-
Notifications
You must be signed in to change notification settings - Fork 4
/
hemibrain_alpns_toons.Rmd
320 lines (272 loc) · 12.6 KB
/
hemibrain_alpns_toons.Rmd
1
2
3
4
5
6
7
8
9
10
11
12
13
14
15
16
17
18
19
20
21
22
23
24
25
26
27
28
29
30
31
32
33
34
35
36
37
38
39
40
41
42
43
44
45
46
47
48
49
50
51
52
53
54
55
56
57
58
59
60
61
62
63
64
65
66
67
68
69
70
71
72
73
74
75
76
77
78
79
80
81
82
83
84
85
86
87
88
89
90
91
92
93
94
95
96
97
98
99
100
101
102
103
104
105
106
107
108
109
110
111
112
113
114
115
116
117
118
119
120
121
122
123
124
125
126
127
128
129
130
131
132
133
134
135
136
137
138
139
140
141
142
143
144
145
146
147
148
149
150
151
152
153
154
155
156
157
158
159
160
161
162
163
164
165
166
167
168
169
170
171
172
173
174
175
176
177
178
179
180
181
182
183
184
185
186
187
188
189
190
191
192
193
194
195
196
197
198
199
200
201
202
203
204
205
206
207
208
209
210
211
212
213
214
215
216
217
218
219
220
221
222
223
224
225
226
227
228
229
230
231
232
233
234
235
236
237
238
239
240
241
242
243
244
245
246
247
248
249
250
251
252
253
254
255
256
257
258
259
260
261
262
263
264
265
266
267
268
269
270
271
272
273
274
275
276
277
278
279
280
281
282
283
284
285
286
287
288
289
290
291
292
293
294
295
296
297
298
299
300
301
302
303
304
305
306
307
308
309
310
311
312
313
314
315
316
317
318
319
320
---
title: "olfactory connectivity"
output: rmarkdown::html_vignette
vignette: >
%\VignetteIndexEntry{olfactory connectivity}
%\VignetteEngine{knitr::rmarkdown}
%\VignetteEncoding{UTF-8}
---
```{r setup, include = FALSE}
knitr::opts_chunk$set(
collapse = TRUE,
comment = "#>"
)
```
# Connection data
In this guide, we will use examine the connectivity between antennal lobe projection neurons (ALPNs), the principal chemosensory second-order neurons of the fly brain, and their third order targets (TONs). We want to see whether certain groups of ALPNs preferentially connect with certain groups of TONs.
## Load google drive
Let's get hemibrainr and our Google drive loaded:
```{r see.paths, eval = FALSE}
# Load package
library(hemibrainr)
library(ggplot2)
library(gplots)
library(dplyr)
library(plyr)
library(reshape2)
library(ComplexHeatmap)
library(LaCroixColoR)
# Else, it wants to see it on the mounted team drive, here
options("Gdrive_hemibrain_data")
# If this does not give a path to the hemibrainr google drive...
## But you do have access, and have set up rclone
### Try:
##### hemibrainr_rclone()
```
## Find neurons of interest
First, let us find these neurons, so we can plot them if we like:
```{r lhmb1, eval = FALSE}
db = hemibrain_neurons()
alpns.nl= db[names(db)%in%hemibrainr::pn.info$bodyid]
ton.nl = db[names(db)%in%hemibrainr::ton.info$bodyid]
```
We can now plot them if we like:
```{r lhmb1.plot, eval = FALSE}
# Get ROIs
lhr = neuprint_ROI_mesh("LH(R)")
alr = neuprint_ROI_mesh("AL(R)")
# Plot
nat::nopen3d()
hemibrain_view()
plot3d(lhr, alpha = 0.3, col = hemibrain_bright_colors["green"], add = TRUE)
plot3d(alr, alpha = 0.3, col = hemibrain_bright_colors["red"], add = TRUE)
plot3d(hemibrain.surf, alpha = 0.1, col = "lightgrey", add = TRUE)
plot3d_split(alpns.nl, lwd =2, soma = 600, col = hemibrain_bright_colours["cerise"])
plot3d_split(ton.nl, lwd =2, soma = 600, col = hemibrain_bright_colour_ramp(length(ton.nl)))
```
## Get neuron connectivity data
Now we can get a precomputed edgelist for the brain from the Google drive, which breaks down connections by axon and dendrite. By default this is read from an SQLlite database on the Google drive. This means we can access the data, without loading a multi Gb .csv into memory:
```{r elist, eval = FALSE}
# Get the edgelist
elist = hemibrain_elist()
meta = rbind.fill(ton.info, pn.info)
```
In our `elist`, count is the number of synaptic connections between two arbours. The arbour types are given by `Label` and `partner.Label`. The neuron identities are given as `pre`, the upstream (source) neuron and `post`, the downstream (target) neuron. The value of `norm` is generated by taking `count` and dividing it by the total number of posynapses on the downstream target's given arbour (note, not all connection in the neuron!). See your [article](https://flyconnectome.github.io/hemibrainr/articles/hemibrain_axons_dendrites.html) on neuron splitting, for more information.
