-
-
Notifications
You must be signed in to change notification settings - Fork 707
New issue
Have a question about this project? Sign up for a free GitHub account to open an issue and contact its maintainers and the community.
By clicking “Sign up for GitHub”, you agree to our terms of service and privacy statement. We’ll occasionally send you account related emails.
Already on GitHub? Sign in to your account
Confirmed cases for 长春市 and 吉林市 were flipped #110
Comments
Surely John Hopkins data about China Covid is definably inaccurate |
I guess John Hopkins data totally different with real China covid data is a big reason for wrong assessment of China's novel coronavirus pneumonia. |
JHU works well at provincial level (ADM 1) but there is no Chinese prefecture/city level data (ADM 2) that I'm aware of. |
I compare JHU with official post already, and they are totally mismatched. about 1200 in recent days, but as I know, the data is totally different. I still couldn't know how to use this data repos, but may you can try to do a comparison |
If want to get Chinese data must know Chinese and spend time to arrange it. |
@beansrowning Finally solved the case |
Hi @beansrowning, Thanks a lot for your report! I have checked your NHC post on 3/15, the database and the dumped CSV/JSON file, and you are right. The values of Changchun and Jilin City on the timestamp 1647311180134 (Tue Mar 15, 2022, 02:26:20 GMT+0000 or Tue Mar 15, 2022, 10:26:20 GMT+0800) are flipped. These data are directly scraped from DXY, which, as far as I know, manually collects and updates on their site and might have some wrong values, especially in the early days. The purpose of the crawler and this data warehouse is to maintain a time-series database, so I prefer not to modify the data directly in the database. But I will address this problem in the Noise Data part on the README file for others' awareness. Thanks again for your contribution. Also, thank you for letting me know the dataset really helped in your research. Being helpful in scientific research is my main purpose in building and maintaining this dataset. Cheers! |
This doesn't seem like something you can fix on your end, but I wanted to bring to your/others awareness that I noticed the values of confirmed cases reported in Changchun and Jilin City were flipped on 3/15, which has thrown off cumulative cases since.
Per NHC on 3/15 (http://www.nhc.gov.cn/xcs/yqtb/202203/8d8d2035b3884fcfb734e0ab07bede79.shtml):
Changchun: +460
Jilin City: +2601
Per DXY scrape:
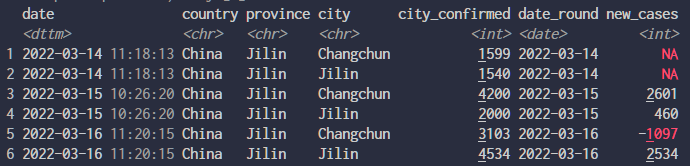
Also, just a thank you for your continued support of this service. I started using these data early in 2020, and they've continued to be valuable some two years later. I've used them to provide situational awareness to US Government and colleagues internationally. I've found no better publicly available source of ADM2-level data to date.
Cheers!
The text was updated successfully, but these errors were encountered: