+ "source": [
+ "class FaceTranslator implements Translator {\n",
+ "\n",
+ " private float shrink;\n",
+ " private float threshold;\n",
+ " private List className;\n",
+ "\n",
+ " FaceTranslator(float shrink, float threshold) {\n",
+ " this.shrink = shrink;\n",
+ " this.threshold = threshold;\n",
+ " className = Arrays.asList(\"Not Face\", \"Face\");\n",
+ " }\n",
+ "\n",
+ " @Override\n",
+ " public DetectedObjects processOutput(TranslatorContext ctx, NDList list) {\n",
+ " return processImageOutput(list, className, threshold);\n",
+ " }\n",
+ "\n",
+ " @Override\n",
+ " public NDList processInput(TranslatorContext ctx, Image input) {\n",
+ " return processImageInput(ctx.getNDManager(), input, shrink);\n",
+ " }\n",
+ "\n",
+ " @Override\n",
+ " public Batchifier getBatchifier() {\n",
+ " return null;\n",
+ " }\n",
+ "}"
+ ]
+ },
+ {
+ "cell_type": "markdown",
+ "metadata": {},
+ "source": [
+ "要執行這個人臉檢測推理,我們必須先從 DJL 的 Paddle Model Zoo 讀取模型,在讀取模型之前我們必須指定好 `Crieteria` . `Crieteria` 是用來確認要從哪邊讀取模型而後執行 `Translator` 來進行模型導入. 接著,我們只要利用 `Predictor` 就可以開始進行推論"
+ ]
+ },
+ {
+ "cell_type": "code",
+ "execution_count": null,
+ "metadata": {},
+ "outputs": [],
+ "source": [
+ "Criteria criteria =\n",
+ " Criteria.builder()\n",
+ " .optApplication(Application.CV.OBJECT_DETECTION)\n",
+ " .setTypes(Image.class, DetectedObjects.class)\n",
+ " .optArtifactId(\"face_detection\")\n",
+ " .optTranslator(new FaceTranslator(0.5f, 0.7f))\n",
+ " .optFilter(\"flavor\", \"server\")\n",
+ " .build();\n",
+ " \n",
+ "var model = ModelZoo.loadModel(criteria);\n",
+ "var predictor = model.newPredictor();\n",
+ "\n",
+ "DetectedObjects inferenceResult = predictor.predict(img);\n",
+ "newImage = img.duplicate(Image.Type.TYPE_INT_ARGB);\n",
+ "newImage.drawBoundingBoxes(inferenceResult);\n",
+ "newImage.getWrappedImage();"
+ ]
+ },
+ {
+ "cell_type": "markdown",
+ "metadata": {},
+ "source": [
+ "如圖片所示,這個推論服務已經可以正確的辨識出圖片中的三張人臉\n",
+ "## 口罩分類模型\n",
+ "一旦有了圖片的座標,我們就可以將圖片裁剪到適當大小並且將其傳給口罩分類模型做後續的推論\n",
+ "### 圖片裁剪\n",
+ "圖中方框位置的數值範圍從0到1, 只要將這個數值乘上圖片的長寬我們就可以將方框對應到圖片中的準確位置. 為了使裁剪後的圖片有更好的精確度,我們將圖片裁剪成方形,讓我們示範一下:"
+ ]
+ },
+ {
+ "cell_type": "code",
+ "execution_count": null,
+ "metadata": {},
+ "outputs": [],
+ "source": [
+ "int[] extendSquare(\n",
+ " double xmin, double ymin, double width, double height, double percentage) {\n",
+ " double centerx = xmin + width / 2;\n",
+ " double centery = ymin + height / 2;\n",
+ " double maxDist = Math.max(width / 2, height / 2) * (1 + percentage);\n",
+ " return new int[] {\n",
+ " (int) (centerx - maxDist), (int) (centery - maxDist), (int) (2 * maxDist)\n",
+ " };\n",
+ "}\n",
+ "\n",
+ "Image getSubImage(Image img, BoundingBox box) {\n",
+ " Rectangle rect = box.getBounds();\n",
+ " int width = img.getWidth();\n",
+ " int height = img.getHeight();\n",
+ " int[] squareBox =\n",
+ " extendSquare(\n",
+ " rect.getX() * width,\n",
+ " rect.getY() * height,\n",
+ " rect.getWidth() * width,\n",
+ " rect.getHeight() * height,\n",
+ " 0.18);\n",
+ " return img.getSubimage(squareBox[0], squareBox[1], squareBox[2], squareBox[2]);\n",
+ "}\n",
+ "\n",
+ "List faces = inferenceResult.items();\n",
+ "getSubImage(img, faces.get(2).getBoundingBox()).getWrappedImage();"
+ ]
+ },
+ {
+ "cell_type": "markdown",
+ "metadata": {},
+ "source": [
+ "### 事先準備 Translator 並讀取模型\n",
+ "在使用臉部檢測模型的時候,我們可以利用 DJL 預先建好的 `ImageClassificationTranslator` 並且加上一些轉換。這個 Translator 提供了一些基礎的圖片翻譯處理並且同時包含一些進階的標準化圖片處理。以這個例子來說, 我們不需要額外建立新的 `Translator` 而使用預先建立的就可以"
+ ]
+ },
+ {
+ "cell_type": "code",
+ "execution_count": null,
+ "metadata": {},
+ "outputs": [],
+ "source": [
+ "var criteria = Criteria.builder()\n",
+ " .optApplication(Application.CV.IMAGE_CLASSIFICATION)\n",
+ " .setTypes(Image.class, Classifications.class)\n",
+ " .optTranslator(\n",
+ " ImageClassificationTranslator.builder()\n",
+ " .addTransform(new Resize(128, 128))\n",
+ " .addTransform(new ToTensor()) // HWC -> CHW div(255)\n",
+ " .addTransform(\n",
+ " new Normalize(\n",
+ " new float[] {0.5f, 0.5f, 0.5f},\n",
+ " new float[] {1.0f, 1.0f, 1.0f}))\n",
+ " .addTransform(nd -> nd.flip(0)) // RGB -> GBR\n",
+ " .build())\n",
+ " .optArtifactId(\"mask_classification\")\n",
+ " .optFilter(\"flavor\", \"server\")\n",
+ " .build();\n",
+ "\n",
+ "var classifyModel = ModelZoo.loadModel(criteria);\n",
+ "var classifier = classifyModel.newPredictor();"
+ ]
+ },
+ {
+ "cell_type": "markdown",
+ "metadata": {},
+ "source": [
+ "### 執行推論任務\n",
+ "最後,要完成一個口罩識別的任務,我們只需要將上述的步驟合在一起即可。我們先將圖片做裁剪後並對其做上述的推論操作,結束之後再生成一個新的分類子類別 `DetectedObjects`:"
+ ]
+ },
+ {
+ "cell_type": "code",
+ "execution_count": null,
+ "metadata": {},
+ "outputs": [],
+ "source": [
+ "List names = new ArrayList<>();\n",
+ "List prob = new ArrayList<>();\n",
+ "List rect = new ArrayList<>();\n",
+ "for (DetectedObjects.DetectedObject face : faces) {\n",
+ " Image subImg = getSubImage(img, face.getBoundingBox());\n",
+ " Classifications classifications = classifier.predict(subImg);\n",
+ " names.add(classifications.best().getClassName());\n",
+ " prob.add(face.getProbability());\n",
+ " rect.add(face.getBoundingBox());\n",
+ "}\n",
+ "\n",
+ "newImage = img.duplicate(Image.Type.TYPE_INT_ARGB);\n",
+ "newImage.drawBoundingBoxes(new DetectedObjects(names, prob, rect));\n",
+ "newImage.getWrappedImage();"
+ ]
+ }
+ ],
+ "metadata": {
+ "kernelspec": {
+ "display_name": "Java",
+ "language": "java",
+ "name": "java"
+ },
+ "language_info": {
+ "codemirror_mode": "java",
+ "file_extension": ".jshell",
+ "mimetype": "text/x-java-source",
+ "name": "Java",
+ "pygments_lexer": "java",
+ "version": "12.0.2+10"
+ }
+ },
+ "nbformat": 4,
+ "nbformat_minor": 4
+}
diff --git a/jupyter/paddlepaddle/paddle_ocr_java_zh.ipynb b/jupyter/paddlepaddle/paddle_ocr_java_zh.ipynb
new file mode 100644
index 00000000000..1df7e59ec2d
--- /dev/null
+++ b/jupyter/paddlepaddle/paddle_ocr_java_zh.ipynb
@@ -0,0 +1,322 @@
+{
+ "cells": [
+ {
+ "attachments": {},
+ "cell_type": "markdown",
+ "metadata": {},
+ "source": [
+ "# PaddleOCR在DJL 上的實現\n",
+ "在這個教程裡,我們會展示利用 PaddleOCR 下載預訓練好文字處理模型並對指定的照片進行文學文字檢測 (OCR)。這個教程總共會分成三個部分:\n",
+ "\n",
+ "- 文字區塊檢測: 從圖片檢測出文字區塊\n",
+ "- 文字角度檢測: 確認文字是否需要旋轉\n",
+ "- 文字識別: 確認區塊內的文字\n",
+ "\n",
+ "## 導入相關環境依賴及子類別\n",
+ "在這個例子中的前處理飛槳深度學習引擎需要搭配DJL混合模式進行深度學習推理,原因是引擎本身沒有包含ND數組操作,因此需要藉用其他引擎的數組操作能力來完成。這邊我們導入Pytorch來做協同的前處理工作:"
+ ]
+ },
+ {
+ "cell_type": "code",
+ "execution_count": null,
+ "metadata": {},
+ "outputs": [],
+ "source": [
+ "// %mavenRepo snapshots https://oss.sonatype.org/content/repositories/snapshots/\n",
+ "\n",
+ "%maven ai.djl:api:0.10.0\n",
+ "%maven ai.djl.paddlepaddle:paddlepaddle-model-zoo:0.10.0\n",
+ "%maven ai.djl.paddlepaddle:paddlepaddle-native-auto:2.0.0\n",
+ "%maven org.slf4j:slf4j-api:1.7.26\n",
+ "%maven org.slf4j:slf4j-simple:1.7.26\n",
+ "\n",
+ "// second engine to do preprocessing and postprocessing\n",
+ "%maven ai.djl.pytorch:pytorch-engine:0.10.0\n",
+ "%maven ai.djl.pytorch:pytorch-native-auto:1.7.1"
+ ]
+ },
+ {
+ "cell_type": "code",
+ "execution_count": null,
+ "metadata": {},
+ "outputs": [],
+ "source": [
+ "import ai.djl.*;\n",
+ "import ai.djl.inference.Predictor;\n",
+ "import ai.djl.modality.Classifications;\n",
+ "import ai.djl.modality.cv.Image;\n",
+ "import ai.djl.modality.cv.ImageFactory;\n",
+ "import ai.djl.modality.cv.output.*;\n",
+ "import ai.djl.modality.cv.util.NDImageUtils;\n",
+ "import ai.djl.ndarray.*;\n",
+ "import ai.djl.ndarray.types.DataType;\n",
+ "import ai.djl.ndarray.types.Shape;\n",
+ "import ai.djl.repository.zoo.*;\n",
+ "import ai.djl.paddlepaddle.zoo.cv.objectdetection.PpWordDetectionTranslator;\n",
+ "import ai.djl.paddlepaddle.zoo.cv.imageclassification.PpWordRotateTranslator;\n",
+ "import ai.djl.paddlepaddle.zoo.cv.wordrecognition.PpWordRecognitionTranslator;\n",
+ "import ai.djl.translate.*;\n",
+ "import java.util.concurrent.ConcurrentHashMap;"
+ ]
+ },
+ {
+ "attachments": {},
+ "cell_type": "markdown",
+ "metadata": {},
+ "source": [
+ "## 圖片讀取\n",
+ "首先讓我們載入這次教程會用到的機票範例圖片:"
+ ]
+ },
+ {
+ "cell_type": "code",
+ "execution_count": null,
+ "metadata": {},
+ "outputs": [],
+ "source": [
+ "String url = \"https://resources.djl.ai/images/flight_ticket.jpg\";\n",
+ "Image img = ImageFactory.getInstance().fromUrl(url);\n",
+ "img.getWrappedImage();"
+ ]
+ },
+ {
+ "attachments": {},
+ "cell_type": "markdown",
+ "metadata": {},
+ "source": [
+ "## 文字區塊檢測\n",
+ "我們首先從 [PaddleOCR](https://github.com/PaddlePaddle/PaddleOCR/blob/release/2.0/doc/doc_en/inference_en.md#convert-detection-model-to-inference-model) 開發套件中讀取文字檢測的模型,之後我們可以生成一個DJL `Predictor` 並將其命名為 `detector`.\n"
+ ]
+ },
+ {
+ "cell_type": "code",
+ "execution_count": null,
+ "metadata": {},
+ "outputs": [],
+ "source": [
+ "var criteria1 = Criteria.builder()\n",
+ " .optEngine(\"PaddlePaddle\")\n",
+ " .setTypes(Image.class, DetectedObjects.class)\n",
+ " .optModelUrls(\"https://resources.djl.ai/test-models/paddleOCR/mobile/det_db.zip\")\n",
+ " .optTranslator(new PpWordDetectionTranslator(new ConcurrentHashMap()))\n",
+ " .build();\n",
+ "var detectionModel = ModelZoo.loadModel(criteria1);\n",
+ "var detector = detectionModel.newPredictor();"
+ ]
+ },
+ {
+ "attachments": {},
+ "cell_type": "markdown",
+ "metadata": {},
+ "source": [
+ "接著我們檢測出圖片中的文字區塊,這個模型的原始輸出是含有標註所有文字區域的圖算法(Bitmap),我們可以利用`PpWordDetectionTranslator` 函式將圖算法的輸出轉成長方形的方框來裁剪圖片"
+ ]
+ },
+ {
+ "cell_type": "code",
+ "execution_count": null,
+ "metadata": {},
+ "outputs": [],
+ "source": [
+ "var detectedObj = detector.predict(img);\n",
+ "Image newImage = img.duplicate(Image.Type.TYPE_INT_ARGB);\n",
+ "newImage.drawBoundingBoxes(detectedObj);\n",
+ "newImage.getWrappedImage();"
+ ]
+ },
+ {
+ "attachments": {},
+ "cell_type": "markdown",
+ "metadata": {},
+ "source": [
+ "如上所示,所標註的文字區塊都非常窄,且沒有包住所有完整的文字區塊。讓我們嘗試使用`extendRect`函式來擴展文字框的長寬到需要的大小, 再利用 `getSubImage` 裁剪並擷取出文子區塊。"
+ ]
+ },
+ {
+ "cell_type": "code",
+ "execution_count": null,
+ "metadata": {},
+ "outputs": [],
+ "source": [
+ "Image getSubImage(Image img, BoundingBox box) {\n",
+ " Rectangle rect = box.getBounds();\n",
+ " double[] extended = extendRect(rect.getX(), rect.getY(), rect.getWidth(), rect.getHeight());\n",
+ " int width = img.getWidth();\n",
+ " int height = img.getHeight();\n",
+ " int[] recovered = {\n",
+ " (int) (extended[0] * width),\n",
+ " (int) (extended[1] * height),\n",
+ " (int) (extended[2] * width),\n",
+ " (int) (extended[3] * height)\n",
+ " };\n",
+ " return img.getSubimage(recovered[0], recovered[1], recovered[2], recovered[3]);\n",
+ "}\n",
+ "\n",