```{r alpn.ton, eval = FALSE}
alpn.lhn.elist <- elist %>%
filter(pre %in% local(pn.info$bodyid)
& post %in% local(ton.info$bodyid)
& pre.Label == "axon"
& post.Label %in% c("dendrite","axon")
& (count > 10 | norm > 0.01)) %>%
collect() # this is now a data frame. This may take a while to load into R.
# Add some cell type information
alpn.lhn.elist <- alpn.lhn.elist %>%
mutate(pre.cell.type = meta[match(pre,meta$bodyid),"cell.type"]) %>%
mutate(post.cell.type = meta[match(post,meta$bodyid),"cell.type"]) %>%
as.data.frame(stringsAsFactors=FALSE)
# Convert edgelist to a connectivity matrix
conn.mat = acast(data = alpn.lhn.elist,
formula = pre.cell.type ~ post.cell.type + post.Label,
fun.aggregate = mean,
value.var = "norm",
fill = 0)
# Negative weights for inhibitory connections
inhib.pns = unique(subset(pn.info, neurotransmitter %in% c("GABA","glutamate"))$cell.type)
conn.mat[inhib.pns,] = -conn.mat[inhib.pns,]
# Max normalisation
normalise <- function(x){
return(x/max(abs(x)))
}
conn.mat.scaled = t(apply(conn.mat,1,normalise))
```
Now we can plot a heatmaps of the ALPN->TON weights, and correlation heatmaps.
First let us get some clusterings for ALPNs and TONs based on ther cross-correlation.
```{r heatmap, eval = FALSE}
# TON correlation matrix
cor.mat = lsa::cosine(conn.mat.scaled)
# ALPN correlation matrix
cor.mat.2 = lsa::cosine(t(conn.mat.scaled))
# Grey tone for heatmap
heatmap.cols = circlize::colorRamp2(c(-1, -0.5, -0.25, 0, 0.25, 0.5, 1),
c(
hemibrain_bright_colors[["navy"]],
hemibrain_bright_colors[["blue"]],
hemibrain_bright_colors[["cyan"]],
"grey90",
hemibrain_bright_colors[["yellow"]],
hemibrain_bright_colors[["orange"]],
hemibrain_bright_colors[["cerise"]])
)
# dendrogram colours
apricot = colorRampPalette(LaCroixColoR::lacroix_palette("Apricot"))
# clustering
cclus = dendroextras::color_clusters(hclust(as.dist(1-cor.mat), method = "ward.D2"), h = 0.1, col = apricot)
rclus = dendroextras::color_clusters(hclust(as.dist(1-cor.mat.2), method = "ward.D2"), h = 0.1, col = apricot)
```
Plot dendrograms:
```{r dendrograms, eval = FALSE}
# Plot ALPN clustering
plot(rclus)
# Plot TON clustering
plot(cclus)
```
We can see what our data like quickly, by plotting some simple heatmaps:
```{r quick.heatmaps, eval = FALSE}
# Normalised matrix
heatmap.2(x = conn.mat.scaled, Rowv = rclus, Colv= cclus,
trace="none", na.color = "darkgrey", scale="none",
density.info = "none", key = TRUE, symkey=FALSE,
srtCol=0, srtRow = 0)
# Correlation matrix for TONs
heatmap.2(x = cor.mat, Rowv = cclus, Colv= cclus,
trace="none", na.color = "darkgrey", scale="none",
density.info = "none", key = TRUE, symkey=FALSE,
srtCol=0, srtRow = 0)
# Correlation matrix for ALPNs
heatmap.2(x = cor.mat.2, Rowv = rclus, Colv= rclus,
trace="none", na.color = "darkgrey", scale="none",
density.info = "none", key = TRUE, symkey=FALSE,
srtCol=0, srtRow = 0)
```
And now we can fully annotate our data with a more complex heatmap:
```{r complex.heatmap, eval = FALSE}
# columns annotations
ton.compartment = ifelse(grepl("axon",colnames(conn.mat.scaled)),"axon","dendrite")
cts = gsub("_axon$|_dendrite$","",colnames(conn.mat.scaled))
ton.layers = paste0("layer_",ton.info[match(cts,ton.info$cell.type),"ct.layer"])
ton.layers[ton.layers=="layer_NA"] = NA
ton.transmitter = ton.info[match(cts,ton.info$cell.type),"putative.classic.transmitter"]
ton.transmitter[ton.transmitter=="unknown"] = NA
ton.transmitter[ton.transmitter=="acetlcholine"] = "acetylcholine"
ton.class = ton.info[match(cts,ton.info$cell.type),"class"]
ton.class[ton.class=="LHN"] = "LHON"
ha = HeatmapAnnotation(
df = data.frame(class = ton.class,
layer = ton.layers,
transmitter = ton.transmitter,
compartment = ton.compartment),
annotation_height = unit(4, "mm"),
col = list(class = paper_colours, layer = paper_colours, transmitter = paper_colours, compartment = paper_colours),
na_col = "white"
)
# Row annotations
pn.class = pn.info[match(rownames(conn.mat.scaled),pn.info$cell.type),"class"]
pn.class[pn.class=="biPN"] = "mPN"
pn.neurotransmitter = pn.info[match(rownames(conn.mat.scaled),pn.info$cell.type),"neurotransmitter"]
pn.ct.layer = as.character(pn.info[match(rownames(conn.mat.scaled),pn.info$cell.type),"ct.layer"])
pn.os = pn.info[match(rownames(conn.mat.scaled),pn.info$cell.type),"odour.scenes"]
pn.os = strsplit(pn.os,"/")
pn.os = sapply(pn.os, sort)
pn.os.1 = sapply(pn.os, function(x) hemibrainr:::nullToNA(x[1]))
pn.os.2 = sapply(pn.os, function(x) hemibrainr:::nullToNA(x[2]))
pn.os.3 = sapply(pn.os, function(x) hemibrainr:::nullToNA(x[3]))
ha2 = HeatmapAnnotation(
df = data.frame(class = pn.class,
layer = pn.ct.layer,
transmitter = pn.neurotransmitter,
modality.1 = pn.os.1,
modality.2 = pn.os.2,
modality.3 = pn.os.3),
annotation_height = unit(4, "mm"),
col = list(class = paper_colours,
layer = paper_colours,
