diff --git a/docs/source/ko/_toctree.yml b/docs/source/ko/_toctree.yml
index 80bd4c991a5bef..92289dafbf3d2c 100644
--- a/docs/source/ko/_toctree.yml
+++ b/docs/source/ko/_toctree.yml
@@ -69,9 +69,9 @@
- sections:
- local: tasks/image_captioning
title: 이미지 캡셔닝
- - local: in_translation
- title: (번역중) Document Question Answering
- title: (번역중) 멀티모달
+ - local: tasks/document_question_answering
+ title: 문서 질의 응답(Document Question Answering)
+ title: 멀티모달
isExpanded: false
title: 태스크 가이드
- sections:
diff --git a/docs/source/ko/tasks/document_question_answering.md b/docs/source/ko/tasks/document_question_answering.md
new file mode 100644
index 00000000000000..b9e98f3bf67235
--- /dev/null
+++ b/docs/source/ko/tasks/document_question_answering.md
@@ -0,0 +1,482 @@
+
+
+# 문서 질의 응답(Document Question Answering) [[document_question_answering]]
+
+[[open-in-colab]]
+
+문서 시각적 질의 응답(Document Visual Question Answering)이라고도 하는
+문서 질의 응답(Document Question Answering)은 문서 이미지에 대한 질문에 답변을 주는 태스크입니다.
+이 태스크를 지원하는 모델의 입력은 일반적으로 이미지와 질문의 조합이고, 출력은 자연어로 된 답변입니다. 이러한 모델은 텍스트, 단어의 위치(바운딩 박스), 이미지 등 다양한 모달리티를 활용합니다.
+
+이 가이드는 다음 내용을 설명합니다:
+
+- [DocVQA dataset](https://huggingface.co/datasets/nielsr/docvqa_1200_examples_donut)을 사용해 [LayoutLMv2](../model_doc/layoutlmv2) 미세 조정하기
+- 추론을 위해 미세 조정된 모델을 사용하기
+
+
+
+이 튜토리얼에서 설명하는 태스크는 다음과 같은 모델 아키텍처에서 지원됩니다:
+
+
+
+[LayoutLM](../model_doc/layoutlm), [LayoutLMv2](../model_doc/layoutlmv2), [LayoutLMv3](../model_doc/layoutlmv3)
+
+
+
+
+
+LayoutLMv2는 토큰의 마지막 은닉층 위에 질의 응답 헤드를 추가해 답변의 시작 토큰과 끝 토큰의 위치를 예측함으로써 문서 질의 응답 태스크를 해결합니다. 즉, 문맥이 주어졌을 때 질문에 답하는 정보를 추출하는 추출형 질의 응답(Extractive question answering)으로 문제를 처리합니다.
+문맥은 OCR 엔진의 출력에서 가져오며, 여기서는 Google의 Tesseract를 사용합니다.
+
+시작하기 전에 필요한 라이브러리가 모두 설치되어 있는지 확인하세요. LayoutLMv2는 detectron2, torchvision 및 테서랙트를 필요로 합니다.
+
+```bash
+pip install -q transformers datasets
+```
+
+```bash
+pip install 'git+https://github.com/facebookresearch/detectron2.git'
+pip install torchvision
+```
+
+```bash
+sudo apt install tesseract-ocr
+pip install -q pytesseract
+```
+
+필요한 라이브러리들을 모두 설치한 후 런타임을 다시 시작합니다.
+
+커뮤니티에 당신의 모델을 공유하는 것을 권장합니다. Hugging Face 계정에 로그인해서 모델을 🤗 Hub에 업로드하세요.
+프롬프트가 실행되면, 로그인을 위해 토큰을 입력하세요:
+
+```py
+>>> from huggingface_hub import notebook_login
+
+>>> notebook_login()
+```
+
+몇 가지 전역 변수를 정의해 보겠습니다.
+
+```py
+>>> model_checkpoint = "microsoft/layoutlmv2-base-uncased"
+>>> batch_size = 4
+```
+
+## 데이터 불러오기 [[load-the-data]]
+
+이 가이드에서는 🤗 Hub에서 찾을 수 있는 전처리된 DocVQA의 작은 샘플을 사용합니다.
+DocVQA의 전체 데이터 세트를 사용하고 싶다면, [DocVQA homepage](https://rrc.cvc.uab.es/?ch=17)에 가입 후 다운로드 할 수 있습니다. 전체 데이터 세트를 다운로드 했다면, 이 가이드를 계속 진행하기 위해 [🤗 dataset에 파일을 가져오는 방법](https://huggingface.co/docs/datasets/loading#local-and-remote-files)을 확인하세요.
+
+```py
+>>> from datasets import load_dataset
+
+>>> dataset = load_dataset("nielsr/docvqa_1200_examples")
+>>> dataset
+DatasetDict({
+ train: Dataset({
+ features: ['id', 'image', 'query', 'answers', 'words', 'bounding_boxes', 'answer'],
+ num_rows: 1000
+ })
+ test: Dataset({
+ features: ['id', 'image', 'query', 'answers', 'words', 'bounding_boxes', 'answer'],
+ num_rows: 200
+ })
+})
+```
+
+보시다시피, 데이터 세트는 이미 훈련 세트와 테스트 세트로 나누어져 있습니다. 무작위로 예제를 살펴보면서 특성을 확인해보세요.
+
+```py
+>>> dataset["train"].features
+```
+
+각 필드가 나타내는 내용은 다음과 같습니다:
+* `id`: 예제의 id
+* `image`: 문서 이미지를 포함하는 PIL.Image.Image 객체
+* `query`: 질문 문자열 - 여러 언어의 자연어로 된 질문
+* `answers`: 사람이 주석을 단 정답 리스트
+* `words` and `bounding_boxes`: OCR의 결과값들이며 이 가이드에서는 사용하지 않을 예정
+* `answer`: 다른 모델과 일치하는 답변이며 이 가이드에서는 사용하지 않을 예정
+
+영어로 된 질문만 남기고 다른 모델에 대한 예측을 포함하는 `answer` 특성을 삭제하겠습니다.
+그리고 주석 작성자가 제공한 데이터 세트에서 첫 번째 답변을 가져옵니다. 또는 무작위로 샘플을 추출할 수도 있습니다.
+
+```py
+>>> updated_dataset = dataset.map(lambda example: {"question": example["query"]["en"]}, remove_columns=["query"])
+>>> updated_dataset = updated_dataset.map(
+... lambda example: {"answer": example["answers"][0]}, remove_columns=["answer", "answers"]
+... )
+```
+
+이 가이드에서 사용하는 LayoutLMv2 체크포인트는 `max_position_embeddings = 512`로 훈련되었습니다(이 정보는 [체크포인트의 `config.json` 파일](https://huggingface.co/microsoft/layoutlmv2-base-uncased/blob/main/config.json#L18)에서 확인할 수 있습니다).
+바로 예제를 잘라낼 수도 있지만, 긴 문서의 끝에 답변이 있어 잘리는 상황을 피하기 위해 여기서는 임베딩이 512보다 길어질 가능성이 있는 몇 가지 예제를 제거하겠습니다.
+데이터 세트에 있는 대부분의 문서가 긴 경우 슬라이딩 윈도우 방법을 사용할 수 있습니다 - 자세한 내용을 확인하고 싶으면 이 [노트북](https://github.com/huggingface/notebooks/blob/main/examples/question_answering.ipynb)을 확인하세요.
+
+```py
+>>> updated_dataset = updated_dataset.filter(lambda x: len(x["words"]) + len(x["question"].split()) < 512)
+```
+
+이 시점에서 이 데이터 세트의 OCR 특성도 제거해 보겠습니다. OCR 특성은 다른 모델을 미세 조정하기 위한 것으로, 이 가이드에서 사용하는 모델의 입력 요구 사항과 일치하지 않기 때문에 이 특성을 사용하기 위해서는 일부 처리가 필요합니다.
+대신, 원본 데이터에 [`LayoutLMv2Processor`]를 사용하여 OCR 및 토큰화를 모두 수행할 수 있습니다.
+이렇게 하면 모델이 요구하는 입력을 얻을 수 있습니다.
+이미지를 수동으로 처리하려면, [`LayoutLMv2` model documentation](../model_doc/layoutlmv2)에서 모델이 요구하는 입력 포맷을 확인해보세요.