+ "double[] extendRect(double xmin, double ymin, double width, double height) {\n",
+ " double centerx = xmin + width / 2;\n",
+ " double centery = ymin + height / 2;\n",
+ " if (width > height) {\n",
+ " width += height * 2.0;\n",
+ " height *= 3.0;\n",
+ " } else {\n",
+ " height += width * 2.0;\n",
+ " width *= 3.0;\n",
+ " }\n",
+ " double newX = centerx - width / 2 < 0 ? 0 : centerx - width / 2;\n",
+ " double newY = centery - height / 2 < 0 ? 0 : centery - height / 2;\n",
+ " double newWidth = newX + width > 1 ? 1 - newX : width;\n",
+ " double newHeight = newY + height > 1 ? 1 - newY : height;\n",
+ " return new double[] {newX, newY, newWidth, newHeight};\n",
+ "}"
+ ]
+ },
+ {
+ "attachments": {},
+ "cell_type": "markdown",
+ "metadata": {},
+ "source": [
+ "讓我們輸出其中一個文字區塊"
+ ]
+ },
+ {
+ "cell_type": "code",
+ "execution_count": null,
+ "metadata": {},
+ "outputs": [],
+ "source": [
+ "List boxes = detectedObj.items();\n",
+ "var sample = getSubImage(img, boxes.get(5).getBoundingBox());\n",
+ "sample.getWrappedImage();"
+ ]
+ },
+ {
+ "attachments": {},
+ "cell_type": "markdown",
+ "metadata": {},
+ "source": [
+ "## 文字角度檢測\n",
+ "我們從 [PaddleOCR](https://github.com/PaddlePaddle/PaddleOCR/blob/release/2.0/doc/doc_en/inference_en.md#convert-angle-classification-model-to-inference-model) 輸出這個模型並確認圖片及文字是否需要旋轉。以下的代碼會讀入這個模型並生成a `rotateClassifier` 子類別"
+ ]
+ },
+ {
+ "cell_type": "code",
+ "execution_count": null,
+ "metadata": {},
+ "outputs": [],
+ "source": [
+ "var criteria2 = Criteria.builder()\n",
+ " .optEngine(\"PaddlePaddle\")\n",
+ " .setTypes(Image.class, Classifications.class)\n",
+ " .optModelUrls(\"https://resources.djl.ai/test-models/paddleOCR/mobile/cls.zip\")\n",
+ " .optTranslator(new PpWordRotateTranslator())\n",
+ " .build();\n",
+ "var rotateModel = ModelZoo.loadModel(criteria2);\n",
+ "var rotateClassifier = rotateModel.newPredictor();"
+ ]
+ },
+ {
+ "attachments": {},
+ "cell_type": "markdown",
+ "metadata": {},
+ "source": [
+ "## 文字識別\n",
+ "\n",
+ "我們從 [PaddleOCR](https://github.com/PaddlePaddle/PaddleOCR/blob/release/2.0/doc/doc_en/inference_en.md#convert-recognition-model-to-inference-model) 輸出這個模型並識別圖片中的文字, 我們一樣仿造上述的步驟讀取這個模型\n"
+ ]
+ },
+ {
+ "cell_type": "code",
+ "execution_count": null,
+ "metadata": {},
+ "outputs": [],
+ "source": [
+ "var criteria3 = Criteria.builder()\n",
+ " .optEngine(\"PaddlePaddle\")\n",
+ " .setTypes(Image.class, String.class)\n",
+ " .optModelUrls(\"https://resources.djl.ai/test-models/paddleOCR/mobile/rec_crnn.zip\")\n",
+ " .optTranslator(new PpWordRecognitionTranslator())\n",
+ " .build();\n",
+ "var recognitionModel = ModelZoo.loadModel(criteria3);\n",
+ "var recognizer = recognitionModel.newPredictor();"
+ ]
+ },
+ {
+ "attachments": {},
+ "cell_type": "markdown",
+ "metadata": {},
+ "source": [
+ "接著我們可以試著套用這兩個模型在先前剪裁好的文字區塊上"
+ ]
+ },
+ {
+ "cell_type": "code",
+ "execution_count": null,
+ "metadata": {},
+ "outputs": [],
+ "source": [
+ "System.out.println(rotateClassifier.predict(sample));\n",
+ "recognizer.predict(sample);"
+ ]
+ },
+ {
+ "attachments": {},
+ "cell_type": "markdown",
+ "metadata": {},
+ "source": [
+ "最後我們把這些模型串連在一起並套用在整張圖片上看看結果會如何。DJL提供了豐富的影像工具包讓你可以從圖片中擷取出文字並且完美呈現"
+ ]
+ },
+ {
+ "cell_type": "code",
+ "execution_count": null,
+ "metadata": {},
+ "outputs": [],
+ "source": [
+ "Image rotateImg(Image image) {\n",
+ " try (NDManager manager = NDManager.newBaseManager()) {\n",
+ " NDArray rotated = NDImageUtils.rotate90(image.toNDArray(manager), 1);\n",
+ " return ImageFactory.getInstance().fromNDArray(rotated);\n",
+ " }\n",
+ "}\n",
+ "\n",
+ "List names = new ArrayList<>();\n",
+ "List prob = new ArrayList<>();\n",
+ "List rect = new ArrayList<>();\n",
+ "\n",
+ "for (int i = 0; i < boxes.size(); i++) {\n",
+ " Image subImg = getSubImage(img, boxes.get(i).getBoundingBox());\n",
+ " if (subImg.getHeight() * 1.0 / subImg.getWidth() > 1.5) {\n",
+ " subImg = rotateImg(subImg);\n",
+ " }\n",
+ " Classifications.Classification result = rotateClassifier.predict(subImg).best();\n",
+ " if (\"Rotate\".equals(result.getClassName()) && result.getProbability() > 0.8) {\n",
+ " subImg = rotateImg(subImg);\n",
+ " }\n",
+ " String name = recognizer.predict(subImg);\n",
+ " names.add(name);\n",
+ " prob.add(-1.0);\n",
+ " rect.add(boxes.get(i).getBoundingBox());\n",
+ "}\n",
+ "newImage.drawBoundingBoxes(new DetectedObjects(names, prob, rect));\n",
+ "newImage.getWrappedImage();"
+ ]
+ }
+ ],
+ "metadata": {
+ "kernelspec": {
+ "display_name": "Java",
+ "language": "java",
+ "name": "java"
+ },
+ "language_info": {
+ "codemirror_mode": "java",
+ "file_extension": ".jshell",
+ "mimetype": "text/x-java-source",
+ "name": "Java",
+ "pygments_lexer": "java",
+ "version": "11.0.5+10-LTS"
+ }
+ },
+ "nbformat": 4,
+ "nbformat_minor": 4
+}
diff --git a/model-zoo/src/main/java/ai/djl/basicmodelzoo/cv/object_detection/ssd/SingleShotDetection.java b/model-zoo/src/main/java/ai/djl/basicmodelzoo/cv/object_detection/ssd/SingleShotDetection.java
index 4d9486c70cd..430c1aa66aa 100644
--- a/model-zoo/src/main/java/ai/djl/basicmodelzoo/cv/object_detection/ssd/SingleShotDetection.java
+++ b/model-zoo/src/main/java/ai/djl/basicmodelzoo/cv/object_detection/ssd/SingleShotDetection.java
@@ -111,46 +111,48 @@ private NDArray concatPredictions(NDList output) {
/** {@inheritDoc} */
@Override
- public Shape[] getOutputShapes(NDManager manager, Shape[] inputShapes) {
- // TODO: output shape is wrong
- Shape[] childInputShapes = inputShapes;
- Shape[] anchorShapes = new Shape[features.size()];
- Shape[] classPredictionShapes = new Shape[features.size()];
- Shape[] anchorPredictionShapes = new Shape[features.size()];
- for (int i = 0; i < features.size(); i++) {
- childInputShapes = features.get(i).getOutputShapes(manager, childInputShapes);
- anchorShapes[i] =
- multiBoxPriors
- .get(i)
- .generateAnchorBoxes(manager.ones(childInputShapes[0]))
- .getShape();
- classPredictionShapes[i] =
- classPredictionBlocks.get(i).getOutputShapes(manager, childInputShapes)[0];
- anchorPredictionShapes[i] =
- anchorPredictionBlocks.get(i).getOutputShapes(manager, childInputShapes)[0];
- }
- Shape anchorOutputShape = new Shape();
- for (Shape shape : anchorShapes) {
- anchorOutputShape = concatShape(anchorOutputShape, shape, 1);
- }
+ public Shape[] getOutputShapes(Shape[] inputShapes) {
+ try (NDManager manager = NDManager.newBaseManager()) {
+ // TODO: output shape is wrong
+ Shape[] childInputShapes = inputShapes;
+ Shape[] anchorShapes = new Shape[features.size()];
+ Shape[] classPredictionShapes = new Shape[features.size()];
+ Shape[] anchorPredictionShapes = new Shape[features.size()];
+ for (int i = 0; i < features.size(); i++) {
+ childInputShapes = features.get(i).getOutputShapes(childInputShapes);
+ anchorShapes[i] =
+ multiBoxPriors
+ .get(i)
+ .generateAnchorBoxes(manager.ones(childInputShapes[0]))
+ .getShape();
+ classPredictionShapes[i] =
+ classPredictionBlocks.get(i).getOutputShapes(childInputShapes)[0];
+ anchorPredictionShapes[i] =
+ anchorPredictionBlocks.get(i).getOutputShapes(childInputShapes)[0];
+ }
+ Shape anchorOutputShape = new Shape();
+ for (Shape shape : anchorShapes) {
+ anchorOutputShape = concatShape(anchorOutputShape, shape, 1);
+ }
- NDList classPredictions = new NDList();
- for (Shape shape : classPredictionShapes) {
- classPredictions.add(manager.ones(shape));
- }
- NDArray classPredictionOutput = concatPredictions(classPredictions);
- Shape classPredictionOutputShape =
- classPredictionOutput
- .reshape(classPredictionOutput.size(0), -1, numClasses + 1)
- .getShape();
- NDList anchorPredictions = new NDList();
- for (Shape shape : anchorPredictionShapes) {
- anchorPredictions.add(manager.ones(shape));
+ NDList classPredictions = new NDList();
+ for (Shape shape : classPredictionShapes) {
+ classPredictions.add(manager.ones(shape));
+ }
+ NDArray classPredictionOutput = concatPredictions(classPredictions);
+ Shape classPredictionOutputShape =
+ classPredictionOutput
+ .reshape(classPredictionOutput.size(0), -1, numClasses + 1)
+ .getShape();
+ NDList anchorPredictions = new NDList();
+ for (Shape shape : anchorPredictionShapes) {
+ anchorPredictions.add(manager.ones(shape));
+ }
+ Shape anchorPredictionOutputShape = concatPredictions(anchorPredictions).getShape();
+ return new Shape[] {
+ anchorOutputShape, classPredictionOutputShape, anchorPredictionOutputShape
+ };
}
- Shape anchorPredictionOutputShape = concatPredictions(anchorPredictions).getShape();
- return new Shape[] {
- anchorOutputShape, classPredictionOutputShape, anchorPredictionOutputShape
- };
}
private Shape concatShape(Shape shape, Shape concat, int axis) {
@@ -177,15 +179,15 @@ private Shape concatShape(Shape shape, Shape concat, int axis) {
/** {@inheritDoc} */
@Override
- public Shape[] initialize(NDManager manager, DataType dataType, Shape... inputShapes) {
+ public void initialize(NDManager manager, DataType dataType, Shape... inputShapes) {
beforeInitialize(inputShapes);
Shape[] shapes = inputShapes;
for (int i = 0; i < features.size(); i++) {
- shapes = features.get(i).initialize(manager, dataType, shapes);
+ features.get(i).initialize(manager, dataType, shapes);
+ shapes = features.get(i).getOutputShapes(shapes);
classPredictionBlocks.get(i).initialize(manager, dataType, shapes);
anchorPredictionBlocks.get(i).initialize(manager, dataType, shapes);
}
- return getOutputShapes(manager, inputShapes);
}
/** {@inheritDoc} */
diff --git a/mxnet/mxnet-engine/src/main/java/ai/djl/mxnet/engine/CachedOp.java b/mxnet/mxnet-engine/src/main/java/ai/djl/mxnet/engine/CachedOp.java
index 30460a25592..d13c75e684a 100644
--- a/mxnet/mxnet-engine/src/main/java/ai/djl/mxnet/engine/CachedOp.java
+++ b/mxnet/mxnet-engine/src/main/java/ai/djl/mxnet/engine/CachedOp.java
@@ -73,7 +73,7 @@ public CachedOp(
this.dataIndicesMap = dataIndices.toMap();
// holds all parameter and data NDArray values, final inputs to CachedOp
this.manager = manager;
- manager.attach(getUid(), this);
+ manager.attachInternal(getUid(), this);
}
/**
@@ -139,7 +139,7 @@ public NDList forward(ParameterStore parameterStore, NDList data, boolean traini
public void close() {
Pointer pointer = handle.getAndSet(null);
if (pointer != null) {
- manager.detach(getUid());
+ manager.detachInternal(getUid());
JnaUtils.freeCachedOp(pointer);
manager = null;
}
diff --git a/mxnet/mxnet-engine/src/main/java/ai/djl/mxnet/engine/MxEngine.java b/mxnet/mxnet-engine/src/main/java/ai/djl/mxnet/engine/MxEngine.java
index c0275e1bdf5..fb9c16df8ec 100644
--- a/mxnet/mxnet-engine/src/main/java/ai/djl/mxnet/engine/MxEngine.java