transmitter = paper_colours,
modality.1 = paper_colours,
modality.2 = paper_colours,
modality.3 = paper_colours),
na_col = "white"
)
# Plot complex heatmap!
pdf("ALPN_TOON_normalised_connectivity.pdf", height = 14, width = 10)
Heatmap(conn.mat.scaled,
split = 10,
name = " ",
cluster_rows = rclus,
cluster_columns = cclus,
top_annotation = ha,
show_row_names = FALSE,
show_column_names = FALSE,
col = heatmap.cols,
heatmap_legend_param = list(by_row = TRUE, ncol = 1, legend_direction = "vertical", grid_width = unit(2, "mm")),
row_title_gp = gpar(fontsize = 14, fontfamily ="Helvetica"),
column_title_gp = gpar(fontsize = 14, fontfamily ="Helvetica"),
row_names_gp = gpar(fontsize = 12, fontfamily ="Helvetica"),
column_names_gp = gpar(fontsize = 12, fontfamily ="Helvetica")) +
Heatmap(pn.class, name = "class", width = unit(5, "mm"), col = paper_colours, na_col = "white",
row_title_gp = gpar(fontsize = 14, fontfamily ="Helvetica"),
column_title_gp = gpar(fontsize = 14, fontfamily ="Helvetica"),
column_names_gp = gpar(fontsize = 12, fontfamily ="Helvetica")) +
Heatmap(pn.ct.layer, name = "layer", width = unit(5, "mm"), col = paper_colours, na_col = "white",
row_title_gp = gpar(fontsize = 14, fontfamily ="Helvetica"),
column_title_gp = gpar(fontsize = 14, fontfamily ="Helvetica"),
column_names_gp = gpar(fontsize = 12, fontfamily ="Helvetica")) +
Heatmap(pn.os.1, name = "modality.1", width = unit(5, "mm"), col = paper_colours, show_row_names = FALSE, na_col = "white",
row_title_gp = gpar(fontsize = 14, fontfamily ="Helvetica"),
column_title_gp = gpar(fontsize = 14, fontfamily ="Helvetica"),
column_names_gp = gpar(fontsize = 12, fontfamily ="Helvetica")) +
Heatmap(pn.os.2, name = "modality.2", width = unit(5, "mm"), col = paper_colours, show_row_names = FALSE, na_col = "white",
row_title_gp = gpar(fontsize = 14, fontfamily ="Helvetica"),
column_title_gp = gpar(fontsize = 14, fontfamily ="Helvetica"),
column_names_gp = gpar(fontsize = 12, fontfamily ="Helvetica")) +
Heatmap(pn.os.3, name = "modality.3", width = unit(5, "mm"), col = paper_colours, show_row_names = FALSE, na_col = "white",
row_title_gp = gpar(fontsize = 14, fontfamily ="Helvetica"),
column_title_gp = gpar(fontsize = 14, fontfamily ="Helvetica"),
column_names_gp = gpar(fontsize = 12, fontfamily ="Helvetica"))
dev.off()
```
<center>
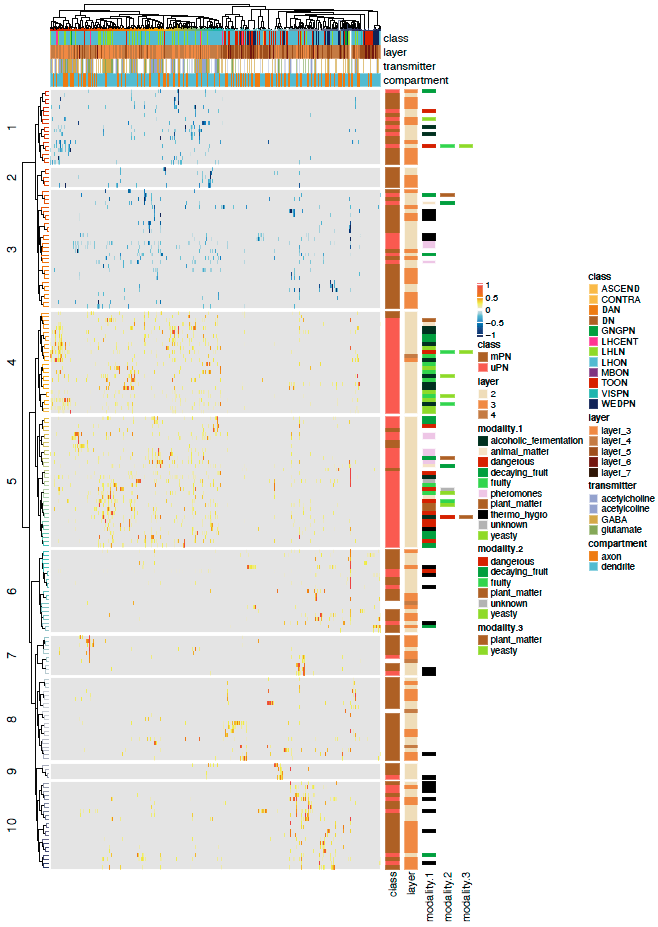
</center>
We can also plot the correlation heatmaps.