+
+```py
+>>> updated_dataset = updated_dataset.remove_columns("words")
+>>> updated_dataset = updated_dataset.remove_columns("bounding_boxes")
+```
+
+마지막으로, 데이터 탐색을 완료하기 위해 이미지 예시를 살펴봅시다.
+
+```py
+>>> updated_dataset["train"][11]["image"]
+```
+
+
+
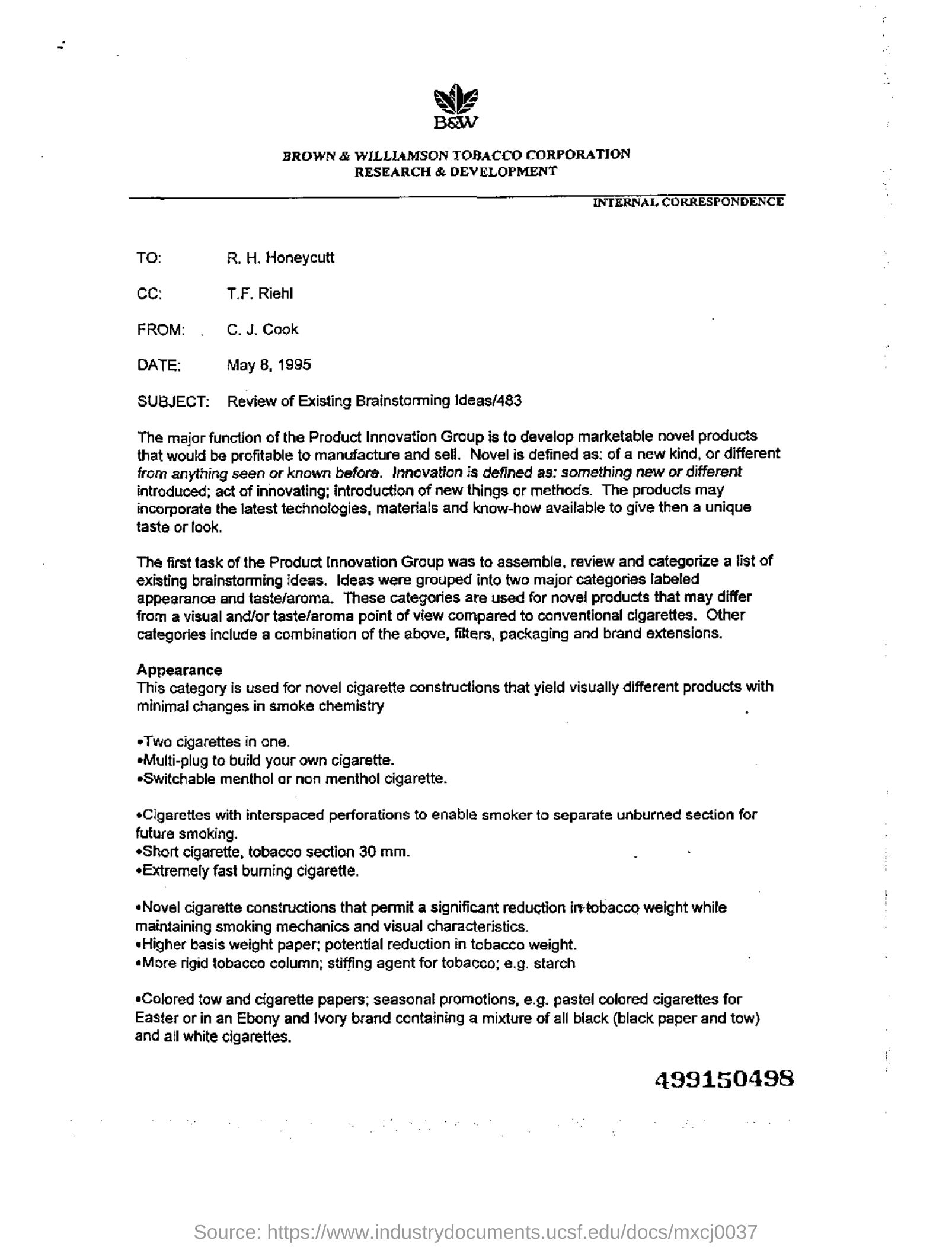
+
+
+## 데이터 전처리 [[preprocess-the-data]]
+
+
+문서 질의 응답 태스크는 멀티모달 태스크이며, 각 모달리티의 입력이 모델의 요구에 맞게 전처리 되었는지 확인해야 합니다.