+++ b/mxnet/mxnet-engine/src/main/java/ai/djl/mxnet/engine/MxEngine.java
@@ -19,6 +19,7 @@
import ai.djl.mxnet.jna.JnaUtils;
import ai.djl.mxnet.jna.LibUtils;
import ai.djl.ndarray.NDManager;
+import ai.djl.nn.SymbolBlock;
import ai.djl.training.GradientCollector;
import ai.djl.training.LocalParameterServer;
import ai.djl.training.ParameterServer;
@@ -39,6 +40,7 @@
public final class MxEngine extends Engine {
public static final String ENGINE_NAME = "MXNet";
+ private static final String MXNET_EXTRA_LIBRARY_VERBOSE = "MXNET_EXTRA_LIBRARY_VERBOSE";
/** Constructs an MXNet Engine. */
private MxEngine() {}
@@ -55,6 +57,10 @@ static Engine newInstance() {
// load extra MXNet library
String paths = System.getenv("MXNET_EXTRA_LIBRARY_PATH");
+ boolean extraLibVerbose = false;
+ if (System.getenv().containsKey(MXNET_EXTRA_LIBRARY_VERBOSE)) {
+ extraLibVerbose = Boolean.parseBoolean(System.getenv(MXNET_EXTRA_LIBRARY_VERBOSE));
+ }
if (paths != null) {
String[] files = paths.split(",");
for (String file : files) {
@@ -62,7 +68,7 @@ static Engine newInstance() {
if (Files.notExists(path)) {
throw new FileNotFoundException("Extra Library not found: " + file);
}
- JnaUtils.loadLib(path.toAbsolutePath().toString(), 1);
+ JnaUtils.loadLib(path.toAbsolutePath().toString(), extraLibVerbose);
}
}
@@ -101,6 +107,12 @@ public boolean hasCapability(String capability) {
return JnaUtils.getFeatures().contains(capability);
}
+ /** {@inheritDoc} */
+ @Override
+ public SymbolBlock newSymbolBlock(NDManager manager) {
+ return new MxSymbolBlock(manager);
+ }
+
/** {@inheritDoc} */
@Override
public Model newModel(String name, Device device) {
diff --git a/mxnet/mxnet-engine/src/main/java/ai/djl/mxnet/engine/MxModel.java b/mxnet/mxnet-engine/src/main/java/ai/djl/mxnet/engine/MxModel.java
index ee8afeba541..5c675a9519c 100644
--- a/mxnet/mxnet-engine/src/main/java/ai/djl/mxnet/engine/MxModel.java
+++ b/mxnet/mxnet-engine/src/main/java/ai/djl/mxnet/engine/MxModel.java
@@ -24,6 +24,8 @@
import ai.djl.training.Trainer;
import ai.djl.training.TrainingConfig;
import ai.djl.training.initializer.Initializer;
+import ai.djl.util.Pair;
+import ai.djl.util.PairList;
import java.io.FileNotFoundException;
import java.io.IOException;
import java.nio.file.Files;
@@ -33,6 +35,7 @@
import java.util.List;
import java.util.Map;
import java.util.concurrent.ConcurrentHashMap;
+import java.util.function.Predicate;
import java.util.stream.Collectors;
import org.slf4j.Logger;
import org.slf4j.LoggerFactory;
@@ -122,12 +125,16 @@ public void load(Path modelPath, String prefix, Map options)
/** {@inheritDoc} */
@Override
public Trainer newTrainer(TrainingConfig trainingConfig) {
- Initializer initializer = trainingConfig.getInitializer();
+ PairList> initializer = trainingConfig.getInitializers();
if (block == null) {
throw new IllegalStateException(
"You must set a block for the model before creating a new trainer");
}
- block.setInitializer(initializer);
+ for (Pair> pair : initializer) {
+ if (pair.getKey() != null && pair.getValue() != null) {
+ block.setInitializer(pair.getKey(), pair.getValue());
+ }
+ }
return new Trainer(this, trainingConfig);
}
@@ -193,20 +200,4 @@ private void loadParameters(Path paramFile, Map options)
dataType = paramNDlist.head().getDataType();
logger.debug("MXNet Model {} ({}) loaded successfully.", paramFile, dataType);
}
-
- /** {@inheritDoc} */
- @Override
- public String toString() {
- StringBuilder sb = new StringBuilder(200);
- sb.append("Model (\n\tName: ").append(modelName);
- if (modelDir != null) {
- sb.append("\n\tModel location: ").append(modelDir.toAbsolutePath());
- }
- sb.append("\n\tData Type: ").append(dataType);
- for (Map.Entry entry : properties.entrySet()) {
- sb.append("\n\t").append(entry.getKey()).append(": ").append(entry.getValue());
- }
- sb.append("\n)");
- return sb.toString();
- }
}
diff --git a/mxnet/mxnet-engine/src/main/java/ai/djl/mxnet/engine/MxNDArray.java b/mxnet/mxnet-engine/src/main/java/ai/djl/mxnet/engine/MxNDArray.java
index e8ecde776a9..e48cce9af31 100644
--- a/mxnet/mxnet-engine/src/main/java/ai/djl/mxnet/engine/MxNDArray.java
+++ b/mxnet/mxnet-engine/src/main/java/ai/djl/mxnet/engine/MxNDArray.java
@@ -92,7 +92,7 @@ public class MxNDArray extends NativeResource implements LazyNDArray {
super(handle);
this.manager = manager;
mxNDArrayEx = new MxNDArrayEx(this);
- manager.attach(getUid(), this);
+ manager.attachInternal(getUid(), this);
}
/**
@@ -163,18 +163,25 @@ public SparseFormat getSparseFormat() {
/** {@inheritDoc} */
@Override
- public NDManager attach(NDManager manager) {
+ public void attach(NDManager manager) {
+ detach();
+ this.manager = (MxNDManager) manager;
+ manager.attachInternal(getUid(), this);
+ }
+
+ /** {@inheritDoc} */
+ @Override
+ public void tempAttach(NDManager manager) {
NDManager original = this.manager;
detach();
this.manager = (MxNDManager) manager;
- manager.attach(getUid(), this);
- return original;
+ manager.tempAttachInternal(original, getUid(), this);
}
/** {@inheritDoc} */
@Override
public void detach() {
- manager.detach(getUid());
+ manager.detachInternal(getUid());
manager = MxNDManager.getSystemManager();
}
@@ -268,14 +275,24 @@ public boolean hasGradient() {
return hasGradient;
}
+ /** {@inheritDoc} */
@Override
public NDArray stopGradient() {
return manager.invoke("stop_gradient", this, null);
}
+ /** {@inheritDoc} */
+ @Override
+ public String[] toStringArray() {
+ throw new UnsupportedOperationException("String NDArray is not supported!");
+ }
+
/** {@inheritDoc} */
@Override
public ByteBuffer toByteBuffer() {
+ if (getSparseFormat() != SparseFormat.DENSE) {
+ throw new IllegalStateException("Require Dense NDArray, actual " + getSparseFormat());
+ }
Shape sh = getShape();
DataType dType = getDataType();
long product = sh.size();
@@ -1602,7 +1619,7 @@ public void close() {
if (pointer != null) {
JnaUtils.waitToRead(pointer);
JnaUtils.freeNdArray(pointer);
- manager.detach(getUid());
+ manager.detachInternal(getUid());
manager = null;
}
}
diff --git a/mxnet/mxnet-engine/src/main/java/ai/djl/mxnet/engine/MxNDArrayEx.java b/mxnet/mxnet-engine/src/main/java/ai/djl/mxnet/engine/MxNDArrayEx.java
index 09f154e964d..5e9c32cf047 100644
--- a/mxnet/mxnet-engine/src/main/java/ai/djl/mxnet/engine/MxNDArrayEx.java
+++ b/mxnet/mxnet-engine/src/main/java/ai/djl/mxnet/engine/MxNDArrayEx.java
@@ -377,8 +377,7 @@ public void adadeltaUpdate(
// create a baseManager to close all intermediate NDArrays
try (NDManager subManager = NDManager.newBaseManager()) {
- List inputManagers = inputs.attach(subManager);
- List weightManagers = weights.attach(subManager);
+ subManager.tempAttachAll(inputs, weights);
// Preprocess Gradient
grad.muli(rescaleGrad);
@@ -394,10 +393,6 @@ public void adadeltaUpdate(
// Update weight
weight.subi(g);
-
- // attach back to their previous managers
- inputs.attach(inputManagers);
- weights.attach(weightManagers);
}
}
diff --git a/mxnet/mxnet-engine/src/main/java/ai/djl/mxnet/engine/MxNDManager.java b/mxnet/mxnet-engine/src/main/java/ai/djl/mxnet/engine/MxNDManager.java
index 1effa33d3a5..cb18c373c68 100644
--- a/mxnet/mxnet-engine/src/main/java/ai/djl/mxnet/engine/MxNDManager.java
+++ b/mxnet/mxnet-engine/src/main/java/ai/djl/mxnet/engine/MxNDManager.java
@@ -81,8 +81,8 @@ public MxNDArray create(Pointer handle) {
* @param fmt the sparse format to use
* @return the created array
*/
- public MxSparseNDArray create(Pointer handle, SparseFormat fmt) {
- return new MxSparseNDArray(this, handle, fmt);
+ public MxNDArray create(Pointer handle, SparseFormat fmt) {
+ return new MxNDArray(this, handle, fmt);
}
/** {@inheritDoc} */
@@ -97,7 +97,7 @@ public MxNDArray create(Shape shape, DataType dataType) {
/** {@inheritDoc} */
@Override
- public MxSparseNDArray createCSR(Buffer data, long[] indptr, long[] indices, Shape shape) {
+ public MxNDArray createCSR(Buffer data, long[] indptr, long[] indices, Shape shape) {
SparseFormat fmt = SparseFormat.CSR;
DataType dataType = DataType.fromBuffer(data);
MxNDArray indptrNd = create(new Shape(indptr.length), DataType.INT64);
@@ -113,7 +113,7 @@ public MxSparseNDArray createCSR(Buffer data, long[] indptr, long[] indices, Sha
new DataType[] {indptrNd.getDataType(), indicesNd.getDataType()},
new Shape[] {indptrNd.getShape(), indicesNd.getShape()},
false);
- MxSparseNDArray sparse = create(handle, fmt);
+ MxNDArray sparse = create(handle, fmt);
MxNDArray dataNd = create(new Shape(data.remaining()), dataType);
dataNd.set(data);
JnaUtils.ndArraySyncCopyFromNdArray(sparse, dataNd, -1);
@@ -124,8 +124,7 @@ public MxSparseNDArray createCSR(Buffer data, long[] indptr, long[] indices, Sha
/** {@inheritDoc} */
@Override
- public MxSparseNDArray createRowSparse(
- Buffer data, Shape dataShape, long[] indices, Shape shape) {
+ public MxNDArray createRowSparse(Buffer data, Shape dataShape, long[] indices, Shape shape) {
SparseFormat fmt = SparseFormat.ROW_SPARSE;
DataType dataType = DataType.fromBuffer(data);
MxNDArray indicesNd = create(new Shape(indices.length), DataType.INT64);
@@ -139,7 +138,7 @@ public MxSparseNDArray createRowSparse(
new DataType[] {indicesNd.getDataType()},
new Shape[] {indicesNd.getShape()},
false);
- MxSparseNDArray sparse = create(handle, fmt);
+ MxNDArray sparse = create(handle, fmt);
MxNDArray dataNd = create(dataShape, dataType);
dataNd.set(data);
JnaUtils.ndArraySyncCopyFromNdArray(sparse, dataNd, -1);
@@ -272,7 +271,7 @@ public NDArray randomMultinomial(int n, NDArray pValues) {
@Override
public MxNDManager newSubManager(Device dev) {
MxNDManager manager = new MxNDManager(this, dev, version);
- attach(manager.uid, manager);
+ attachInternal(manager.uid, manager);
return manager;
}
@@ -386,11 +385,11 @@ private static final class SystemManager extends MxNDManager {
/** {@inheritDoc} */
@Override
- public void attach(String resourceId, AutoCloseable resource) {}
+ public void attachInternal(String resourceId, AutoCloseable resource) {}
/** {@inheritDoc} */
@Override
- public void detach(String resourceId) {}
+ public void detachInternal(String resourceId) {}
/** {@inheritDoc} */
@Override
diff --git a/mxnet/mxnet-engine/src/main/java/ai/djl/mxnet/engine/MxSparseNDArray.java b/mxnet/mxnet-engine/src/main/java/ai/djl/mxnet/engine/MxSparseNDArray.java
deleted file mode 100644
index 942591ceafe..00000000000
--- a/mxnet/mxnet-engine/src/main/java/ai/djl/mxnet/engine/MxSparseNDArray.java
+++ /dev/null
@@ -1,62 +0,0 @@
-/*
- * Copyright 2019 Amazon.com, Inc. or its affiliates. All Rights Reserved.
- *
- * Licensed under the Apache License, Version 2.0 (the "License"). You may not use this file except in compliance
- * with the License. A copy of the License is located at
- *
- * http://aws.amazon.com/apache2.0/
- *
- * or in the "license" file accompanying this file. This file is distributed on an "AS IS" BASIS, WITHOUT WARRANTIES
- * OR CONDITIONS OF ANY KIND, either express or implied. See the License for the specific language governing permissions
- * and limitations under the License.
- */
-package ai.djl.mxnet.engine;
-
-import ai.djl.ndarray.NDArray;
-import ai.djl.ndarray.index.NDIndex;
-import ai.djl.ndarray.types.SparseFormat;
-import com.sun.jna.Pointer;
-import java.nio.Buffer;
-import java.nio.ByteBuffer;
-
-/**
- * {@code MxSparseNDArray} is an instance of {@link MxNDArray} and {@link NDArray} for sparse
- * NDArrays.
- *
- *
{@code MxSparseNDArray}s are created automatically when the Engine creates Arrays that are
- * sparse. They can be created deliberately by specifying the {@link SparseFormat}. Some operations
- * may not be supported with Sparse NDArrays in MXNet.
- *
- * @see SparseFormat
- */
-public class MxSparseNDArray extends MxNDArray {
-
- /**
- * Constructs a {@code MxSparseNDArray} for the given data.
- *
- * @param manager the manager to attach the array to
- * @param handle the pointer to the native memory of the MXNDArray
- * @param fmt the sparse format
- */
- MxSparseNDArray(MxNDManager manager, Pointer handle, SparseFormat fmt) {
- super(manager, handle, fmt);
- }
-
- /** {@inheritDoc} */
- @Override
- public void set(Buffer data) {
- throw new IllegalStateException("Unsupported operation for Sparse");
- }
-
- /** {@inheritDoc} */
- @Override
- public NDArray get(NDIndex index) {
- return toDense().get(index);
- }
-
- /** {@inheritDoc} */
- @Override
- public ByteBuffer toByteBuffer() {
- return toDense().toByteBuffer();
- }
-}
diff --git a/mxnet/mxnet-engine/src/main/java/ai/djl/mxnet/engine/MxSymbolBlock.java b/mxnet/mxnet-engine/src/main/java/ai/djl/mxnet/engine/MxSymbolBlock.java
index ffc04996a4b..b2c8d6cdb67 100644
--- a/mxnet/mxnet-engine/src/main/java/ai/djl/mxnet/engine/MxSymbolBlock.java
+++ b/mxnet/mxnet-engine/src/main/java/ai/djl/mxnet/engine/MxSymbolBlock.java
@@ -21,7 +21,6 @@
import ai.djl.ndarray.types.Shape;
import ai.djl.nn.AbstractSymbolBlock;
import ai.djl.nn.Parameter;
-import ai.djl.nn.ParameterType;
import ai.djl.nn.SymbolBlock;
import ai.djl.training.ParameterStore;
import ai.djl.util.PairList;
@@ -201,7 +200,7 @@ protected NDList forwardInternal(
/** {@inheritDoc} */
@Override
- public Shape[] getOutputShapes(NDManager manager, Shape[] inputShapes) {
+ public Shape[] getOutputShapes(Shape[] inputShapes) {
if (outputShapes == null) {
String[] outputNames = symbol.getOutputNames();
outputShapes = new Shape[outputNames.length];
@@ -232,9 +231,7 @@ public void removeLastBlock() {
}
}
- /** {@inheritDoc} */
- @Override
- public Shape getParameterShape(String name, Shape[] inputShapes) {
+ private Shape getParameterShape(String name, Shape[] inputShapes) {
if (paramShapes == null) {
PairList pairs = new PairList<>();
for (int i = 0; i < inputNames.size(); i++) {
@@ -263,10 +260,8 @@ public void saveParameters(DataOutputStream os) throws IOException {
for (String name : inputNames) {
os.writeUTF(name);
}
- for (Parameter parameter : parameters.values()) {
- if (!inputNames.contains(parameter.getName())) {
- parameter.save(os);
- }
+ for (Parameter parameter : mxNetParams) {
+ parameter.save(os);
}
}
@@ -289,21 +284,20 @@ public void loadParameters(NDManager manager, DataInputStream is)
throw new MalformedModelException("InputStream ends at symbol loading!");
}
// init block only if it is not set
- if (symbol == null) {
- symbol =
- Symbol.loadJson(
- (MxNDManager) manager, new String(bytes, StandardCharsets.UTF_8));
- initBlock();
- }
+ symbol =
+ Symbol.loadJson(
+ (MxNDManager) manager, new String(bytes, StandardCharsets.UTF_8));
+ initBlock();
}
int size = is.readInt();
for (int i = 0; i < size; ++i) {
inputNames.add(is.readUTF());
}
- for (Parameter parameter : parameters.values()) {
+ for (Parameter parameter : mxNetParams) {
parameter.load(this.manager, is);
}
+ setInputNames(inputNames);
}
private void initBlock() {
@@ -314,25 +308,32 @@ private void initBlock() {
Set auxNameSet = new HashSet<>(Arrays.asList(symbol.getAuxNames()));
for (String name : allNames) {
- ParameterType type = inferType(name);
+ Parameter.Type type = inferType(name);
boolean requireGrad = !auxNameSet.contains(name);
- mxNetParams.add(new Parameter(name, this, type, requireGrad));
+ mxNetParams.add(
+ Parameter.builder()
+ .setName(name)
+ .setType(type)
+ .optRequiresGrad(requireGrad)
+ .build());
}
first = true;
}
- private static ParameterType inferType(String name) {
+ private static Parameter.Type inferType(String name) {
if (name.endsWith("bias")) {
- return ParameterType.BIAS;
+ return Parameter.Type.BIAS;
} else if (name.endsWith("gamma")) {
- return ParameterType.GAMMA;
+ return Parameter.Type.GAMMA;
} else if (name.endsWith("beta")) {
- return ParameterType.BETA;
+ return Parameter.Type.BETA;
} else if (name.endsWith("moving_mean") || name.endsWith("running_mean")) {
- return ParameterType.RUNNING_MEAN;
+ return Parameter.Type.RUNNING_MEAN;
} else if (name.endsWith("moving_var") || name.endsWith("running_var")) {
- return ParameterType.RUNNING_VAR;
+ return Parameter.Type.RUNNING_VAR;
+ } else if (name.endsWith("weight")) {
+ return Parameter.Type.WEIGHT;
}
- return ParameterType.OTHER;
+ return Parameter.Type.OTHER;
}
}
diff --git a/mxnet/mxnet-engine/src/main/java/ai/djl/mxnet/engine/Symbol.java b/mxnet/mxnet-engine/src/main/java/ai/djl/mxnet/engine/Symbol.java
index 9bd825b3c7d..8fcc92e5061 100644
--- a/mxnet/mxnet-engine/src/main/java/ai/djl/mxnet/engine/Symbol.java
+++ b/mxnet/mxnet-engine/src/main/java/ai/djl/mxnet/engine/Symbol.java
@@ -52,7 +52,7 @@ public class Symbol extends NativeResource {
Symbol(MxNDManager manager, Pointer pointer) {
super(pointer);
this.manager = manager;
- manager.attach(getUid(), this);
+ manager.attachInternal(getUid(), this);
// argParams = JnaUtils.listSymbolArguments(getHandle());
// auxParams = JnaUtils.listSymbolAuxiliaryStates(getHandle());
}
@@ -311,7 +311,7 @@ public String toString() {
public void close() {
Pointer pointer = handle.getAndSet(null);
if (pointer != null) {
- manager.detach(getUid());
+ manager.detachInternal(getUid());
JnaUtils.freeSymbol(pointer);
manager = null;
}
diff --git a/mxnet/mxnet-engine/src/main/java/ai/djl/mxnet/jna/JnaUtils.java b/mxnet/mxnet-engine/src/main/java/ai/djl/mxnet/jna/JnaUtils.java
index 0527049a25a..43daa3e388f 100644
--- a/mxnet/mxnet-engine/src/main/java/ai/djl/mxnet/jna/JnaUtils.java
+++ b/mxnet/mxnet-engine/src/main/java/ai/djl/mxnet/jna/JnaUtils.java
@@ -1254,8 +1254,9 @@ public static List> inferShape(Symbol symbol, PairListcom.microsoft.onnxruntimeonnxruntime_gpu
- 1.5.2
+ 1.7.0runtime
```
@@ -83,5 +83,5 @@ Gradle:
implementation("ai.djl.onnxruntime:onnxruntime-engine:0.10.0") {
exclude group: "com.microsoft.onnxruntime", module: "onnxruntime"
}
- implementation "com.microsoft.onnxruntime:onnxruntime_gpu:1.5.2"
+ implementation "com.microsoft.onnxruntime:onnxruntime_gpu:1.7.0"
```
diff --git a/onnxruntime/onnxruntime-engine/src/main/java/ai/djl/onnxruntime/engine/OrtEngine.java b/onnxruntime/onnxruntime-engine/src/main/java/ai/djl/onnxruntime/engine/OrtEngine.java
index 9b95bc9249a..0be98f3f722 100644
--- a/onnxruntime/onnxruntime-engine/src/main/java/ai/djl/onnxruntime/engine/OrtEngine.java
+++ b/onnxruntime/onnxruntime-engine/src/main/java/ai/djl/onnxruntime/engine/OrtEngine.java
@@ -17,6 +17,7 @@
import ai.djl.Model;
import ai.djl.engine.Engine;
import ai.djl.ndarray.NDManager;
+import ai.djl.nn.SymbolBlock;
import ai.djl.training.GradientCollector;
import ai.onnxruntime.OrtEnvironment;
@@ -56,6 +57,9 @@ public int getRank() {
}
private Engine getAlternativeEngine() {
+ if (Boolean.getBoolean("ai.djl.onnx.disable_alternative")) {
+ return null;
+ }
if (alternativeEngine == null) {
Engine engine = Engine.getInstance();
if (engine.getRank() < getRank()) {
@@ -69,7 +73,7 @@ private Engine getAlternativeEngine() {
/** {@inheritDoc} */
@Override
public String getVersion() {
- return "1.5.2";
+ return "1.7.0";
}
/** {@inheritDoc} */
@@ -85,6 +89,12 @@ public Model newModel(String name, Device device) {
return new OrtModel(name, newBaseManager(device), env);
}
+ /** {@inheritDoc} */
+ @Override
+ public SymbolBlock newSymbolBlock(NDManager manager) {
+ throw new UnsupportedOperationException("ONNXRuntime does not support empty SymbolBlock");
+ }
+
/** {@inheritDoc} */
@Override
public NDManager newBaseManager() {
diff --git a/onnxruntime/onnxruntime-engine/src/main/java/ai/djl/onnxruntime/engine/OrtNDArray.java b/onnxruntime/onnxruntime-engine/src/main/java/ai/djl/onnxruntime/engine/OrtNDArray.java
index 42556a788ca..997f6c29162 100644
--- a/onnxruntime/onnxruntime-engine/src/main/java/ai/djl/onnxruntime/engine/OrtNDArray.java
+++ b/onnxruntime/onnxruntime-engine/src/main/java/ai/djl/onnxruntime/engine/OrtNDArray.java
@@ -13,12 +13,16 @@
package ai.djl.onnxruntime.engine;
import ai.djl.Device;
+import ai.djl.engine.EngineException;
import ai.djl.ndarray.NDArray;
import ai.djl.ndarray.NDArrayAdapter;
import ai.djl.ndarray.NDManager;
import ai.djl.ndarray.types.DataType;
import ai.djl.ndarray.types.Shape;
import ai.onnxruntime.OnnxTensor;
+import ai.onnxruntime.OrtException;
+import java.nio.ByteBuffer;
+import java.nio.ByteOrder;
import java.util.Arrays;
import java.util.UUID;
@@ -44,7 +48,7 @@ public class OrtNDArray implements NDArrayAdapter {
this.manager = manager;
this.tensor = tensor;
uid = UUID.randomUUID().toString();
- manager.attach(uid, this);
+ manager.attachInternal(uid, this);
}
OnnxTensor getTensor() {
@@ -102,35 +106,57 @@ public Shape getShape() {
/** {@inheritDoc} */
@Override
- public NDManager attach(NDManager manager) {
+ public void attach(NDManager manager) {
+ detach();
+ this.manager = (OrtNDManager) manager;
+ manager.attachInternal(getUid(), this);
+ }
+
+ /** {@inheritDoc} */
+ @Override
+ public void tempAttach(NDManager manager) {
detach();
NDManager original = this.manager;
this.manager = (OrtNDManager) manager;
- manager.attach(getUid(), this);
- return original;
+ manager.tempAttachInternal(original, getUid(), this);
}
/** {@inheritDoc} */
@Override
public void detach() {
- manager.detach(getUid());
+ manager.detachInternal(getUid());
manager = OrtNDManager.getSystemManager();
}
+ /** {@inheritDoc} */
+ @Override
+ public String[] toStringArray() {
+ try {
+ return (String[]) tensor.getValue();
+ } catch (OrtException e) {
+ throw new EngineException(e);
+ }
+ }
+
+ /** {@inheritDoc} */
+ @Override
+ public ByteBuffer toByteBuffer() {
+ return tensor.getByteBuffer().order(ByteOrder.nativeOrder());
+ }
+
/** {@inheritDoc} */
@Override
public String toString() {
if (isClosed) {
return "This array is already closed";
}
- return "ND: "
- + getShape()
- + ' '
- + getDevice()
- + ' '
- + getDataType()
- + '\n'
- + Arrays.toString(toArray());
+ String arrStr;
+ if (getDataType() == DataType.STRING) {
+ arrStr = Arrays.toString(toStringArray());
+ } else {
+ arrStr = Arrays.toString(toArray());
+ }
+ return "ND: " + getShape() + ' ' + getDevice() + ' ' + getDataType() + '\n' + arrStr;
}
/** {@inheritDoc} */
diff --git a/onnxruntime/onnxruntime-engine/src/main/java/ai/djl/onnxruntime/engine/OrtNDManager.java b/onnxruntime/onnxruntime-engine/src/main/java/ai/djl/onnxruntime/engine/OrtNDManager.java
index ee427153209..4120b64df40 100644
--- a/onnxruntime/onnxruntime-engine/src/main/java/ai/djl/onnxruntime/engine/OrtNDManager.java
+++ b/onnxruntime/onnxruntime-engine/src/main/java/ai/djl/onnxruntime/engine/OrtNDManager.java
@@ -63,6 +63,33 @@ public OrtNDArray create(Buffer data, Shape shape, DataType dataType) {
}
}
+ /** {@inheritDoc} */
+ @Override
+ public NDArray create(String data) {
+ return create(new String[] {data}, new Shape(1));
+ }
+
+ /** {@inheritDoc} */
+ @Override
+ public NDArray create(String[] data) {
+ return create(data, new Shape(data.length));
+ }
+
+ /**
+ * Create A String tensor based on the provided shape.