```{r heatmap.cor, eval = FALSE}
# Plot for TONs
pdf("ALPN_to_TOON_crosscorrelation_TOONs.pdf", height = 7, width = 10)
Heatmap(cor.mat,
name = "ALPNs' targets' cosine similarity",
split = NULL,
cluster_rows = cclus,
cluster_columns = cclus,
bottom_annotation = ha,
show_row_names = FALSE,
show_column_names = FALSE,
col = heatmap.cols,
row_title_gp = gpar(fontsize = 14, fontfamily ="Helvetica"),
column_title_gp = gpar(fontsize = 14, fontfamily ="Helvetica"),
row_names_gp = gpar(fontsize = 12, fontfamily ="Helvetica"),
column_names_gp = gpar(fontsize = 12, fontfamily ="Helvetica"))
dev.off()
# Plot for ALPNs
pdf("ALPN_to_TOON_crosscorrelation_ALPNs.pdf", height = 7, width = 10)
Heatmap(cor.mat.2,
name = "ALPNs' cosine similarity",
split = NULL,
cluster_rows = rclus,
cluster_columns = rclus,
bottom_annotation = ha2,
show_row_names = FALSE,
show_column_names = FALSE,
col = heatmap.cols,
row_title_gp = gpar(fontsize = 14, fontfamily ="Helvetica"),
column_title_gp = gpar(fontsize = 14, fontfamily ="Helvetica"),
row_names_gp = gpar(fontsize = 12, fontfamily ="Helvetica"),
column_names_gp = gpar(fontsize = 12, fontfamily ="Helvetica")
)
dev.off()
```
<center>
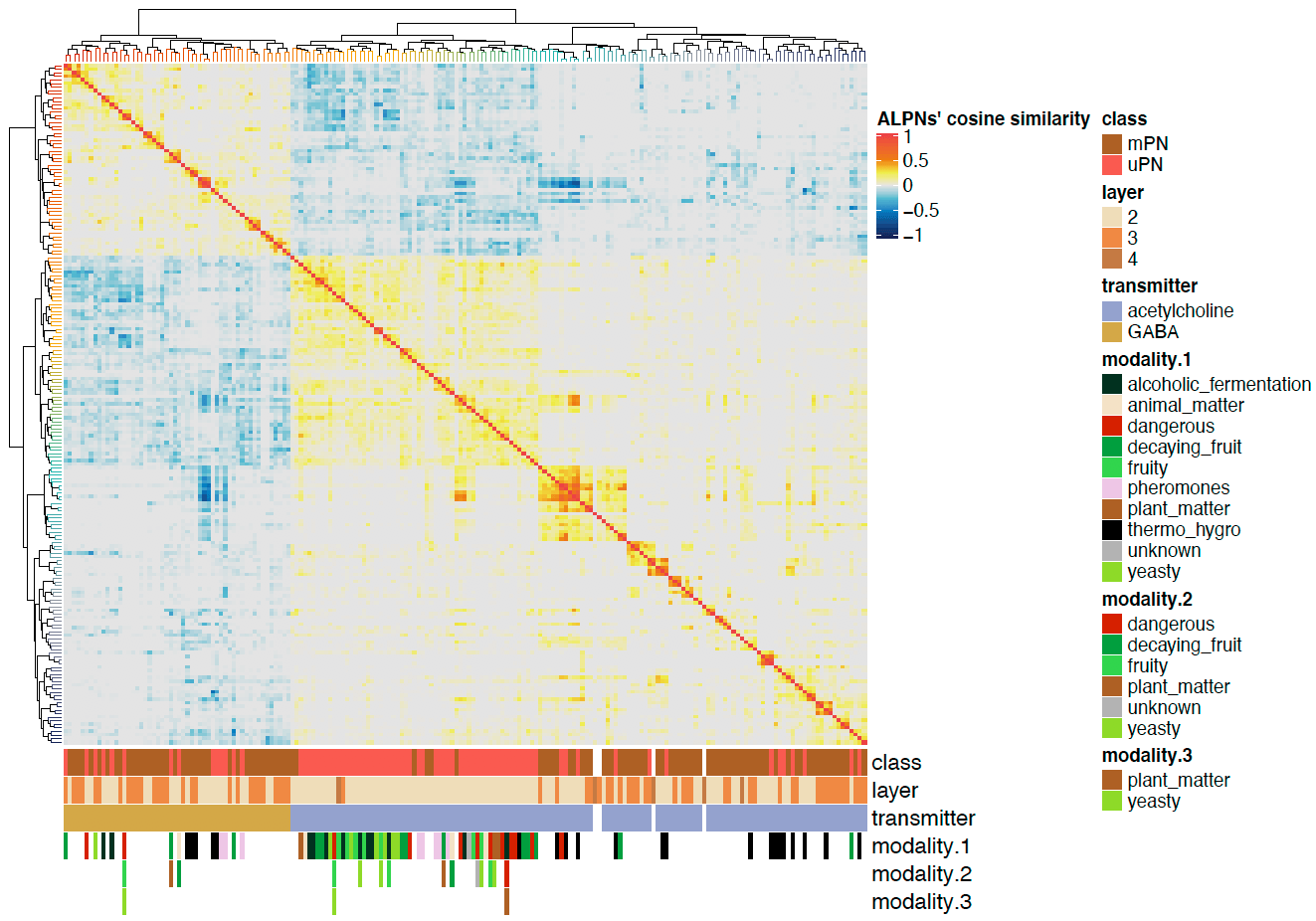
</center>
<center>
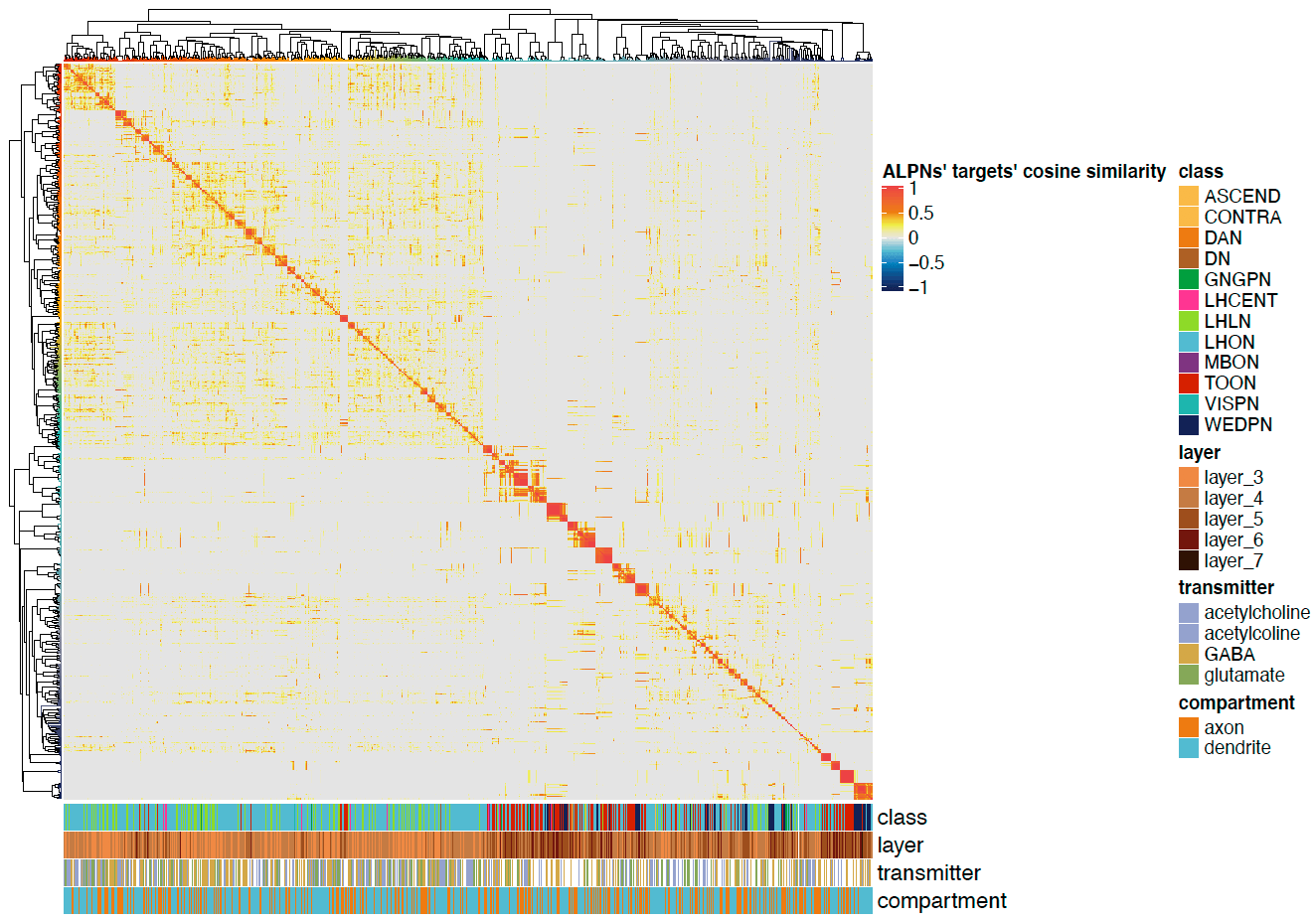
</center>