+이미지 데이터를 처리할 수 있는 이미지 프로세서와 텍스트 데이터를 인코딩할 수 있는 토크나이저를 결합한 [`LayoutLMv2Processor`]를 가져오는 것부터 시작해 보겠습니다.
+
+```py
+>>> from transformers import AutoProcessor
+
+>>> processor = AutoProcessor.from_pretrained(model_checkpoint)
+```
+
+### 문서 이미지 전처리 [[preprocessing-document-images]]
+
+먼저, 프로세서의 `image_processor`를 사용해 모델에 대한 문서 이미지를 준비해 보겠습니다.
+기본값으로, 이미지 프로세서는 이미지 크기를 224x224로 조정하고 색상 채널의 순서가 올바른지 확인한 후 단어와 정규화된 바운딩 박스를 얻기 위해 테서랙트를 사용해 OCR를 적용합니다.
+이 튜토리얼에서 우리가 필요한 것과 기본값은 완전히 동일합니다. 이미지 배치에 기본 이미지 처리를 적용하고 OCR의 결과를 변환하는 함수를 작성합니다.
+
+```py
+>>> image_processor = processor.image_processor
+
+
+>>> def get_ocr_words_and_boxes(examples):
+... images = [image.convert("RGB") for image in examples["image"]]
+... encoded_inputs = image_processor(images)
+
+... examples["image"] = encoded_inputs.pixel_values
+... examples["words"] = encoded_inputs.words
+... examples["boxes"] = encoded_inputs.boxes
+
+... return examples
+```
+
+이 전처리를 데이터 세트 전체에 빠르게 적용하려면 [`~datasets.Dataset.map`]를 사용하세요.
+
+```py
+>>> dataset_with_ocr = updated_dataset.map(get_ocr_words_and_boxes, batched=True, batch_size=2)
+```
+
+### 텍스트 데이터 전처리 [[preprocessing-text-data]]
+
+이미지에 OCR을 적용했으면 데이터 세트의 텍스트 부분을 모델에 맞게 인코딩해야 합니다.
+이 인코딩에는 이전 단계에서 가져온 단어와 박스를 토큰 수준의 `input_ids`, `attention_mask`, `token_type_ids` 및 `bbox`로 변환하는 작업이 포함됩니다.
+텍스트를 전처리하려면 프로세서의 `tokenizer`가 필요합니다.
+
+```py
+>>> tokenizer = processor.tokenizer
+```
+
+위에서 언급한 전처리 외에도 모델을 위해 레이블을 추가해야 합니다. 🤗 Transformers의 `xxxForQuestionAnswering` 모델의 경우, 레이블은 `start_positions`와 `end_positions`로 구성되며 어떤 토큰이 답변의 시작과 끝에 있는지를 나타냅니다.
+
+레이블 추가를 위해서, 먼저 더 큰 리스트(단어 리스트)에서 하위 리스트(단어로 분할된 답변)을 찾을 수 있는 헬퍼 함수를 정의합니다.
+
+이 함수는 `words_list`와 `answer_list`, 이렇게 두 리스트를 입력으로 받습니다.
+그런 다음 `words_list`를 반복하여 `words_list`의 현재 단어(words_list[i])가 `answer_list`의 첫 번째 단어(answer_list[0])와 같은지,
+현재 단어에서 시작해 `answer_list`와 같은 길이만큼의 `words_list`의 하위 리스트가 `answer_list`와 일치하는지 확인합니다.
+이 조건이 참이라면 일치하는 항목을 발견했음을 의미하며, 함수는 일치 항목, 시작 인덱스(idx) 및 종료 인덱스(idx + len(answer_list) - 1)를 기록합니다. 일치하는 항목이 두 개 이상 발견되면 함수는 첫 번째 항목만 반환합니다. 일치하는 항목이 없다면 함수는 (`None`, 0, 0)을 반환합니다.
+
+```py
+>>> def subfinder(words_list, answer_list):
+... matches = []
+... start_indices = []
+... end_indices = []
+... for idx, i in enumerate(range(len(words_list))):
+... if words_list[i] == answer_list[0] and words_list[i : i + len(answer_list)] == answer_list:
+... matches.append(answer_list)
+... start_indices.append(idx)
+... end_indices.append(idx + len(answer_list) - 1)
+... if matches:
+... return matches[0], start_indices[0], end_indices[0]
+... else:
+... return None, 0, 0
+```
+
+이 함수가 어떻게 정답의 위치를 찾는지 설명하기 위해 다음 예제에서 함수를 사용해 보겠습니다:
+
+```py
+>>> example = dataset_with_ocr["train"][1]
+>>> words = [word.lower() for word in example["words"]]
+>>> match, word_idx_start, word_idx_end = subfinder(words, example["answer"].lower().split())
+>>> print("Question: ", example["question"])
+>>> print("Words:", words)
+>>> print("Answer: ", example["answer"])
+>>> print("start_index", word_idx_start)
+>>> print("end_index", word_idx_end)
+Question: Who is in cc in this letter?
+Words: ['wie', 'baw', 'brown', '&', 'williamson', 'tobacco', 'corporation', 'research', '&', 'development', 'internal', 'correspondence', 'to:', 'r.', 'h.', 'honeycutt', 'ce:', 't.f.', 'riehl', 'from:', '.', 'c.j.', 'cook', 'date:', 'may', '8,', '1995', 'subject:', 'review', 'of', 'existing', 'brainstorming', 'ideas/483', 'the', 'major', 'function', 'of', 'the', 'product', 'innovation', 'graup', 'is', 'to', 'develop', 'marketable', 'nove!', 'products', 'that', 'would', 'be', 'profitable', 'to', 'manufacture', 'and', 'sell.', 'novel', 'is', 'defined', 'as:', 'of', 'a', 'new', 'kind,', 'or', 'different', 'from', 'anything', 'seen', 'or', 'known', 'before.', 'innovation', 'is', 'defined', 'as:', 'something', 'new', 'or', 'different', 'introduced;', 'act', 'of', 'innovating;', 'introduction', 'of', 'new', 'things', 'or', 'methods.', 'the', 'products', 'may', 'incorporate', 'the', 'latest', 'technologies,', 'materials', 'and', 'know-how', 'available', 'to', 'give', 'then', 'a', 'unique', 'taste', 'or', 'look.', 'the', 'first', 'task', 'of', 'the', 'product', 'innovation', 'group', 'was', 'to', 'assemble,', 'review', 'and', 'categorize', 'a', 'list', 'of', 'existing', 'brainstorming', 'ideas.', 'ideas', 'were', 'grouped', 'into', 'two', 'major', 'categories', 'labeled', 'appearance', 'and', 'taste/aroma.', 'these', 'categories', 'are', 'used', 'for', 'novel', 'products', 'that', 'may', 'differ', 'from', 'a', 'visual', 'and/or', 'taste/aroma', 'point', 'of', 'view', 'compared', 'to', 'canventional', 'cigarettes.', 'other', 'categories', 'include', 'a', 'combination', 'of', 'the', 'above,', 'filters,', 'packaging', 'and', 'brand', 'extensions.', 'appearance', 'this', 'category', 'is', 'used', 'for', 'novel', 'cigarette', 'constructions', 'that', 'yield', 'visually', 'different', 'products', 'with', 'minimal', 'changes', 'in', 'smoke', 'chemistry', 'two', 'cigarettes', 'in', 'cne.', 'emulti-plug', 'te', 'build', 'yaur', 'awn', 'cigarette.', 'eswitchable', 'menthol', 'or', 'non', 'menthol', 'cigarette.', '*cigarettes', 'with', 'interspaced', 'perforations', 'to', 'enable', 'smoker', 'to', 'separate', 'unburned', 'section', 'for', 'future', 'smoking.', '«short', 'cigarette,', 'tobacco', 'section', '30', 'mm.', '«extremely', 'fast', 'buming', 'cigarette.', '«novel', 'cigarette', 'constructions', 'that', 'permit', 'a', 'significant', 'reduction', 'iretobacco', 'weight', 'while', 'maintaining', 'smoking', 'mechanics', 'and', 'visual', 'characteristics.', 'higher', 'basis', 'weight', 'paper:', 'potential', 'reduction', 'in', 'tobacco', 'weight.', '«more', 'rigid', 'tobacco', 'column;', 'stiffing', 'agent', 'for', 'tobacco;', 'e.g.', 'starch', '*colored', 'tow', 'and', 'cigarette', 'papers;', 'seasonal', 'promotions,', 'e.g.', 'pastel', 'colored', 'cigarettes', 'for', 'easter', 'or', 'in', 'an', 'ebony', 'and', 'ivory', 'brand', 'containing', 'a', 'mixture', 'of', 'all', 'black', '(black', 'paper', 'and', 'tow)', 'and', 'ail', 'white', 'cigarettes.', '499150498']
+Answer: T.F. Riehl
+start_index 17
+end_index 18
+```
+
+한편, 위 예제가 인코딩되면 다음과 같이 표시됩니다:
+
+```py
+>>> encoding = tokenizer(example["question"], example["words"], example["boxes"])
+>>> tokenizer.decode(encoding["input_ids"])
+[CLS] who is in cc in this letter? [SEP] wie baw brown & williamson tobacco corporation research & development ...