+ *
+ * @param data the flattened String array
+ * @param shape the shape of the String NDArray
+ * @return a new instance of {@link NDArray}
+ */
+ public NDArray create(String[] data, Shape shape) {
+ try {
+ return new OrtNDArray(this, OrtUtils.toTensor(env, data, shape));
+ } catch (OrtException e) {
+ throw new EngineException(e);
+ }
+ }
+
/** {@inheritDoc} */
@Override
public NDArray zeros(Shape shape, DataType dataType) {
@@ -109,7 +136,7 @@ public NDArray ones(Shape shape, DataType dataType) {
@Override
public OrtNDManager newSubManager(Device device) {
OrtNDManager manager = new OrtNDManager(this, device, env);
- attach(manager.uid, manager);
+ attachInternal(manager.uid, manager);
return manager;
}
@@ -128,11 +155,11 @@ private static final class SystemManager extends OrtNDManager {
/** {@inheritDoc} */
@Override
- public void attach(String resourceId, AutoCloseable resource) {}
+ public void attachInternal(String resourceId, AutoCloseable resource) {}
/** {@inheritDoc} */
@Override
- public void detach(String resourceId) {}
+ public void detachInternal(String resourceId) {}
/** {@inheritDoc} */
@Override
diff --git a/onnxruntime/onnxruntime-engine/src/main/java/ai/djl/onnxruntime/engine/OrtUtils.java b/onnxruntime/onnxruntime-engine/src/main/java/ai/djl/onnxruntime/engine/OrtUtils.java
index 44d06580bb6..90d8e200e98 100644
--- a/onnxruntime/onnxruntime-engine/src/main/java/ai/djl/onnxruntime/engine/OrtUtils.java
+++ b/onnxruntime/onnxruntime-engine/src/main/java/ai/djl/onnxruntime/engine/OrtUtils.java
@@ -63,6 +63,12 @@ public static OnnxTensor toTensor(
}
}
+ public static OnnxTensor toTensor(OrtEnvironment env, String[] inputs, Shape shape)
+ throws OrtException {
+ long[] sh = shape.getShape();
+ return OnnxTensor.createTensor(env, inputs, sh);
+ }
+
public static NDArray toNDArray(NDManager manager, OnnxTensor tensor) {
if (manager instanceof OrtNDManager) {
return ((OrtNDManager) manager).create(tensor);
@@ -92,6 +98,8 @@ public static DataType toDataType(OnnxJavaType javaType) {
return DataType.BOOLEAN;
case UNKNOWN:
return DataType.UNKNOWN;
+ case STRING:
+ return DataType.STRING;
default:
throw new UnsupportedOperationException("type is not supported: " + javaType);
}
diff --git a/onnxruntime/onnxruntime-engine/src/main/java/ai/djl/onnxruntime/zoo/OrtModelZoo.java b/onnxruntime/onnxruntime-engine/src/main/java/ai/djl/onnxruntime/zoo/OrtModelZoo.java
index 0c5dfa5ec9e..3dadcc532af 100644
--- a/onnxruntime/onnxruntime-engine/src/main/java/ai/djl/onnxruntime/zoo/OrtModelZoo.java
+++ b/onnxruntime/onnxruntime-engine/src/main/java/ai/djl/onnxruntime/zoo/OrtModelZoo.java
@@ -13,7 +13,7 @@
package ai.djl.onnxruntime.zoo;
import ai.djl.onnxruntime.engine.OrtEngine;
-import ai.djl.onnxruntime.zoo.tabular.randomforest.IrisClassificationModelLoader;
+import ai.djl.onnxruntime.zoo.tabular.softmax_regression.IrisClassificationModelLoader;
import ai.djl.repository.Repository;
import ai.djl.repository.zoo.ModelZoo;
import java.util.Collections;
diff --git a/onnxruntime/onnxruntime-engine/src/main/java/ai/djl/onnxruntime/zoo/tabular/randomforest/IrisClassificationModelLoader.java b/onnxruntime/onnxruntime-engine/src/main/java/ai/djl/onnxruntime/zoo/tabular/softmax_regression/IrisClassificationModelLoader.java
similarity index 96%
rename from onnxruntime/onnxruntime-engine/src/main/java/ai/djl/onnxruntime/zoo/tabular/randomforest/IrisClassificationModelLoader.java
rename to onnxruntime/onnxruntime-engine/src/main/java/ai/djl/onnxruntime/zoo/tabular/softmax_regression/IrisClassificationModelLoader.java
index 67109f547ea..8b48fbb393b 100644
--- a/onnxruntime/onnxruntime-engine/src/main/java/ai/djl/onnxruntime/zoo/tabular/randomforest/IrisClassificationModelLoader.java
+++ b/onnxruntime/onnxruntime-engine/src/main/java/ai/djl/onnxruntime/zoo/tabular/softmax_regression/IrisClassificationModelLoader.java
@@ -10,9 +10,10 @@
* OR CONDITIONS OF ANY KIND, either express or implied. See the License for the specific language governing permissions
* and limitations under the License.
*/
-package ai.djl.onnxruntime.zoo.tabular.randomforest;
+package ai.djl.onnxruntime.zoo.tabular.softmax_regression;
import ai.djl.Application;
+import ai.djl.Application.Tabular;
import ai.djl.MalformedModelException;
import ai.djl.Model;
import ai.djl.modality.Classifications;
@@ -39,7 +40,7 @@
/** Model loader for onnx iris_flowers models. */
public class IrisClassificationModelLoader extends BaseModelLoader {
- private static final Application APPLICATION = Application.Tabular.RANDOM_FOREST;
+ private static final Application APPLICATION = Tabular.SOFTMAX_REGRESSION;
private static final String GROUP_ID = OrtModelZoo.GROUP_ID;
private static final String ARTIFACT_ID = "iris_flowers";
private static final String VERSION = "0.0.1";
diff --git a/onnxruntime/onnxruntime-engine/src/main/java/ai/djl/onnxruntime/zoo/tabular/randomforest/IrisFlower.java b/onnxruntime/onnxruntime-engine/src/main/java/ai/djl/onnxruntime/zoo/tabular/softmax_regression/IrisFlower.java
similarity index 96%
rename from onnxruntime/onnxruntime-engine/src/main/java/ai/djl/onnxruntime/zoo/tabular/randomforest/IrisFlower.java
rename to onnxruntime/onnxruntime-engine/src/main/java/ai/djl/onnxruntime/zoo/tabular/softmax_regression/IrisFlower.java
index ba77ec71b96..27263e520f2 100644
--- a/onnxruntime/onnxruntime-engine/src/main/java/ai/djl/onnxruntime/zoo/tabular/randomforest/IrisFlower.java
+++ b/onnxruntime/onnxruntime-engine/src/main/java/ai/djl/onnxruntime/zoo/tabular/softmax_regression/IrisFlower.java
@@ -10,7 +10,7 @@
* OR CONDITIONS OF ANY KIND, either express or implied. See the License for the specific language governing permissions
* and limitations under the License.
*/
-package ai.djl.onnxruntime.zoo.tabular.randomforest;
+package ai.djl.onnxruntime.zoo.tabular.softmax_regression;
/** A class holds the iris flower features. */
public class IrisFlower {
diff --git a/onnxruntime/onnxruntime-engine/src/main/java/ai/djl/onnxruntime/zoo/tabular/randomforest/package-info.java b/onnxruntime/onnxruntime-engine/src/main/java/ai/djl/onnxruntime/zoo/tabular/softmax_regression/package-info.java
similarity index 92%
rename from onnxruntime/onnxruntime-engine/src/main/java/ai/djl/onnxruntime/zoo/tabular/randomforest/package-info.java
rename to onnxruntime/onnxruntime-engine/src/main/java/ai/djl/onnxruntime/zoo/tabular/softmax_regression/package-info.java
index 7759b6962de..d6e6aee3bf3 100644
--- a/onnxruntime/onnxruntime-engine/src/main/java/ai/djl/onnxruntime/zoo/tabular/randomforest/package-info.java
+++ b/onnxruntime/onnxruntime-engine/src/main/java/ai/djl/onnxruntime/zoo/tabular/softmax_regression/package-info.java
@@ -14,4 +14,4 @@
/**
* Contains classes for the classification models in the {@link ai.djl.onnxruntime.zoo.OrtModelZoo}.
*/
-package ai.djl.onnxruntime.zoo.tabular.randomforest;
+package ai.djl.onnxruntime.zoo.tabular.softmax_regression;
diff --git a/onnxruntime/onnxruntime-engine/src/test/java/ai/djl/onnxruntime/engine/OrtTest.java b/onnxruntime/onnxruntime-engine/src/test/java/ai/djl/onnxruntime/engine/OrtTest.java
index d867cbb324b..67369346487 100644
--- a/onnxruntime/onnxruntime-engine/src/test/java/ai/djl/onnxruntime/engine/OrtTest.java
+++ b/onnxruntime/onnxruntime-engine/src/test/java/ai/djl/onnxruntime/engine/OrtTest.java
@@ -12,12 +12,17 @@
*/
package ai.djl.onnxruntime.engine;
+import ai.djl.MalformedModelException;
import ai.djl.Model;
import ai.djl.ModelException;
import ai.djl.inference.Predictor;
import ai.djl.modality.Classifications;
-import ai.djl.onnxruntime.zoo.tabular.randomforest.IrisFlower;
+import ai.djl.ndarray.NDArray;
+import ai.djl.ndarray.NDList;
+import ai.djl.ndarray.types.Shape;
+import ai.djl.onnxruntime.zoo.tabular.softmax_regression.IrisFlower;
import ai.djl.repository.zoo.Criteria;
+import ai.djl.repository.zoo.ModelNotFoundException;
import ai.djl.repository.zoo.ModelZoo;
import ai.djl.repository.zoo.ZooModel;
import ai.djl.translate.TranslateException;
@@ -63,4 +68,30 @@ public void testOrt() throws TranslateException, ModelException, IOException {
throw new SkipException("Ignore missing libgomp.so.1 error.");
}
}
+
+ @Test
+ public void testStringTensor()
+ throws MalformedModelException, ModelNotFoundException, IOException,
+ TranslateException {
+ System.setProperty("ai.djl.onnx.disable_alternative", "true");
+ Criteria criteria =
+ Criteria.builder()
+ .setTypes(NDList.class, NDList.class)
+ .optEngine("OnnxRuntime")
+ .optModelUrls(
+ "https://resources.djl.ai/test-models/onnxruntime/pipeline_tfidf.zip")
+ .build();
+ try (ZooModel model = ModelZoo.loadModel(criteria);
+ Predictor predictor = model.newPredictor()) {
+ OrtNDManager manager = (OrtNDManager) model.getNDManager();
+ NDArray stringNd =
+ manager.create(
+ new String[] {" Re: Jack can't hide from keith@cco.", " I like dogs"},
+ new Shape(1, 2));
+ NDList result = predictor.predict(new NDList(stringNd));
+ Assert.assertEquals(result.size(), 2);
+ Assert.assertEquals(result.get(0).toLongArray(), new long[] {1});
+ }
+ System.clearProperty("ai.djl.onnx.disable_alternative");
+ }
}
diff --git a/onnxruntime/onnxruntime-engine/src/test/resources/mlrepo/model/tabular/random_forest/ai/djl/onnxruntime/iris_flowers/metadata.json b/onnxruntime/onnxruntime-engine/src/test/resources/mlrepo/model/tabular/softmax_regression/ai/djl/onnxruntime/iris_flowers/metadata.json
similarity index 94%
rename from onnxruntime/onnxruntime-engine/src/test/resources/mlrepo/model/tabular/random_forest/ai/djl/onnxruntime/iris_flowers/metadata.json
rename to onnxruntime/onnxruntime-engine/src/test/resources/mlrepo/model/tabular/softmax_regression/ai/djl/onnxruntime/iris_flowers/metadata.json
index ced6365dc22..e5979c1b771 100644
--- a/onnxruntime/onnxruntime-engine/src/test/resources/mlrepo/model/tabular/random_forest/ai/djl/onnxruntime/iris_flowers/metadata.json
+++ b/onnxruntime/onnxruntime-engine/src/test/resources/mlrepo/model/tabular/softmax_regression/ai/djl/onnxruntime/iris_flowers/metadata.json
@@ -1,7 +1,7 @@
{
"metadataVersion": "0.2",
"resourceType": "model",
- "application": "tabular/random_forest",
+ "application": "tabular/softmax_regression",
"groupId": "ai.djl.onnxruntime",
"artifactId": "iris_flowers",
"name": "iris_flowers",
diff --git a/paddlepaddle/paddlepaddle-engine/src/main/java/ai/djl/paddlepaddle/engine/PpEngine.java b/paddlepaddle/paddlepaddle-engine/src/main/java/ai/djl/paddlepaddle/engine/PpEngine.java
index 22faec1e947..d2913b2eeca 100644
--- a/paddlepaddle/paddlepaddle-engine/src/main/java/ai/djl/paddlepaddle/engine/PpEngine.java
+++ b/paddlepaddle/paddlepaddle-engine/src/main/java/ai/djl/paddlepaddle/engine/PpEngine.java
@@ -16,6 +16,7 @@
import ai.djl.Model;
import ai.djl.engine.Engine;
import ai.djl.ndarray.NDManager;
+import ai.djl.nn.SymbolBlock;
import ai.djl.paddlepaddle.jni.JniUtils;
import ai.djl.paddlepaddle.jni.LibUtils;
import ai.djl.training.GradientCollector;
@@ -56,10 +57,13 @@ public int getRank() {
}
Engine getAlternativeEngine() {
+ if (Boolean.getBoolean("ai.djl.paddlepaddle.disable_alternative")) {
+ return null;
+ }
if (alternativeEngine == null) {
Engine engine = Engine.getInstance();
if (engine.getRank() < getRank()) {
- // alternativeEngine should not have the same rank as ORT
+ // alternativeEngine should not have the same rank as Paddle
alternativeEngine = engine;
}
}
@@ -85,6 +89,12 @@ public Model newModel(String name, Device device) {
return new PpModel(name, newBaseManager(device));
}
+ /** {@inheritDoc} */
+ @Override
+ public SymbolBlock newSymbolBlock(NDManager manager) {
+ throw new UnsupportedOperationException("PaddlePaddle does not support empty SymbolBlock");
+ }
+
/** {@inheritDoc} */
@Override
public NDManager newBaseManager() {
diff --git a/paddlepaddle/paddlepaddle-engine/src/main/java/ai/djl/paddlepaddle/engine/PpModel.java b/paddlepaddle/paddlepaddle-engine/src/main/java/ai/djl/paddlepaddle/engine/PpModel.java
index 458b7333d23..25ebbb4c680 100644
--- a/paddlepaddle/paddlepaddle-engine/src/main/java/ai/djl/paddlepaddle/engine/PpModel.java
+++ b/paddlepaddle/paddlepaddle-engine/src/main/java/ai/djl/paddlepaddle/engine/PpModel.java
@@ -96,22 +96,6 @@ private String[] findModelFile(Path dir) {
return null;
}
- /** {@inheritDoc} */
- @Override
- public String toString() {
- StringBuilder sb = new StringBuilder(200);
- sb.append("Model (\n\tName: ").append(modelName);
- if (modelDir != null) {
- sb.append("\n\tModel location: ").append(modelDir.toAbsolutePath());
- }
- sb.append("\n\tData Type: ").append(dataType);
- for (Map.Entry entry : properties.entrySet()) {
- sb.append("\n\t").append(entry.getKey()).append(": ").append(entry.getValue());
- }
- sb.append("\n)");
- return sb.toString();
- }
-
/** {@inheritDoc} */
@Override
public Predictor newPredictor(Translator translator) {
diff --git a/paddlepaddle/paddlepaddle-engine/src/main/java/ai/djl/paddlepaddle/engine/PpNDArray.java b/paddlepaddle/paddlepaddle-engine/src/main/java/ai/djl/paddlepaddle/engine/PpNDArray.java
index 121213161ee..cdb7ba30ba8 100644
--- a/paddlepaddle/paddlepaddle-engine/src/main/java/ai/djl/paddlepaddle/engine/PpNDArray.java
+++ b/paddlepaddle/paddlepaddle-engine/src/main/java/ai/djl/paddlepaddle/engine/PpNDArray.java
@@ -39,24 +39,7 @@ public class PpNDArray extends NativeResource implements NDArrayAdapter {
public PpNDArray(PpNDManager manager, long handle) {
super(handle);
this.manager = manager;
- manager.attach(getUid(), this);
- }
-
- /**
- * Constructs an PaddlePaddle NDArray from a {@link PpNDManager} (internal. Use {@link
- * NDManager} instead).
- *
- * @param manager the manager to attach the new array to
- * @param pointer the native tensor handle
- * @param shape the shape of {@code PpNDArray}
- * @param dataType the data type of {@code PpNDArray}
- */
- public PpNDArray(PpNDManager manager, long pointer, Shape shape, DataType dataType) {
- super(pointer);
- this.manager = manager;
- this.shape = shape;
- this.dataType = dataType;
- manager.attach(getUid(), this);
+ manager.attachInternal(getUid(), this);
}
/** {@inheritDoc} */
@@ -103,18 +86,25 @@ public Shape getShape() {
/** {@inheritDoc} */
@Override
- public NDManager attach(NDManager manager) {
+ public void attach(NDManager manager) {
+ detach();
+ this.manager = (PpNDManager) manager;
+ manager.attachInternal(getUid(), this);
+ }
+
+ /** {@inheritDoc} */
+ @Override
+ public void tempAttach(NDManager manager) {
detach();
NDManager original = this.manager;
this.manager = (PpNDManager) manager;
- manager.attach(getUid(), this);
- return original;
+ manager.tempAttachInternal(original, getUid(), this);
}
/** {@inheritDoc} */
@Override
public void detach() {
- manager.detach(getUid());
+ manager.detachInternal(getUid());
manager = PpNDManager.getSystemManager();
}
diff --git a/paddlepaddle/paddlepaddle-engine/src/main/java/ai/djl/paddlepaddle/engine/PpNDManager.java b/paddlepaddle/paddlepaddle-engine/src/main/java/ai/djl/paddlepaddle/engine/PpNDManager.java
index b277456af7b..f0aca98460d 100644
--- a/paddlepaddle/paddlepaddle-engine/src/main/java/ai/djl/paddlepaddle/engine/PpNDManager.java
+++ b/paddlepaddle/paddlepaddle-engine/src/main/java/ai/djl/paddlepaddle/engine/PpNDManager.java
@@ -51,7 +51,7 @@ public PpNDManager newSubManager() {
@Override
public PpNDManager newSubManager(Device device) {
PpNDManager manager = new PpNDManager(this, device);
- attach(manager.uid, manager);
+ attachInternal(manager.uid, manager);
return manager;
}
@@ -156,11 +156,11 @@ private static final class SystemManager extends PpNDManager {
/** {@inheritDoc} */
@Override
- public void attach(String resourceId, AutoCloseable resource) {}
+ public void attachInternal(String resourceId, AutoCloseable resource) {}
/** {@inheritDoc} */
@Override
- public void detach(String resourceId) {}
+ public void detachInternal(String resourceId) {}
/** {@inheritDoc} */
@Override
diff --git a/paddlepaddle/paddlepaddle-engine/src/main/java/ai/djl/paddlepaddle/engine/PpSymbolBlock.java b/paddlepaddle/paddlepaddle-engine/src/main/java/ai/djl/paddlepaddle/engine/PpSymbolBlock.java
index 522a928b32b..43b3a960f4c 100644
--- a/paddlepaddle/paddlepaddle-engine/src/main/java/ai/djl/paddlepaddle/engine/PpSymbolBlock.java
+++ b/paddlepaddle/paddlepaddle-engine/src/main/java/ai/djl/paddlepaddle/engine/PpSymbolBlock.java
@@ -98,7 +98,7 @@ private NDList getOutputs(PpNDArray[] outputs, boolean foreignEngine, NDManager
/** {@inheritDoc} */
@Override
- public Shape[] getOutputShapes(NDManager manager, Shape[] inputShapes) {
+ public Shape[] getOutputShapes(Shape[] inputShapes) {
return new Shape[0];
}
}
diff --git a/pytorch/pytorch-engine/src/main/java/ai/djl/pytorch/engine/PtEngine.java b/pytorch/pytorch-engine/src/main/java/ai/djl/pytorch/engine/PtEngine.java
index 4830addd0b8..4002ef76308 100644
--- a/pytorch/pytorch-engine/src/main/java/ai/djl/pytorch/engine/PtEngine.java
+++ b/pytorch/pytorch-engine/src/main/java/ai/djl/pytorch/engine/PtEngine.java
@@ -17,6 +17,7 @@
import ai.djl.engine.Engine;
import ai.djl.engine.EngineException;
import ai.djl.ndarray.NDManager;
+import ai.djl.nn.SymbolBlock;
import ai.djl.pytorch.jni.JniUtils;
import ai.djl.pytorch.jni.LibUtils;
import ai.djl.training.GradientCollector;
@@ -81,6 +82,12 @@ public boolean hasCapability(String capability) {
return JniUtils.getFeatures().contains(capability);
}
+ /** {@inheritDoc} */
+ @Override
+ public SymbolBlock newSymbolBlock(NDManager manager) {
+ return new PtSymbolBlock((PtNDManager) manager);
+ }
+
/** {@inheritDoc} */
@Override
public Model newModel(String name, Device device) {
diff --git a/pytorch/pytorch-engine/src/main/java/ai/djl/pytorch/engine/PtModel.java b/pytorch/pytorch-engine/src/main/java/ai/djl/pytorch/engine/PtModel.java
index 51ec90497ac..20ee7430fb2 100644
--- a/pytorch/pytorch-engine/src/main/java/ai/djl/pytorch/engine/PtModel.java
+++ b/pytorch/pytorch-engine/src/main/java/ai/djl/pytorch/engine/PtModel.java
@@ -17,17 +17,22 @@
import ai.djl.MalformedModelException;
import ai.djl.Model;
import ai.djl.ndarray.types.DataType;
+import ai.djl.nn.Parameter;
import ai.djl.pytorch.jni.JniUtils;
import ai.djl.training.Trainer;
import ai.djl.training.TrainingConfig;
import ai.djl.training.initializer.Initializer;
+import ai.djl.util.Pair;
+import ai.djl.util.PairList;
import java.io.FileNotFoundException;
import java.io.IOException;
+import java.io.InputStream;
import java.nio.file.Files;
import java.nio.file.Path;
import java.util.ArrayList;
import java.util.List;
import java.util.Map;
+import java.util.function.Predicate;
import java.util.stream.Collectors;
/**
@@ -97,6 +102,18 @@ public void load(Path modelPath, String prefix, Map options)
}
}
+ /**
+ * Load PyTorch model from {@link InputStream}.