+```
+
+이제 인코딩된 입력에서 정답의 위치를 찾아야 합니다.
+* `token_type_ids`는 어떤 토큰이 질문에 속하는지, 그리고 어떤 토큰이 문서의 단어에 포함되는지를 알려줍니다.
+* `tokenizer.cls_token_id` 입력의 시작 부분에 있는 특수 토큰을 찾는 데 도움을 줍니다.
+* `word_ids`는 원본 `words`에서 찾은 답변을 전체 인코딩된 입력의 동일한 답과 일치시키고 인코딩된 입력에서 답변의 시작/끝 위치를 결정합니다.
+
+위 내용들을 염두에 두고 데이터 세트 예제의 배치를 인코딩하는 함수를 만들어 보겠습니다:
+
+```py
+>>> def encode_dataset(examples, max_length=512):
+... questions = examples["question"]
+... words = examples["words"]
+... boxes = examples["boxes"]
+... answers = examples["answer"]
+
+... # 예제 배치를 인코딩하고 start_positions와 end_positions를 초기화합니다
+... encoding = tokenizer(questions, words, boxes, max_length=max_length, padding="max_length", truncation=True)
+... start_positions = []
+... end_positions = []
+
+... # 배치의 예제를 반복합니다
+... for i in range(len(questions)):
+... cls_index = encoding["input_ids"][i].index(tokenizer.cls_token_id)
+
+... # 예제의 words에서 답변의 위치를 찾습니다
+... words_example = [word.lower() for word in words[i]]
+... answer = answers[i]
+... match, word_idx_start, word_idx_end = subfinder(words_example, answer.lower().split())
+
+... if match:
+... # 일치하는 항목을 발견하면, `token_type_ids`를 사용해 인코딩에서 단어가 시작하는 위치를 찾습니다
+... token_type_ids = encoding["token_type_ids"][i]
+... token_start_index = 0
+... while token_type_ids[token_start_index] != 1:
+... token_start_index += 1
+
+... token_end_index = len(encoding["input_ids"][i]) - 1
+... while token_type_ids[token_end_index] != 1:
+... token_end_index -= 1
+
+... word_ids = encoding.word_ids(i)[token_start_index : token_end_index + 1]
+... start_position = cls_index
+... end_position = cls_index
+
+... # words의 답변 위치와 일치할 때까지 word_ids를 반복하고 `token_start_index`를 늘립니다
+... # 일치하면 `token_start_index`를 인코딩에서 답변의 `start_position`으로 저장합니다
+... for id in word_ids:
+... if id == word_idx_start:
+... start_position = token_start_index
+... else:
+... token_start_index += 1
+
+... # 비슷하게, 끝에서 시작해 `word_ids`를 반복하며 답변의 `end_position`을 찾습니다
+... for id in word_ids[::-1]:
+... if id == word_idx_end:
+... end_position = token_end_index
+... else:
+... token_end_index -= 1
+
+... start_positions.append(start_position)
+... end_positions.append(end_position)
+
+... else:
+... start_positions.append(cls_index)
+... end_positions.append(cls_index)
+
+... encoding["image"] = examples["image"]
+... encoding["start_positions"] = start_positions
+... encoding["end_positions"] = end_positions
+
+... return encoding
+```
+
+이제 이 전처리 함수가 있으니 전체 데이터 세트를 인코딩할 수 있습니다:
+
+```py
+>>> encoded_train_dataset = dataset_with_ocr["train"].map(
+... encode_dataset, batched=True, batch_size=2, remove_columns=dataset_with_ocr["train"].column_names
+... )
+>>> encoded_test_dataset = dataset_with_ocr["test"].map(
+... encode_dataset, batched=True, batch_size=2, remove_columns=dataset_with_ocr["test"].column_names
+... )
+```
+
+인코딩된 데이터 세트의 특성이 어떻게 생겼는지 확인해 보겠습니다:
+
+```py
+>>> encoded_train_dataset.features
+{'image': Sequence(feature=Sequence(feature=Sequence(feature=Value(dtype='uint8', id=None), length=-1, id=None), length=-1, id=None), length=-1, id=None),
+ 'input_ids': Sequence(feature=Value(dtype='int32', id=None), length=-1, id=None),
+ 'token_type_ids': Sequence(feature=Value(dtype='int8', id=None), length=-1, id=None),
+ 'attention_mask': Sequence(feature=Value(dtype='int8', id=None), length=-1, id=None),
+ 'bbox': Sequence(feature=Sequence(feature=Value(dtype='int64', id=None), length=-1, id=None), length=-1, id=None),
+ 'start_positions': Value(dtype='int64', id=None),
+ 'end_positions': Value(dtype='int64', id=None)}
+```
+
+## 평가 [[evaluation]]
+
+문서 질의 응답을 평가하려면 상당한 양의 후처리가 필요합니다. 시간이 너무 많이 걸리지 않도록 이 가이드에서는 평가 단계를 생략합니다.
+[`Trainer`]가 훈련 과정에서 평가 손실(evaluation loss)을 계속 계산하기 때문에 모델의 성능을 대략적으로 알 수 있습니다.
+추출적(Extractive) 질의 응답은 보통 F1/exact match 방법을 사용해 평가됩니다.
+직접 구현해보고 싶으시다면, Hugging Face course의 [Question Answering chapter](https://huggingface.co/course/chapter7/7?fw=pt#postprocessing)을 참고하세요.
+
+## 훈련 [[train]]
+
+축하합니다! 이 가이드의 가장 어려운 부분을 성공적으로 처리했으니 이제 나만의 모델을 훈련할 준비가 되었습니다.
+훈련은 다음과 같은 단계로 이루어져 있습니다:
+* 전처리에서의 동일한 체크포인트를 사용하기 위해 [`AutoModelForDocumentQuestionAnswering`]으로 모델을 가져옵니다.
+* [`TrainingArguments`]로 훈련 하이퍼파라미터를 정합니다.
+* 예제를 배치 처리하는 함수를 정의합니다. 여기서는 [`DefaultDataCollator`]가 적당합니다.
+* 모델, 데이터 세트, 데이터 콜레이터(Data collator)와 함께 [`Trainer`]에 훈련 인수들을 전달합니다.
+* [`~Trainer.train`]을 호출해서 모델을 미세 조정합니다.
+
+```py
+>>> from transformers import AutoModelForDocumentQuestionAnswering
+
+>>> model = AutoModelForDocumentQuestionAnswering.from_pretrained(model_checkpoint)
+```
+
+[`TrainingArguments`]에서 `output_dir`을 사용하여 모델을 저장할 위치를 지정하고, 적절한 하이퍼파라미터를 설정합니다.
+모델을 커뮤니티와 공유하려면 `push_to_hub`를 `True`로 설정하세요 (모델을 업로드하려면 Hugging Face에 로그인해야 합니다).
+이 경우 `output_dir`은 모델의 체크포인트를 푸시할 레포지토리의 이름이 됩니다.