+ *
+ *
Currently, only TorchScript file are supported
+ *
+ * @param modelStream the stream of the model file
+ * @throws IOException model loading error
+ */
+ public void load(InputStream modelStream) throws IOException {
+ block = JniUtils.loadModule((PtNDManager) manager, modelStream, manager.getDevice(), false);
+ }
+
private Path findModelFile(String prefix) {
if (Files.isRegularFile(modelDir)) {
Path file = modelDir;
@@ -125,12 +142,16 @@ private Path findModelFile(String prefix) {
/** {@inheritDoc} */
@Override
public Trainer newTrainer(TrainingConfig trainingConfig) {
- Initializer initializer = trainingConfig.getInitializer();
+ PairList> initializer = trainingConfig.getInitializers();
if (block == null) {
throw new IllegalStateException(
"You must set a block for the model before creating a new trainer");
}
- block.setInitializer(initializer);
+ for (Pair> pair : initializer) {
+ if (pair.getKey() != null && pair.getValue() != null) {
+ block.setInitializer(pair.getKey(), pair.getValue());
+ }
+ }
return new Trainer(this, trainingConfig);
}
diff --git a/pytorch/pytorch-engine/src/main/java/ai/djl/pytorch/engine/PtNDArray.java b/pytorch/pytorch-engine/src/main/java/ai/djl/pytorch/engine/PtNDArray.java
index dedf1988561..93f811326c0 100644
--- a/pytorch/pytorch-engine/src/main/java/ai/djl/pytorch/engine/PtNDArray.java
+++ b/pytorch/pytorch-engine/src/main/java/ai/djl/pytorch/engine/PtNDArray.java
@@ -69,7 +69,7 @@ public PtNDArray(PtNDManager manager, long handle) {
super(handle);
this.manager = manager;
this.ptNDArrayEx = new PtNDArrayEx(this);
- manager.attach(getUid(), this);
+ manager.attachInternal(getUid(), this);
}
/**
@@ -84,7 +84,7 @@ public PtNDArray(PtNDManager manager, long handle, ByteBuffer data) {
super(handle);
this.manager = manager;
this.ptNDArrayEx = new PtNDArrayEx(this);
- manager.attach(getUid(), this);
+ manager.attachInternal(getUid(), this);
dataRef = data;
}
@@ -211,6 +211,12 @@ public ByteBuffer toByteBuffer() {
return JniUtils.getByteBuffer(this);
}
+ /** {@inheritDoc} */
+ @Override
+ public String[] toStringArray() {
+ throw new UnsupportedOperationException("String NDArray is not supported!");
+ }
+
/** {@inheritDoc} */
@Override
public void set(Buffer data) {
@@ -279,18 +285,25 @@ public void copyTo(NDArray array) {
/** {@inheritDoc} */
@Override
- public NDManager attach(NDManager manager) {
+ public void attach(NDManager manager) {
+ detach();
+ this.manager = (PtNDManager) manager;
+ manager.attachInternal(getUid(), this);
+ }
+
+ /** {@inheritDoc} */
+ @Override
+ public void tempAttach(NDManager manager) {
detach();
NDManager original = this.manager;
this.manager = (PtNDManager) manager;
- manager.attach(getUid(), this);
- return original;
+ manager.tempAttachInternal(original, getUid(), this);
}
/** {@inheritDoc} */
@Override
public void detach() {
- manager.detach(getUid());
+ manager.detachInternal(getUid());
manager = PtNDManager.getSystemManager();
}
@@ -1436,7 +1449,7 @@ public void close() {
Long pointer = handle.getAndSet(null);
if (pointer != null) {
JniUtils.deleteNDArray(pointer);
- manager.detach(getUid());
+ manager.detachInternal(getUid());
manager = null;
dataRef = null;
}
diff --git a/pytorch/pytorch-engine/src/main/java/ai/djl/pytorch/engine/PtNDManager.java b/pytorch/pytorch-engine/src/main/java/ai/djl/pytorch/engine/PtNDManager.java
index ac51d54180c..7d2fa9a9123 100644
--- a/pytorch/pytorch-engine/src/main/java/ai/djl/pytorch/engine/PtNDManager.java
+++ b/pytorch/pytorch-engine/src/main/java/ai/djl/pytorch/engine/PtNDManager.java
@@ -181,7 +181,7 @@ public NDArray randomNormal(float loc, float scale, Shape shape, DataType dataTy
@Override
public PtNDManager newSubManager(Device device) {
PtNDManager manager = new PtNDManager(this, device);
- attach(manager.uid, manager);
+ attachInternal(manager.uid, manager);
return manager;
}
@@ -200,11 +200,11 @@ private static final class SystemManager extends PtNDManager {
/** {@inheritDoc} */
@Override
- public void attach(String resourceId, AutoCloseable resource) {}
+ public void attachInternal(String resourceId, AutoCloseable resource) {}
/** {@inheritDoc} */
@Override
- public void detach(String resourceId) {}
+ public void detachInternal(String resourceId) {}
/** {@inheritDoc} */
@Override
diff --git a/pytorch/pytorch-engine/src/main/java/ai/djl/pytorch/engine/PtSymbolBlock.java b/pytorch/pytorch-engine/src/main/java/ai/djl/pytorch/engine/PtSymbolBlock.java
index 7cf0e5e3ac8..9eebb11846b 100644
--- a/pytorch/pytorch-engine/src/main/java/ai/djl/pytorch/engine/PtSymbolBlock.java
+++ b/pytorch/pytorch-engine/src/main/java/ai/djl/pytorch/engine/PtSymbolBlock.java
@@ -65,7 +65,7 @@ public PtSymbolBlock(PtNDManager manager, long handle) {
this.handle = new AtomicReference<>(handle);
this.manager = manager;
uid = String.valueOf(handle);
- manager.attach(uid, this);
+ manager.attachInternal(uid, this);
// training mode is on by default
isTrain = true;
first = true;
@@ -90,7 +90,7 @@ public void close() {
Long pointer = handle.getAndSet(null);
if (pointer != null) {
JniUtils.deleteModule(pointer);
- manager.detach(uid);
+ manager.detachInternal(uid);
manager = null;
}
}
@@ -155,7 +155,7 @@ public PairList describeOutput() {
/** {@inheritDoc} */
@Override
- public Shape[] getOutputShapes(NDManager manager, Shape[] inputShapes) {
+ public Shape[] getOutputShapes(Shape[] inputShapes) {
return new Shape[0];
}
@@ -177,7 +177,7 @@ public void loadParameters(NDManager manager, DataInputStream is)
long rawHandle = JniUtils.loadModuleHandle(is, manager.getDevice(), true);
this.handle = new AtomicReference<>(rawHandle);
uid = String.valueOf(rawHandle);
- manager.attach(uid, this);
+ manager.attachInternal(uid, this);
}
/**
diff --git a/pytorch/pytorch-engine/src/test/java/ai/djl/pytorch/integration/PtModelTest.java b/pytorch/pytorch-engine/src/test/java/ai/djl/pytorch/integration/PtModelTest.java
new file mode 100644
index 00000000000..cda9b86d8dd
--- /dev/null
+++ b/pytorch/pytorch-engine/src/test/java/ai/djl/pytorch/integration/PtModelTest.java
@@ -0,0 +1,43 @@
+/*
+ * Copyright 2021 Amazon.com, Inc. or its affiliates. All Rights Reserved.
+ *
+ * Licensed under the Apache License, Version 2.0 (the "License"). You may not use this file except in compliance
+ * with the License. A copy of the License is located at
+ *
+ * http://aws.amazon.com/apache2.0/
+ *
+ * or in the "license" file accompanying this file. This file is distributed on an "AS IS" BASIS, WITHOUT WARRANTIES
+ * OR CONDITIONS OF ANY KIND, either express or implied. See the License for the specific language governing permissions
+ * and limitations under the License.
+ */
+package ai.djl.pytorch.integration;
+
+import ai.djl.Model;
+import ai.djl.inference.Predictor;
+import ai.djl.ndarray.NDArray;
+import ai.djl.ndarray.NDList;
+import ai.djl.ndarray.types.Shape;
+import ai.djl.pytorch.engine.PtModel;
+import ai.djl.translate.NoopTranslator;
+import ai.djl.translate.TranslateException;
+import java.io.IOException;
+import java.net.URL;
+import org.testng.Assert;
+import org.testng.annotations.Test;
+
+public class PtModelTest {
+
+ @Test
+ public void testLoadFromStream() throws IOException, TranslateException {
+ URL url =
+ new URL("https://djl-ai.s3.amazonaws.com/resources/test-models/traced_resnet18.pt");
+ try (PtModel model = (PtModel) Model.newInstance("test model")) {
+ model.load(url.openStream());
+ try (Predictor predictor = model.newPredictor(new NoopTranslator())) {
+ NDArray array = model.getNDManager().ones(new Shape(1, 3, 224, 224));
+ NDArray result = predictor.predict(new NDList(array)).singletonOrThrow();
+ Assert.assertEquals(result.getShape(), new Shape(1, 1000));
+ }
+ }
+ }
+}
diff --git a/serving/src/test/java/ai/djl/serving/ModelServerTest.java b/serving/src/test/java/ai/djl/serving/ModelServerTest.java
index fe6eef7ec25..1195af84b25 100644
--- a/serving/src/test/java/ai/djl/serving/ModelServerTest.java
+++ b/serving/src/test/java/ai/djl/serving/ModelServerTest.java
@@ -434,6 +434,7 @@ private void testInvalidRootRequest() throws InterruptedException {
HttpRequest req = new DefaultFullHttpRequest(HttpVersion.HTTP_1_1, HttpMethod.GET, "/");
channel.writeAndFlush(req).sync();
latch.await();
+ channel.closeFuture().sync();
channel.close();
ErrorResponse resp = JsonUtils.GSON.fromJson(result, ErrorResponse.class);
@@ -452,6 +453,7 @@ private void testInvalidUri() throws InterruptedException {
new DefaultFullHttpRequest(HttpVersion.HTTP_1_1, HttpMethod.GET, "/InvalidUrl");
channel.writeAndFlush(req).sync();
latch.await();
+ channel.closeFuture().sync();
channel.close();
ErrorResponse resp = JsonUtils.GSON.fromJson(result, ErrorResponse.class);
@@ -471,6 +473,7 @@ private void testInvalidDescribeModel() throws InterruptedException {
HttpVersion.HTTP_1_1, HttpMethod.OPTIONS, "/predictions/InvalidModel");
channel.writeAndFlush(req).sync();
latch.await();
+ channel.closeFuture().sync();
channel.close();
ErrorResponse resp = JsonUtils.GSON.fromJson(result, ErrorResponse.class);
@@ -489,6 +492,7 @@ private void testInvalidPredictionsUri() throws InterruptedException {
new DefaultFullHttpRequest(HttpVersion.HTTP_1_1, HttpMethod.GET, "/predictions");
channel.writeAndFlush(req).sync();
latch.await();
+ channel.closeFuture().sync();
channel.close();
ErrorResponse resp = JsonUtils.GSON.fromJson(result, ErrorResponse.class);
@@ -508,6 +512,7 @@ private void testPredictionsModelNotFound() throws InterruptedException {
HttpVersion.HTTP_1_1, HttpMethod.GET, "/predictions/InvalidModel");
channel.writeAndFlush(req).sync();
latch.await();
+ channel.closeFuture().sync();
channel.close();
ErrorResponse resp = JsonUtils.GSON.fromJson(result, ErrorResponse.class);
@@ -526,6 +531,7 @@ private void testInvalidManagementUri() throws InterruptedException {
new DefaultFullHttpRequest(HttpVersion.HTTP_1_1, HttpMethod.GET, "/InvalidUrl");
channel.writeAndFlush(req).sync();
latch.await();
+ channel.closeFuture().sync();
channel.close();
ErrorResponse resp = JsonUtils.GSON.fromJson(result, ErrorResponse.class);
@@ -544,6 +550,7 @@ private void testInvalidManagementMethod() throws InterruptedException {
new DefaultFullHttpRequest(HttpVersion.HTTP_1_1, HttpMethod.PUT, "/models");
channel.writeAndFlush(req).sync();
latch.await();
+ channel.closeFuture().sync();
channel.close();
ErrorResponse resp = JsonUtils.GSON.fromJson(result, ErrorResponse.class);
@@ -562,6 +569,7 @@ private void testInvalidPredictionsMethod() throws InterruptedException {
new DefaultFullHttpRequest(HttpVersion.HTTP_1_1, HttpMethod.POST, "/models/noop");
channel.writeAndFlush(req).sync();
latch.await();
+ channel.closeFuture().sync();
channel.close();
ErrorResponse resp = JsonUtils.GSON.fromJson(result, ErrorResponse.class);
@@ -581,6 +589,7 @@ private void testDescribeModelNotFound() throws InterruptedException {
HttpVersion.HTTP_1_1, HttpMethod.GET, "/models/InvalidModel");
channel.writeAndFlush(req).sync();
latch.await();
+ channel.closeFuture().sync();
channel.close();
ErrorResponse resp = JsonUtils.GSON.fromJson(result, ErrorResponse.class);
@@ -599,6 +608,7 @@ private void testRegisterModelMissingUrl() throws InterruptedException {
new DefaultFullHttpRequest(HttpVersion.HTTP_1_1, HttpMethod.POST, "/models");
channel.writeAndFlush(req).sync();
latch.await();
+ channel.closeFuture().sync();
channel.close();
ErrorResponse resp = JsonUtils.GSON.fromJson(result, ErrorResponse.class);