+
+```py
+>>> from transformers import TrainingArguments
+
+>>> # 본인의 레포지토리 ID로 바꾸세요
+>>> repo_id = "MariaK/layoutlmv2-base-uncased_finetuned_docvqa"
+
+>>> training_args = TrainingArguments(
+... output_dir=repo_id,
+... per_device_train_batch_size=4,
+... num_train_epochs=20,
+... save_steps=200,
+... logging_steps=50,
+... evaluation_strategy="steps",
+... learning_rate=5e-5,
+... save_total_limit=2,
+... remove_unused_columns=False,
+... push_to_hub=True,
+... )
+```
+
+간단한 데이터 콜레이터를 정의하여 예제를 함께 배치합니다.
+
+```py
+>>> from transformers import DefaultDataCollator
+
+>>> data_collator = DefaultDataCollator()
+```
+
+마지막으로, 모든 것을 한 곳에 모아 [`~Trainer.train`]을 호출합니다:
+
+```py
+>>> from transformers import Trainer
+
+>>> trainer = Trainer(
+... model=model,
+... args=training_args,
+... data_collator=data_collator,
+... train_dataset=encoded_train_dataset,
+... eval_dataset=encoded_test_dataset,
+... tokenizer=processor,
+... )
+
+>>> trainer.train()
+```
+
+최종 모델을 🤗 Hub에 추가하려면, 모델 카드를 생성하고 `push_to_hub`를 호출합니다:
+
+```py
+>>> trainer.create_model_card()
+>>> trainer.push_to_hub()
+```
+
+## 추론 [[inference]]
+
+이제 LayoutLMv2 모델을 미세 조정하고 🤗 Hub에 업로드했으니 추론에도 사용할 수 있습니다.
+추론을 위해 미세 조정된 모델을 사용해 보는 가장 간단한 방법은 [`Pipeline`]을 사용하는 것 입니다.
+
+예를 들어 보겠습니다:
+```py
+>>> example = dataset["test"][2]
+>>> question = example["query"]["en"]
+>>> image = example["image"]
+>>> print(question)
+>>> print(example["answers"])
+'Who is ‘presiding’ TRRF GENERAL SESSION (PART 1)?'
+['TRRF Vice President', 'lee a. waller']
+```
+
+그 다음, 모델로 문서 질의 응답을 하기 위해 파이프라인을 인스턴스화하고 이미지 + 질문 조합을 전달합니다.
+
+```py
+>>> from transformers import pipeline
+
+>>> qa_pipeline = pipeline("document-question-answering", model="MariaK/layoutlmv2-base-uncased_finetuned_docvqa")
+>>> qa_pipeline(image, question)
+[{'score': 0.9949808120727539,
+ 'answer': 'Lee A. Waller',
+ 'start': 55,
+ 'end': 57}]
+```
+
+원한다면 파이프라인의 결과를 수동으로 복제할 수도 있습니다:
+1. 이미지와 질문을 가져와 모델의 프로세서를 사용해 모델에 맞게 준비합니다.
+2. 모델을 통해 결과 또는 전처리를 전달합니다.
+3. 모델은 어떤 토큰이 답변의 시작에 있는지, 어떤 토큰이 답변이 끝에 있는지를 나타내는 `start_logits`와 `end_logits`를 반환합니다. 둘 다 (batch_size, sequence_length) 형태를 갖습니다.
+4. `start_logits`와 `end_logits`의 마지막 차원을 최대로 만드는 값을 찾아 예상 `start_idx`와 `end_idx`를 얻습니다.
+5. 토크나이저로 답변을 디코딩합니다.
+
+```py
+>>> import torch
+>>> from transformers import AutoProcessor
+>>> from transformers import AutoModelForDocumentQuestionAnswering
+
+>>> processor = AutoProcessor.from_pretrained("MariaK/layoutlmv2-base-uncased_finetuned_docvqa")
+>>> model = AutoModelForDocumentQuestionAnswering.from_pretrained("MariaK/layoutlmv2-base-uncased_finetuned_docvqa")
+
+>>> with torch.no_grad():
+... encoding = processor(image.convert("RGB"), question, return_tensors="pt")
+... outputs = model(**encoding)
+... start_logits = outputs.start_logits
+... end_logits = outputs.end_logits
+... predicted_start_idx = start_logits.argmax(-1).item()
+... predicted_end_idx = end_logits.argmax(-1).item()
+
+>>> processor.tokenizer.decode(encoding.input_ids.squeeze()[predicted_start_idx : predicted_end_idx + 1])
+'lee a. waller'
+```
\ No newline at end of file