@@ -618,6 +628,7 @@ private void testRegisterModelNotFound() throws InterruptedException {
HttpVersion.HTTP_1_1, HttpMethod.POST, "/models?url=InvalidUrl");
channel.writeAndFlush(req).sync();
latch.await();
+ channel.closeFuture().sync();
channel.close();
ErrorResponse resp = JsonUtils.GSON.fromJson(result, ErrorResponse.class);
@@ -643,6 +654,7 @@ private void testRegisterModelConflict()
+ URLEncoder.encode(url, StandardCharsets.UTF_8.name()));
channel.writeAndFlush(req);
latch.await();
+ channel.closeFuture().sync();
channel.close();
ErrorResponse resp = JsonUtils.GSON.fromJson(result, ErrorResponse.class);
@@ -663,6 +675,7 @@ private void testInvalidScaleModel() throws InterruptedException {
"/models/mlp?min_worker=10&max_worker=1");
channel.writeAndFlush(req).sync();
latch.await();
+ channel.closeFuture().sync();
channel.close();
ErrorResponse resp = JsonUtils.GSON.fromJson(result, ErrorResponse.class);
@@ -681,6 +694,7 @@ private void testScaleModelNotFound() throws InterruptedException {
new DefaultFullHttpRequest(HttpVersion.HTTP_1_1, HttpMethod.PUT, "/models/fake");
channel.writeAndFlush(req).sync();
latch.await();
+ channel.closeFuture().sync();
channel.close();
ErrorResponse resp = JsonUtils.GSON.fromJson(result, ErrorResponse.class);
@@ -699,6 +713,7 @@ private void testUnregisterModelNotFound() throws InterruptedException {
new DefaultFullHttpRequest(HttpVersion.HTTP_1_1, HttpMethod.DELETE, "/models/fake");
channel.writeAndFlush(req).sync();
latch.await();
+ channel.closeFuture().sync();
channel.close();
ErrorResponse resp = JsonUtils.GSON.fromJson(result, ErrorResponse.class);
@@ -731,6 +746,7 @@ private void testServiceUnavailable() throws InterruptedException {
req.headers().set(HttpHeaderNames.CONTENT_TYPE, HttpHeaderValues.APPLICATION_OCTET_STREAM);
channel.writeAndFlush(req).sync();
latch.await();
+ channel.closeFuture().sync();
channel.close();
ErrorResponse resp = JsonUtils.GSON.fromJson(result, ErrorResponse.class);
diff --git a/tensorflow/tensorflow-engine/src/main/java/ai/djl/tensorflow/engine/TfEngine.java b/tensorflow/tensorflow-engine/src/main/java/ai/djl/tensorflow/engine/TfEngine.java
index 6b2629a72f6..9ab49d24b87 100644
--- a/tensorflow/tensorflow-engine/src/main/java/ai/djl/tensorflow/engine/TfEngine.java
+++ b/tensorflow/tensorflow-engine/src/main/java/ai/djl/tensorflow/engine/TfEngine.java
@@ -18,6 +18,7 @@
import ai.djl.engine.EngineException;
import ai.djl.engine.StandardCapabilities;
import ai.djl.ndarray.NDManager;
+import ai.djl.nn.SymbolBlock;
import ai.djl.training.GradientCollector;
import ai.djl.util.RandomUtils;
import org.tensorflow.EagerSession;
@@ -56,6 +57,12 @@ public Model newModel(String name, Device device) {
return new TfModel(name, device);
}
+ /** {@inheritDoc} */
+ @Override
+ public SymbolBlock newSymbolBlock(NDManager manager) {
+ throw new UnsupportedOperationException("TensorFlow does not support empty SymbolBlock");
+ }
+
/** {@inheritDoc} */
@Override
public String getEngineName() {
diff --git a/tensorflow/tensorflow-engine/src/main/java/ai/djl/tensorflow/engine/TfNDArray.java b/tensorflow/tensorflow-engine/src/main/java/ai/djl/tensorflow/engine/TfNDArray.java
index 478257d23b7..76dcefbbf3a 100644
--- a/tensorflow/tensorflow-engine/src/main/java/ai/djl/tensorflow/engine/TfNDArray.java
+++ b/tensorflow/tensorflow-engine/src/main/java/ai/djl/tensorflow/engine/TfNDArray.java
@@ -73,7 +73,7 @@ public class TfNDArray implements NDArray {
this.manager = (TfNDManager) manager;
this.tf = this.manager.getTf();
uid = UUID.randomUUID().toString();
- manager.attach(uid, this);
+ manager.attachInternal(uid, this);
this.operand =
this.manager
.getEagerSession()
@@ -257,6 +257,13 @@ public boolean[] toBooleanArray() {
return result;
}
+ @Override
+ public String[] toStringArray() {
+ // TODO: Parse String Array from bytes[]
+ throw new UnsupportedOperationException(
+ "TensorFlow does not supporting printing String NDArray");
+ }
+
/** {@inheritDoc} */
@Override
public ByteBuffer toByteBuffer() {
@@ -279,18 +286,25 @@ public void set(Buffer data) {
/** {@inheritDoc} */
@Override
- public NDManager attach(NDManager manager) {
+ public void attach(NDManager manager) {
+ detach();
+ this.manager = (TfNDManager) manager;
+ manager.attachInternal(uid, this);
+ }
+
+ /** {@inheritDoc} */
+ @Override
+ public void tempAttach(NDManager manager) {
detach();
NDManager original = this.manager;
this.manager = (TfNDManager) manager;
- manager.attach(uid, this);
- return original;
+ manager.tempAttachInternal(original, uid, this);
}
/** {@inheritDoc} */
@Override
public void detach() {
- manager.detach(getUid());
+ manager.detachInternal(getUid());
manager = TfNDManager.getSystemManager();
}
diff --git a/tensorflow/tensorflow-engine/src/main/java/ai/djl/tensorflow/engine/TfNDManager.java b/tensorflow/tensorflow-engine/src/main/java/ai/djl/tensorflow/engine/TfNDManager.java
index efa991c8008..90048fe9c2c 100644
--- a/tensorflow/tensorflow-engine/src/main/java/ai/djl/tensorflow/engine/TfNDManager.java
+++ b/tensorflow/tensorflow-engine/src/main/java/ai/djl/tensorflow/engine/TfNDManager.java
@@ -149,12 +149,19 @@ public NDArray create(float data) {
/** {@inheritDoc} */
@Override
public NDArray create(String data) {
- // create scalar tensor with float
try (Tensor tensor = TString.scalarOf(data)) {
return new TfNDArray(this, tensor);
}
}
+ /** {@inheritDoc} */
+ @Override
+ public NDArray create(String[] data) {
+ try (Tensor tensor = TString.vectorOf(data)) {
+ return new TfNDArray(this, tensor);
+ }
+ }
+
/** {@inheritDoc} */
@Override
public NDArray create(Shape shape, DataType dataType) {
@@ -416,7 +423,7 @@ public NDArray randomNormal(float loc, float scale, Shape shape, DataType dataTy
@Override
public TfNDManager newSubManager(Device device) {
TfNDManager manager = new TfNDManager(this, device);
- attach(manager.uid, manager);
+ attachInternal(manager.uid, manager);
// initialize eager sessions and operators only for sub managers
manager.getEagerSession();
manager.getTf();
@@ -440,11 +447,11 @@ private static final class SystemManager extends TfNDManager {
/** {@inheritDoc} */
@Override
- public void attach(String resrouceId, AutoCloseable resource) {}
+ public void attachInternal(String resrouceId, AutoCloseable resource) {}
/** {@inheritDoc} */
@Override
- public void detach(String resourceId) {}
+ public void detachInternal(String resourceId) {}
/** {@inheritDoc} */
@Override
diff --git a/tensorflow/tensorflow-engine/src/main/java/ai/djl/tensorflow/engine/TfSymbolBlock.java b/tensorflow/tensorflow-engine/src/main/java/ai/djl/tensorflow/engine/TfSymbolBlock.java
index 43f667d2dc2..7a6f8bdb753 100644
--- a/tensorflow/tensorflow-engine/src/main/java/ai/djl/tensorflow/engine/TfSymbolBlock.java
+++ b/tensorflow/tensorflow-engine/src/main/java/ai/djl/tensorflow/engine/TfSymbolBlock.java
@@ -131,8 +131,8 @@ protected NDList forwardInternal(
/** {@inheritDoc} */
@Override
- public Shape[] initialize(NDManager manager, DataType dataType, Shape... inputShapes) {
- return new Shape[0];
+ public void initialize(NDManager manager, DataType dataType, Shape... inputShapes) {
+ throw new IllegalStateException("TfSymbolBlock can't be initialized");
}
/** {@inheritDoc} */
@@ -197,7 +197,7 @@ public final PairList describeOutput() {
/** {@inheritDoc} */
@Override
- public Shape[] getOutputShapes(NDManager manager, Shape[] inputShapes) {
+ public Shape[] getOutputShapes(Shape[] inputShapes) {
return new Shape[0];
}
diff --git a/tflite/tflite-engine/src/main/java/ai/djl/tflite/engine/TfLiteEngine.java b/tflite/tflite-engine/src/main/java/ai/djl/tflite/engine/TfLiteEngine.java
index 6d74876c825..140d6369f36 100644
--- a/tflite/tflite-engine/src/main/java/ai/djl/tflite/engine/TfLiteEngine.java
+++ b/tflite/tflite-engine/src/main/java/ai/djl/tflite/engine/TfLiteEngine.java
@@ -17,6 +17,7 @@
import ai.djl.Model;
import ai.djl.engine.Engine;
import ai.djl.ndarray.NDManager;
+import ai.djl.nn.SymbolBlock;
import ai.djl.training.GradientCollector;
/**
@@ -53,6 +54,9 @@ public int getRank() {
}
private Engine getAlternativeEngine() {
+ if (Boolean.getBoolean("ai.djl.tflite.disable_alternative")) {
+ return null;
+ }
if (alternativeEngine == null) {
Engine engine = Engine.getInstance();
if (engine.getRank() < getRank()) {
@@ -66,7 +70,7 @@ private Engine getAlternativeEngine() {
/** {@inheritDoc} */
@Override
public String getVersion() {
- return "1.4.0";
+ return "2.4.1";
}
/** {@inheritDoc} */
@@ -83,6 +87,12 @@ public Model newModel(String name, Device device) {
return new TfLiteModel(name, newBaseManager(device));
}
+ /** {@inheritDoc} */
+ @Override
+ public SymbolBlock newSymbolBlock(NDManager manager) {
+ throw new UnsupportedOperationException("TFLite does not support empty SymbolBlock");
+ }
+
/** {@inheritDoc} */
@Override
public NDManager newBaseManager() {
diff --git a/tflite/tflite-engine/src/main/java/ai/djl/tflite/engine/TfLiteNDArray.java b/tflite/tflite-engine/src/main/java/ai/djl/tflite/engine/TfLiteNDArray.java
index 9b59b4893ab..86a87cc8fac 100644
--- a/tflite/tflite-engine/src/main/java/ai/djl/tflite/engine/TfLiteNDArray.java
+++ b/tflite/tflite-engine/src/main/java/ai/djl/tflite/engine/TfLiteNDArray.java
@@ -39,7 +39,7 @@ public class TfLiteNDArray implements NDArrayAdapter {
TfLiteNDArray(TfLiteNDManager manager, Tensor tensor) {
this.manager = manager;
uid = UUID.randomUUID().toString();
- manager.attach(uid, this);
+ manager.attachInternal(uid, this);
this.tensor = tensor;
shape = new Shape(Arrays.stream(tensor.shape()).mapToLong(i -> i).toArray());
dataType = TfLiteDataType.fromTf(tensor.dataType());
@@ -103,18 +103,25 @@ public SparseFormat getSparseFormat() {
/** {@inheritDoc} */
@Override
- public NDManager attach(NDManager manager) {
+ public void attach(NDManager manager) {
+ detach();
+ this.manager = (TfLiteNDManager) manager;
+ manager.attachInternal(getUid(), this);
+ }
+
+ /** {@inheritDoc} */
+ @Override
+ public void tempAttach(NDManager manager) {
detach();
NDManager original = this.manager;
this.manager = (TfLiteNDManager) manager;
- manager.attach(getUid(), this);
- return original;
+ manager.tempAttachInternal(original, getUid(), this);
}
/** {@inheritDoc} */
@Override
public void detach() {
- manager.detach(getUid());
+ manager.detachInternal(getUid());
manager = TfLiteNDManager.getSystemManager();
}
diff --git a/tflite/tflite-engine/src/main/java/ai/djl/tflite/engine/TfLiteNDManager.java b/tflite/tflite-engine/src/main/java/ai/djl/tflite/engine/TfLiteNDManager.java
index 8d55da2e7a1..64da62767f7 100644
--- a/tflite/tflite-engine/src/main/java/ai/djl/tflite/engine/TfLiteNDManager.java
+++ b/tflite/tflite-engine/src/main/java/ai/djl/tflite/engine/TfLiteNDManager.java
@@ -132,7 +132,7 @@ public NDArray ones(Shape shape, DataType dataType) {
@Override
public TfLiteNDManager newSubManager(Device device) {
TfLiteNDManager manager = new TfLiteNDManager(this, device);
- attach(manager.uid, manager);
+ attachInternal(manager.uid, manager);
return manager;
}
@@ -151,11 +151,11 @@ private static final class SystemManager extends TfLiteNDManager {
/** {@inheritDoc} */
@Override
- public void attach(String resourceId, AutoCloseable resource) {}
+ public void attachInternal(String resourceId, AutoCloseable resource) {}
/** {@inheritDoc} */
@Override
- public void detach(String resourceId) {}
+ public void detachInternal(String resourceId) {}
/** {@inheritDoc} */
@Override